Heuristic Approximation of Early-Stage CNN Data Representation for Vision Intelligence Systems
2018 IEEE 36th International Conference on Computer Design (ICCD)(2018)
摘要
Massive memory requirements and disruptive I/O traffic in Convolutional Neural Networks (CNNs) restrict hardware/software optimization for training and inference tasks using larger networks despite their success in improving the intelligence of systems into which they are integrated. Interestingly, it has been observed that the early CNN layers in vision tasks consistently produce edge-like feature representations that can be mimicked by traditional vision algorithms. In this work, we demonstrate how to exploit this to create common edge-like features for sharing among multiple CNNs, and how to heuristically approximate them in a reduced dimensionality. In our proposed approximation, the feature space of three representative CNNs decreases by 1.6×-5.1×, and the size of training dataset is halved. As a result, we enhance both inference throughput and training speed by 2×, while providing accuracies that are still close to the original versions. We anticipate that this approach will lead to new opportunities in distributed intelligence systems, and the technique for redesigning CNN models based on dimensional reduction of feature space is orthogonal to and compatible with many other existing hardware/software optimizations.
更多查看译文
关键词
Approximate computing,Accelerator architectures,Neural networks
AI 理解论文
溯源树
样例
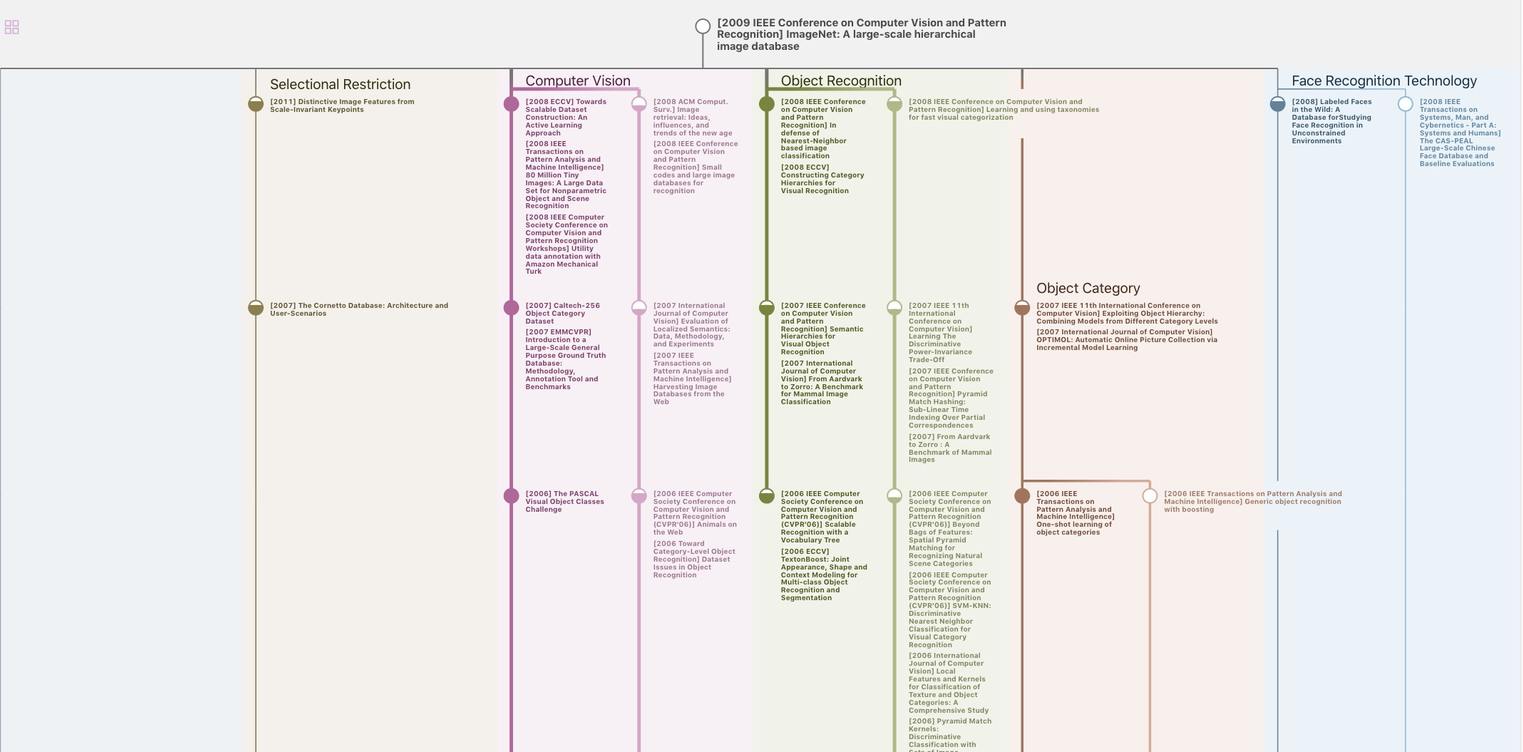
生成溯源树,研究论文发展脉络
Chat Paper
正在生成论文摘要