A Learning-Guided Hierarchical Approach for Biomedical Image Segmentation
2018 31st IEEE International System-on-Chip Conference (SOCC)(2018)
摘要
In recent years, deep convolutional networks have been widely used for a variety of visual recognition tasks, including biomedical applications. In most studies related to biomedical domain (e.g., cell tracking), the first step is to perform symmetric segmentation on target images. Such image datasets have usually the following challenges: (1) they lack human labeled training data, (2) the locations of the objects in images are as equally important as classifying them, and (3) the result accuracy is more critical than that in traditional image segmentation. To address these problems, recent studies employ large deep neural networks to perform segmentation on biomedical images. However, such neural network approaches are very compute intensive due to the high resolution and large quantity of electron microscopy data. Additionally, some of the efforts that make use of neural network models involve redundancy as target biomedical images usually contain smaller regions of interest. Motivated by these observations, in this paper, we propose and experimentally evaluate a more efficient framework, especially suited for image segmentation on embedded systems. This approach involves first “tiling” the target image, followed by processing the tiles that only contain an object of interest in a hierarchical fashion. Our detailed experimental evaluations using four different datasets indicate that our tiling-based approach can save about 61% of execution time on average, while achieving, at the same time, a slightly higher accuracy compared to the baseline (state of the art) approach.
更多查看译文
关键词
biomedical image segmentation,attention model,cell tracking
AI 理解论文
溯源树
样例
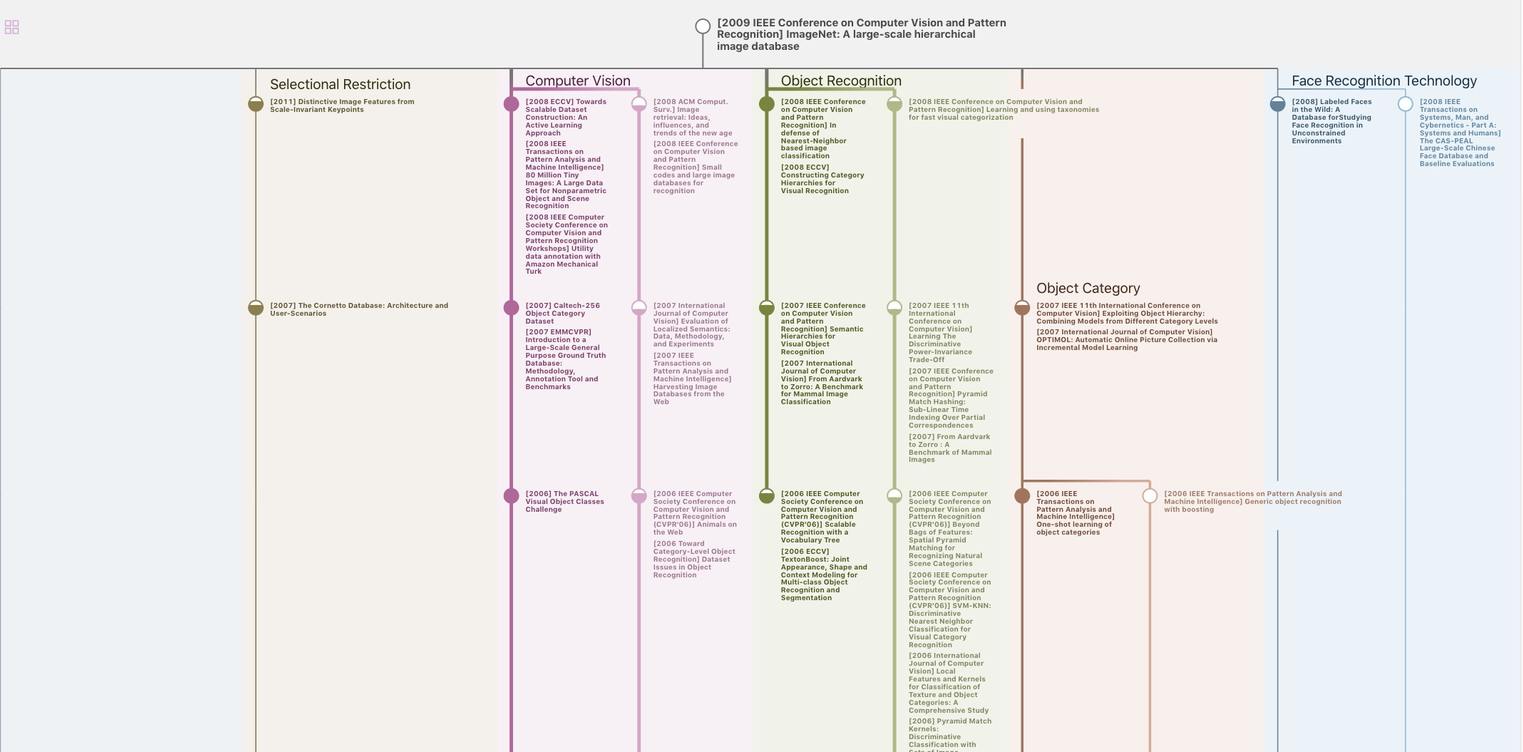
生成溯源树,研究论文发展脉络
Chat Paper
正在生成论文摘要