State Transition Pattern with Periodic Wildcard Gaps
2018 IEEE International Conference on Big Knowledge (ICBK)(2018)
摘要
Sequence pattern discovery is a key issue in multivariate time series analysis. Popular methods consist of three stages: feature extraction, feature clustering, and block sequence discovery. Both cross and temporal associations are obtained during the third stage. In this paper, we propose a new type of pattern called a state transition pattern with periodic wildcard gaps (STAP) to enrich cross associations. We design an approach that consists of three stages, that is, feature extraction, frequent state discovery, and pattern synthesis, to obtain frequent STAPs. Compared with previous approaches, STAP emonstrates stronger cross associations by considering different variables multaneously. We propose two pre-pruning and the Apriori-pruning technique to speed up pattern discovery. We also propose a type of graph to visualize STAPs. Experimental results on three real-world datasets and one artificial dataset demonstrate that STAPs capture richer cross and temporal associations.
更多查看译文
关键词
cross association,multivariate time series,sequential pattern
AI 理解论文
溯源树
样例
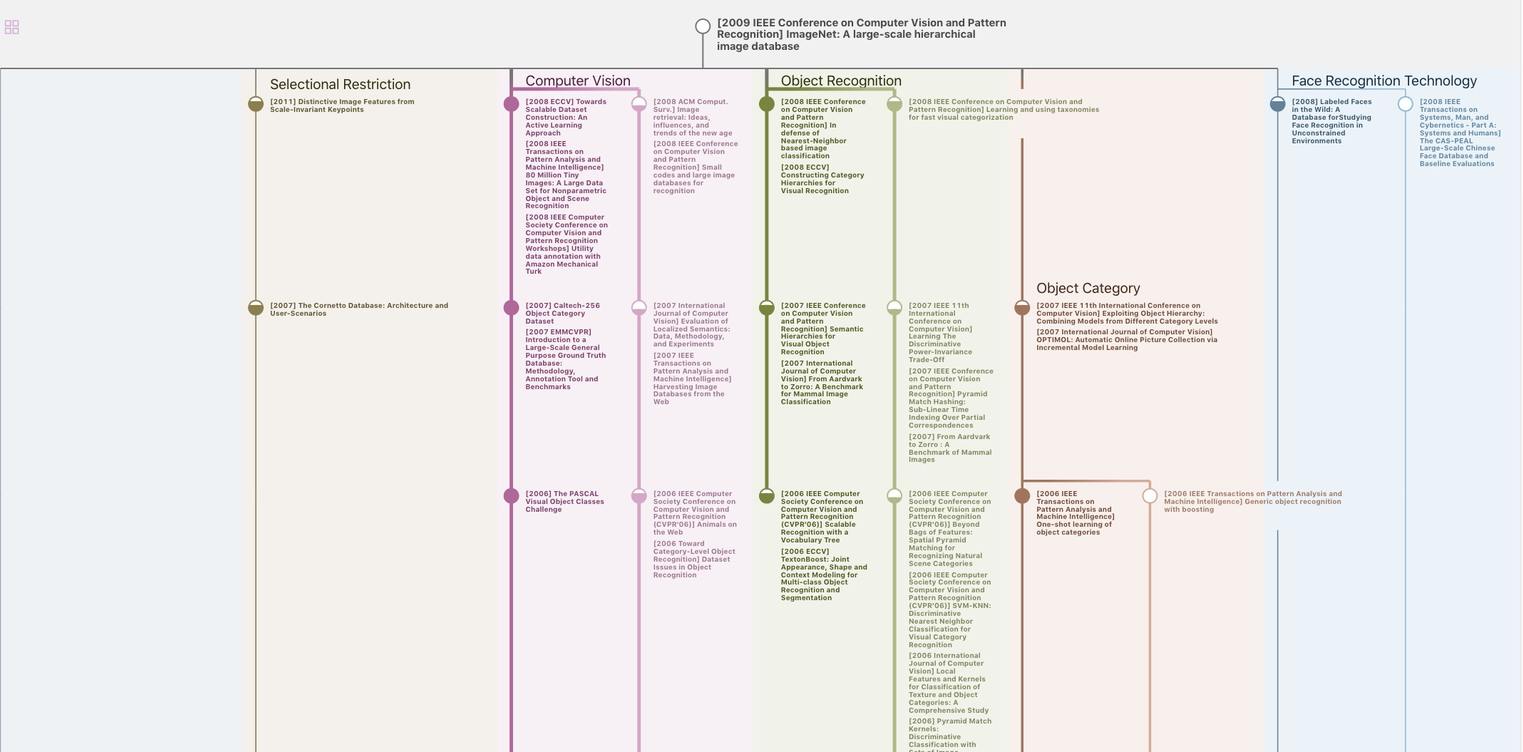
生成溯源树,研究论文发展脉络
Chat Paper
正在生成论文摘要