Privacy-preserving Data Mining in Industry.
WSDM(2019)
摘要
Preserving privacy of users is a key requirement of web-scale data mining applications and systems such as web search, recommender systems, crowdsourced platforms, and analytics applications, and has witnessed a renewed focus in light of recent data breaches and new regulations such as GDPR. In this tutorial, we will first present an overview of privacy breaches over the last two decades and the lessons learned, key regulations and laws, and evolution of privacy techniques leading to differential privacy definition / techniques. Then, we will focus on the application of privacy-preserving data mining techniques in practice, by presenting case studies such as Apple's differential privacy deployment for iOS / macOS, Google's RAPPOR, LinkedIn Salary, and Microsoft's differential privacy deployment for collecting Windows telemetry. We will conclude with open problems and challenges for the data mining / machine learning community, based on our experiences in industry.
更多查看译文
关键词
apple's differential privacy deployment for ios and macos, differential privacy, google's rappor, linkedin salary, local differential privacy, microsoft's differential privacy deployment for collecting windows telemetry, privacy breaches, privacy case studies in industry, privacy-preserving data mining, privacy-preserving web analytics and reporting
AI 理解论文
溯源树
样例
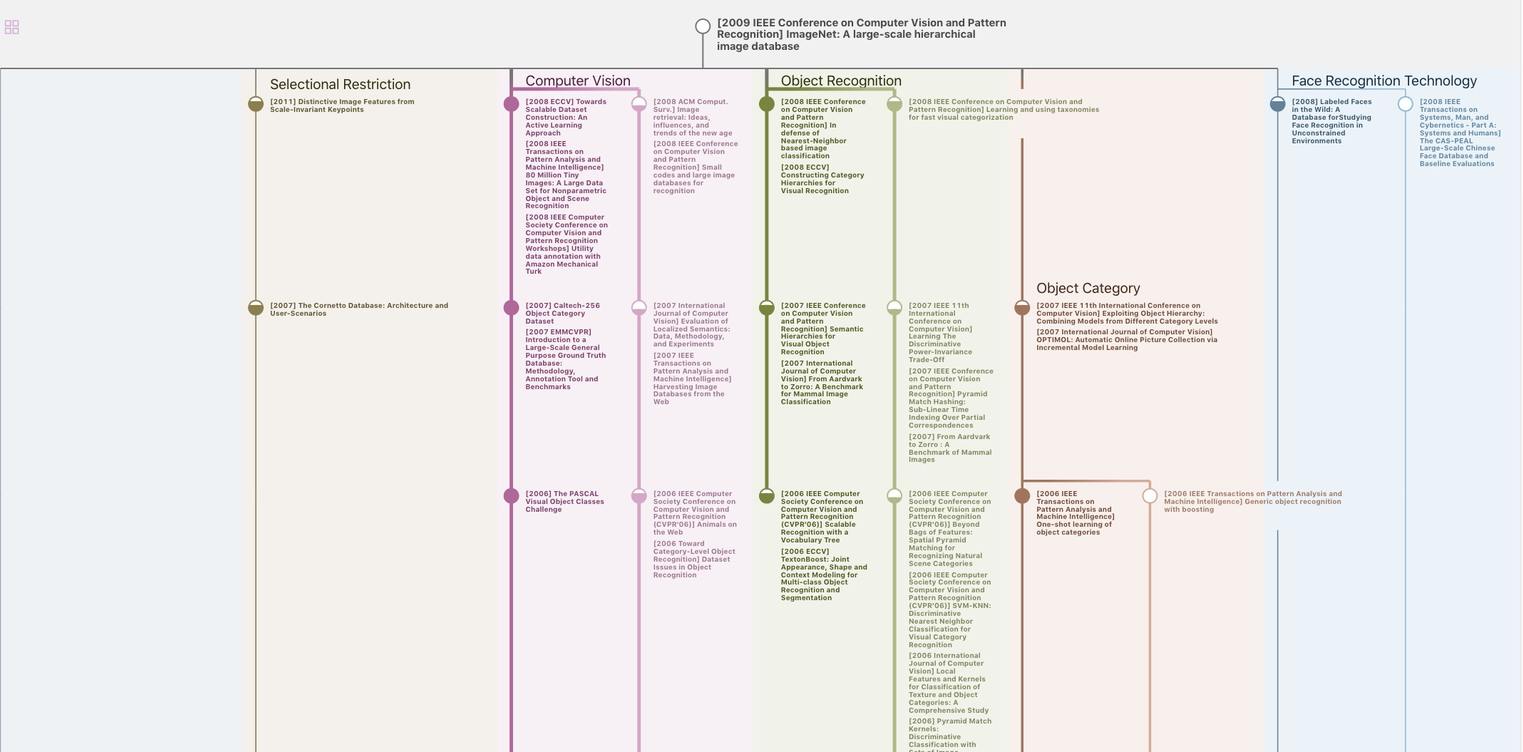
生成溯源树,研究论文发展脉络
Chat Paper
正在生成论文摘要