Building robust classifiers through generation of confident out of distribution examples.
arXiv: Machine Learning(2018)
摘要
Deep learning models are known to be overconfident in their predictions on out of distribution inputs. There have been several pieces of work to address this issue, including a number of approaches for building Bayesian neural networks, as well as closely related work on detection of out of distribution samples. Recently, there has been work on building classifiers that are robust to out of distribution samples by adding a regularization term that maximizes the entropy of the classifier output on out of distribution data. To approximate out of distribution samples (which are not known apriori), a GAN was used for generation of samples at the edges of the training distribution. In this paper, we introduce an alternative GAN based approach for building a robust classifier, where the idea is to use the GAN to explicitly generate out of distribution samples that the classifier is confident on (low entropy), and have the classifier maximize the entropy for these samples. We showcase the effectiveness of our approach relative to state-of-the-art on hand-written characters as well as on a variety of natural image datasets.
更多查看译文
AI 理解论文
溯源树
样例
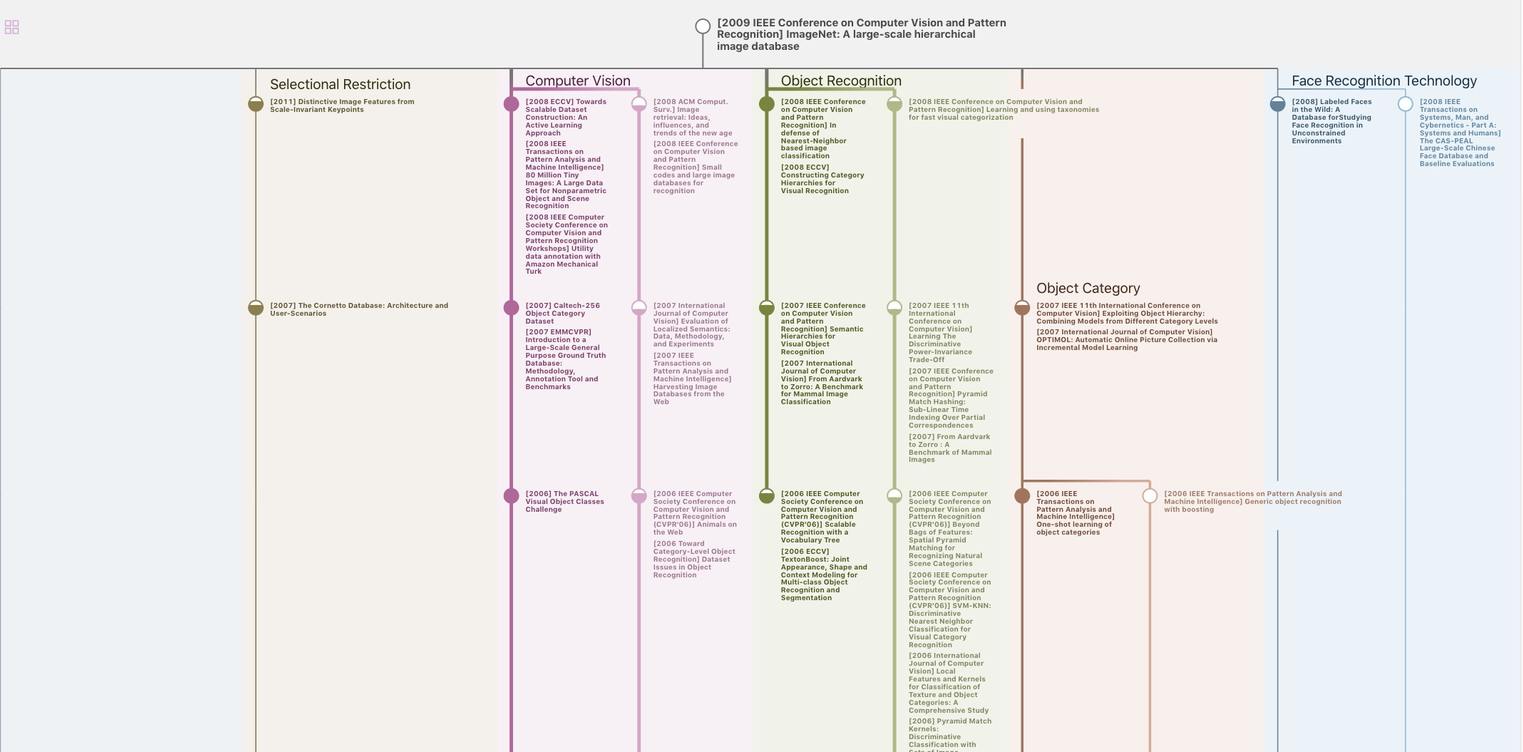
生成溯源树,研究论文发展脉络
Chat Paper
正在生成论文摘要