A novel density peaks clustering with sensitivity of local density and density-adaptive metric
Knowledge and Information Systems(2018)
摘要
The density peaks (DP) clustering approach is a novel density-based clustering algorithm. On the basis of the prior assumption of consistency for semi-supervised learning problems, we further make the assumptions of consistency for density-based clustering. The first one is the assumption of the local consistency, which means nearby points are likely to have the similar local density; the second one is the assumption of the global consistency, which means points on the same high-density area (or the same structure, i.e., the same cluster) are likely to have the same label. According to the first assumption, we provide a new option based on the sensitivity of the local density for the local density. In addition, we redefine δ and redesign the assignation strategy based on a new density-adaptive metric according to the second assumption. We compare the performance of our algorithm with traditional clustering schemes, including DP, K -means, fuzzy C-means, Gaussian mixture model, and self-organizing maps. Experiments on different benchmark data sets demonstrate the effectiveness of the proposed algorithm.
更多查看译文
关键词
Clustering analysis,Density peaks clustering,Sensitivity of local density,Density-adaptive distance
AI 理解论文
溯源树
样例
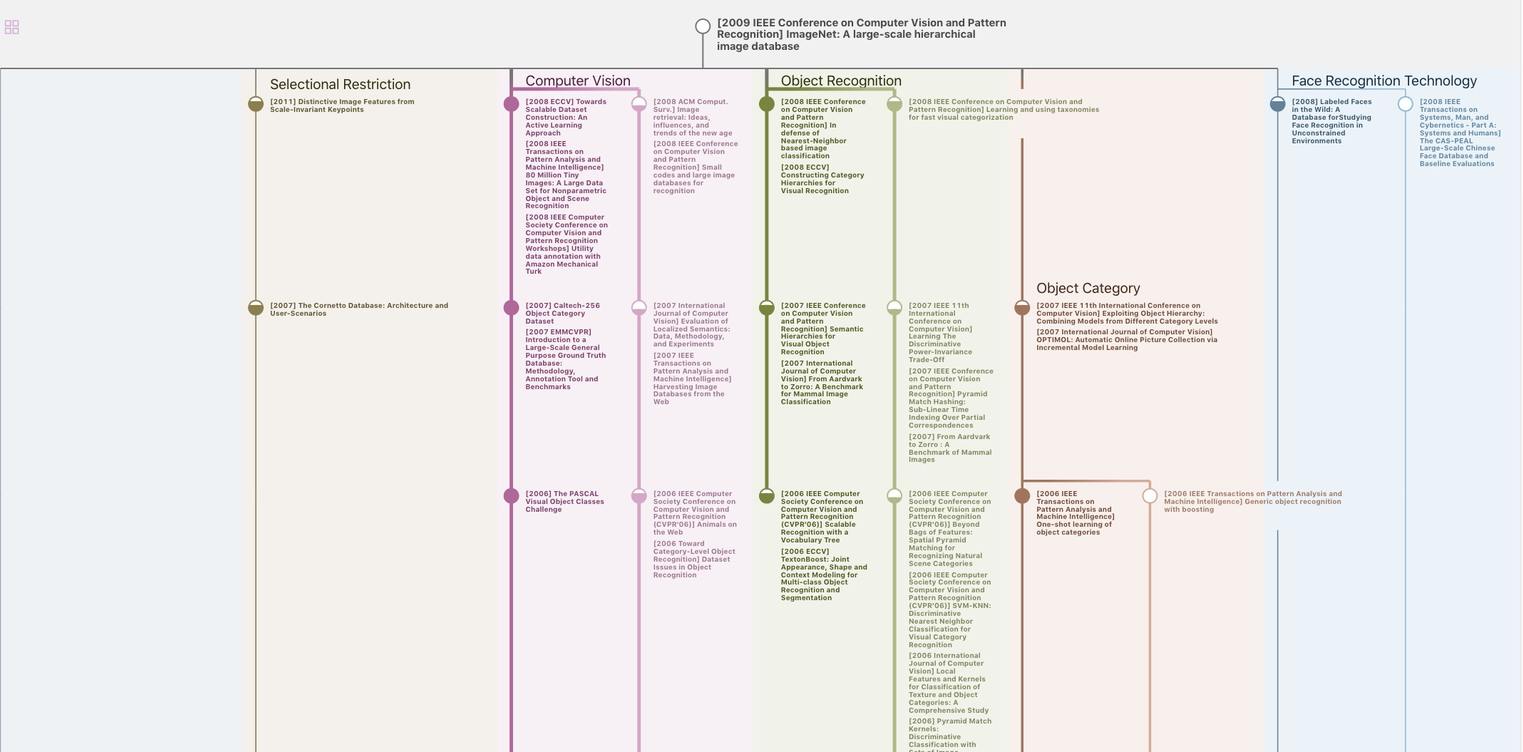
生成溯源树,研究论文发展脉络
Chat Paper
正在生成论文摘要