Finding Multi-granularity Community Structures in Social Networks Based on Significance of Community Partition.
ICDM Workshops(2018)
摘要
Community structure detection is an important and valuable task in social network studies as it is the base for many social network applications such as link prediction, recommendation, etc. Most social networks have an inherent multi-granular structure, which leads to different community structures at different granularities. However, few studies pay attention to such multi-granular characteristics of social networks. In this paper, a method called MGCD (Multi-Granularity Community Detection) is proposed for finding multi-granularity community structures of social networks. At first, a network embedding method is used to obtain the low-dimensional vector representation for each node. Then, an effective embedding-based strategy for weakening the detected community structures is proposed. Finally, a joint learning framework, which combines network embedding and community structure weakening is developed for identifying the multi-granularity community structures of social networks. Experimental results on real-world networks show that MGCD outperforms the state-of-the-art benchmark methods on finding multi-granularity community structure tasks.
更多查看译文
关键词
Social networking (online),Image edge detection,Linear programming,Computational intelligence,Telecommunications,Gaussian mixture model
AI 理解论文
溯源树
样例
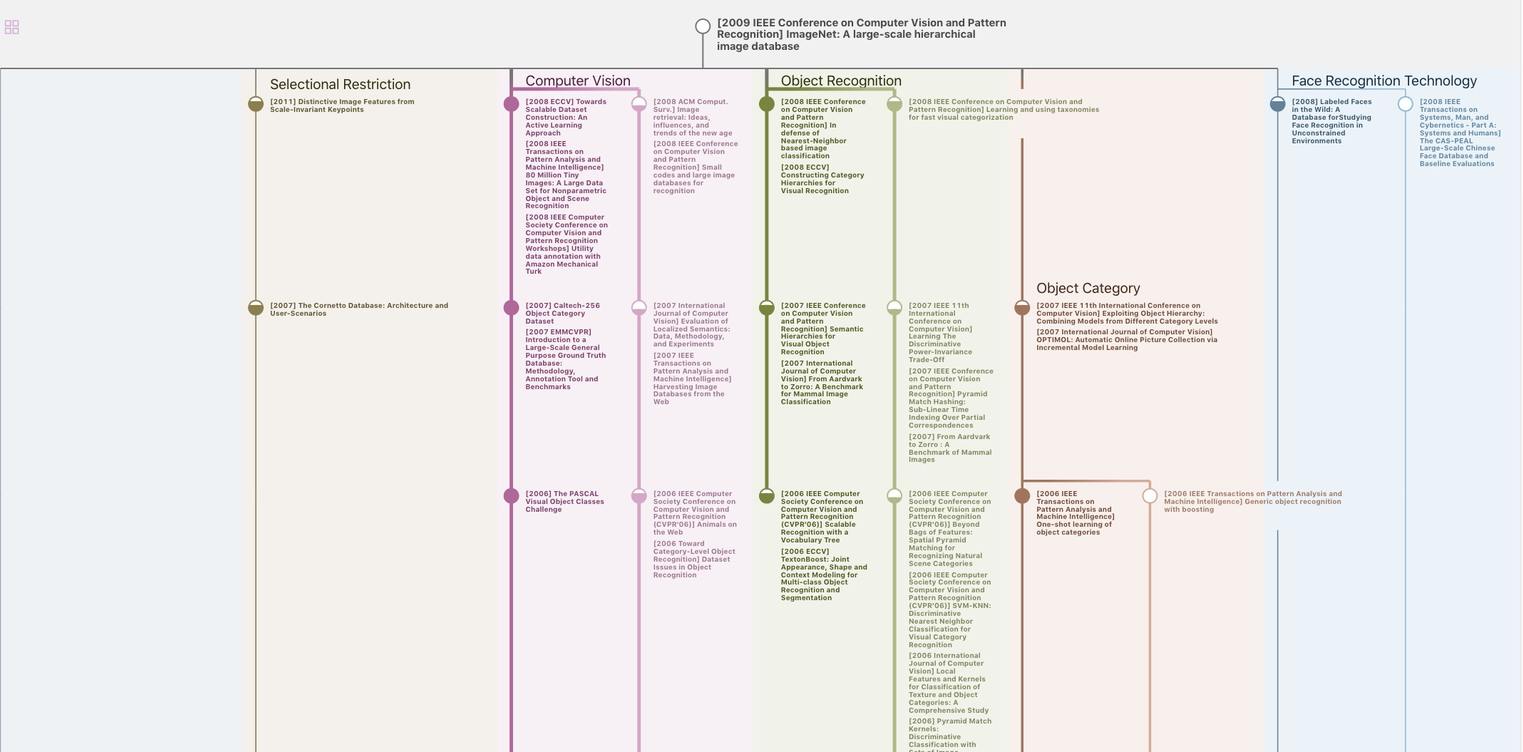
生成溯源树,研究论文发展脉络
Chat Paper
正在生成论文摘要