Electrodermal Activity Based Pre-surgery Stress Detection Using a Wrist Wearable.
IEEE journal of biomedical and health informatics(2020)
摘要
Surgery is a particularly potent stressor and the detrimental effects of stress on people undergoing any surgery is indisputable. When left unchecked, the pre-surgery stress adversely impacts people's physical and psychological well-being, and may even evolve into severe pathological states. Therefore, it is essential to identify levels of preoperative stress in surgical patients. This paper focuses on developing an automatic pre-surgery stress detection scheme based on electrodermal activity (EDA). The measurement set up involves a wrist wearable that monitors EDA of a subject continuously in the most non-invasive and unobtrusive manner. Data were collected from 41 subjects [17 females and 24 males, age: 54.8
$\pm$
16.8 years (mean
$\pm$
SD)], who subsequently underwent different surgical procedures at the Sri Ramakrishna Hospital, Coimbatore, India. A supervised machine learning algorithm that detects motion artifacts in the recorded EDA data was developed. It yielded an accuracy of 97.83% on a new user dataset. The clean EDA data were further analyzed to determine low, moderate, and high levels of stress. A novel localized supervised learning scheme based on the adaptive partitioning of the dataset was adopted for stress detection. Consequently, the interindividual variability in the EDA due to person-specific factors such as the sweat gland density and skin thickness, which may lead to erroneous classification, could be eliminated. The scheme yielded a classification accuracy of 85.06% on a new user dataset and proved to be more effective than the general supervised classification model.
更多查看译文
关键词
Stress,Biomedical monitoring,Surgery,Wrist,Stress measurement,Informatics,Monitoring
AI 理解论文
溯源树
样例
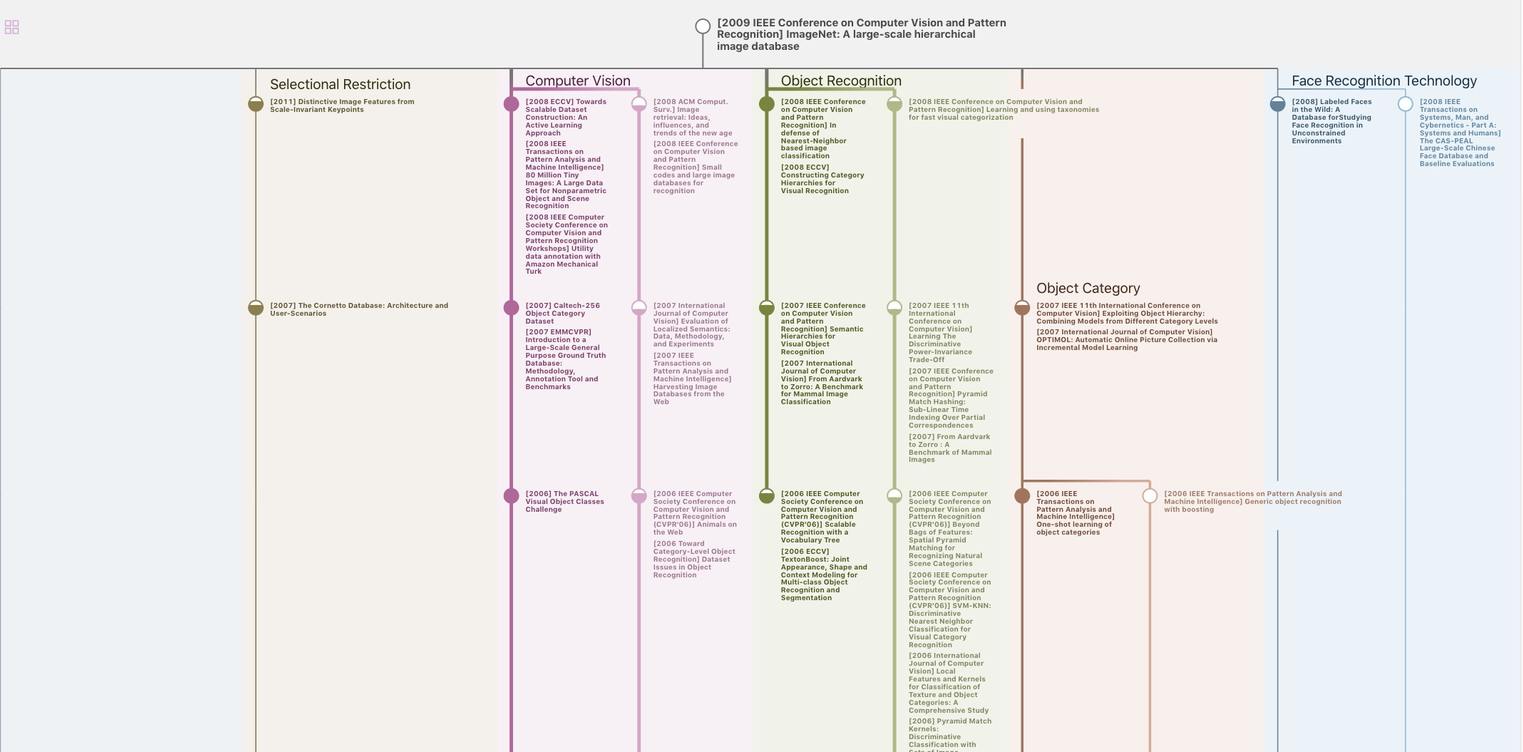
生成溯源树,研究论文发展脉络
Chat Paper
正在生成论文摘要