Color Quantization in Transfer Learning and Noisy Scenarios: An Empirical Analysis Using Convolutional Networks
2018 31st SIBGRAPI Conference on Graphics, Patterns and Images (SIBGRAPI)(2018)
摘要
Transfer learning is seen as one of the most promising areas of machine learning. Lately, features from pre-trained models have been used to achieve state-of-the-art results in several machine vision problems. Those models are usually employed when the problem of interest does not have enough supervised examples to support the network training from scratch. Most applications use networks pre-trained on noise-free RGB image datasets, what is observed even when the target domain counts on grayscale images or when data is degraded by noise. In this paper, we evaluate the use of Convolutional Neural Networks (CNNs) on such transfer learning scenarios and the impact of using RGB trained networks on grayscale image tasks. Our results confirm that the use of networks trained using colored images on grayscale tasks hinders the overall performance when compared to a similar network trained on a quantized version of the original dataset. Results also show that higher quantization levels (resulting in less colors) increase the robustness of CNN features in the presence of noise.
更多查看译文
关键词
transfer learning,deep learning,computer vision
AI 理解论文
溯源树
样例
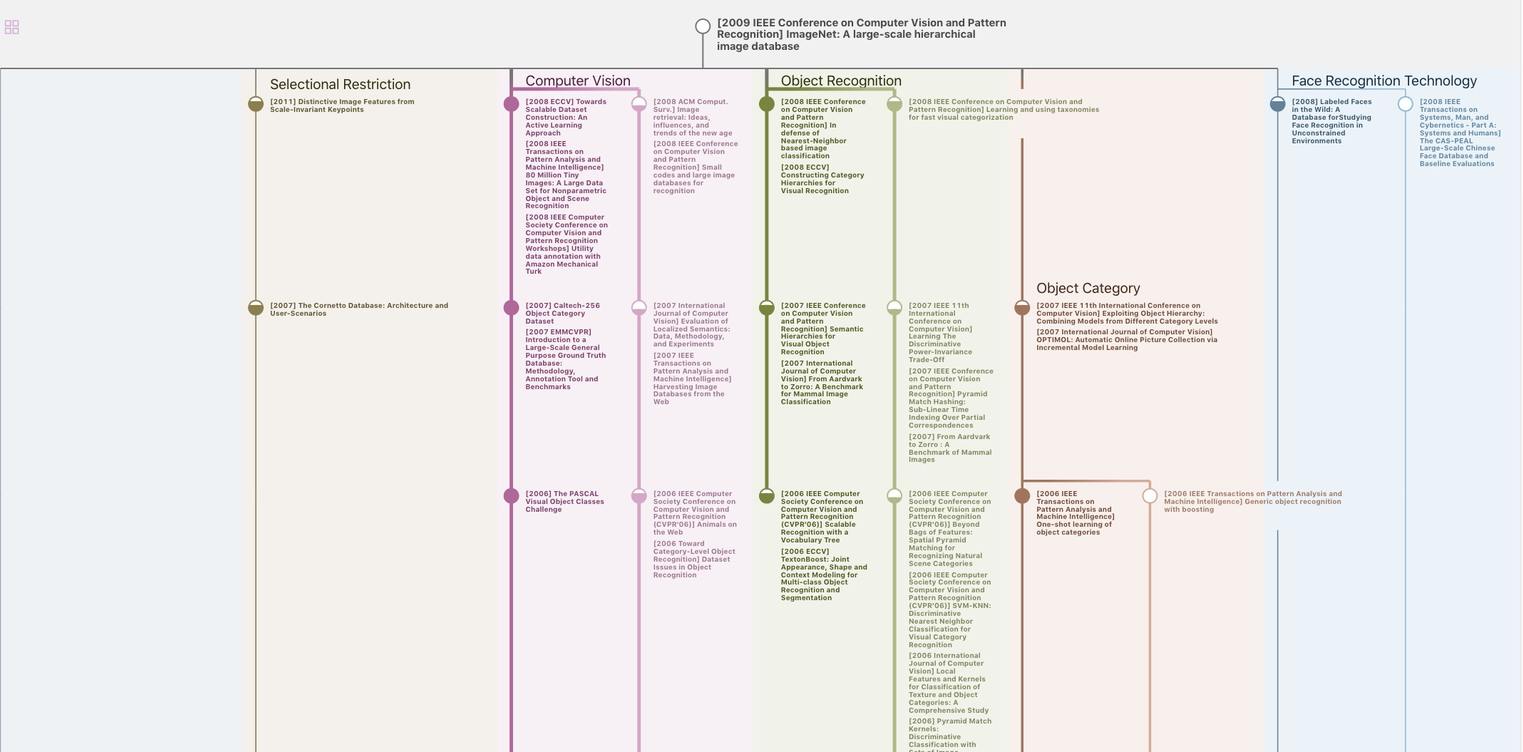
生成溯源树,研究论文发展脉络
Chat Paper
正在生成论文摘要