Deep Dilated Convolutional Network for Material Recognition
2018 Eighth International Conference on Image Processing Theory, Tools and Applications (IPTA)(2018)
摘要
Material is actually one of the intrinsic features for objects, consequently material recognition plays an important role in image understanding. For the same material, it may have various shapes and appearances, but keeps the same physical characteristic, which brings great challenges for material recognition. Most recent material recognition methods are based on image patches, and cannot give accurate segmentation results for each specific material. In this paper, we propose a deep learning based method to do pixel level material segmentation for whole images directly. In classical convolutional network, the spacial size of features becomes smaller and smaller with the increasing of convolutional layers, which loses the details for pixel-wise segmentation. Therefore we propose to use dilated convolutional layers to keep the details of features. In addition, the dilated convolutional features are combined with traditional convolutional features to remove the artifacts that are brough by dilated convolution. In the experiments, the proposed dilated network showed its effectiveness on the popular MINC dataset and its extended version.
更多查看译文
关键词
Material recognition,Dilated convolution network
AI 理解论文
溯源树
样例
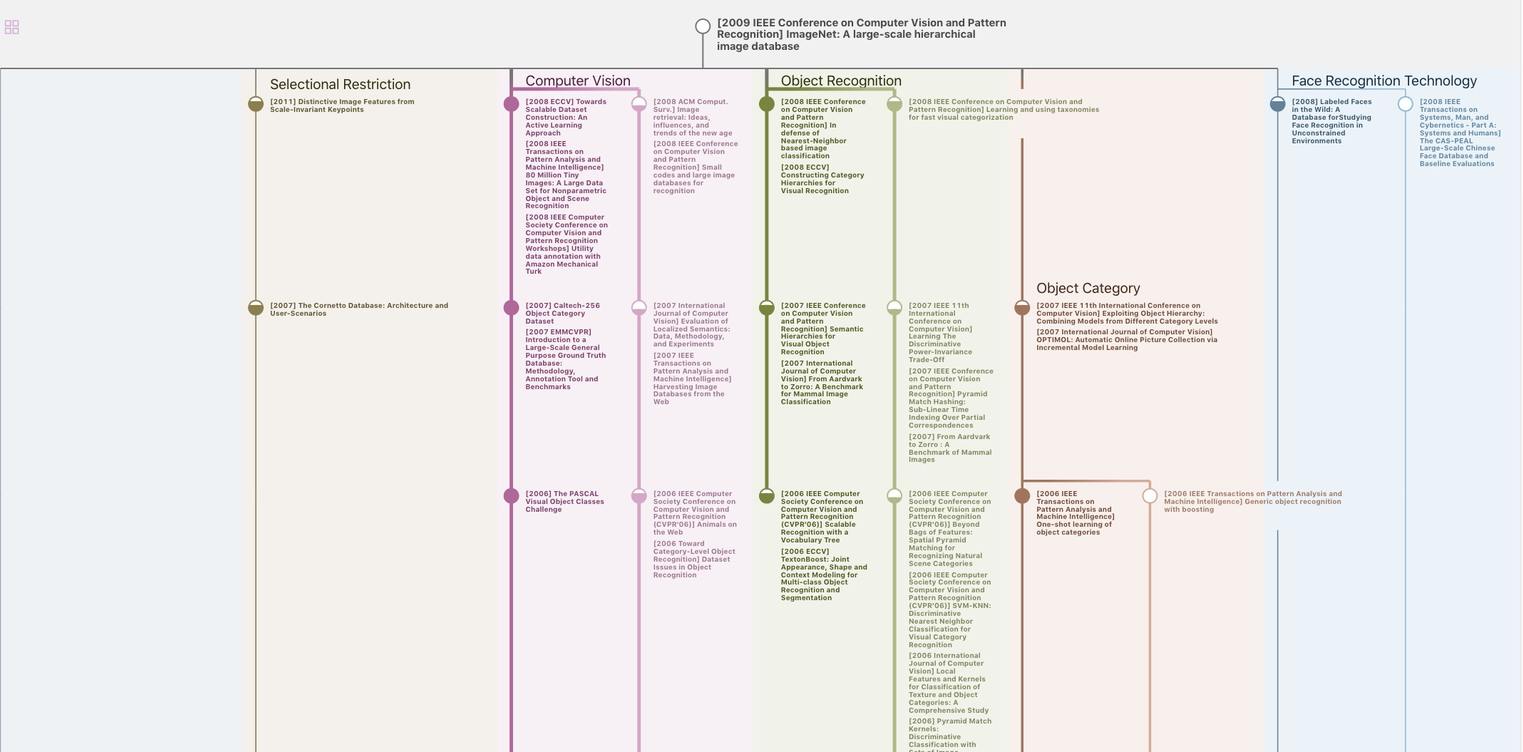
生成溯源树,研究论文发展脉络
Chat Paper
正在生成论文摘要