Long Short-Term Memory for Radio Frequency Spectral Prediction and its Real-Time FPGA Implementation
MILCOM 2018 - 2018 IEEE Military Communications Conference (MILCOM)(2018)
摘要
Reactive communication waveforms hosted in current generation tactical radios often fail to achieve good performance and resilience in highly dynamic and complex environments. Arguably, novel waveforms that can proactively adapt to anticipated channel conditions may better meet the challenges of the tactical environment. This motivates the ability to accurately predict spectral behaviour in real-time. A Long Short- Term Memory (LSTM) network is a type of recurrent neural network which has been extremely successful in dealing with time-dependent signal processing problems such as speech recognition and machine translation. In this paper, we apply it to the task of spectral prediction and present a module generator for a latency-optimised Field-Programmable Gate Array (FPGA) implementation. We show that our implementation obtains superior results to other time series prediction techniques including a naïve predictor, moving average and ARIMA for the problem of radio frequency spectral prediction. For a single LSTM layer plus a fully- connected output layer with 32 inputs and 32 outputs, we demonstrate that a prediction latency of 4.3 μ s on a Xilinx XC7K410T Kintex-7 FPGA is achievable.
更多查看译文
关键词
radio frequency spectral prediction,real-time FPGA implementation,reactive communication waveforms,anticipated channel conditions,tactical environment,recurrent neural network,time-dependent signal processing problems,speech recognition,machine translation,module generator,time series prediction techniques,single LSTM layer,Xilinx XC7K410T Kintex-7 FPGA,ARIMA,moving average,naïve predictor,latency-optimised field-programmable gate array,spectral behaviour prediction,generation tactical radios,long short-term memory network
AI 理解论文
溯源树
样例
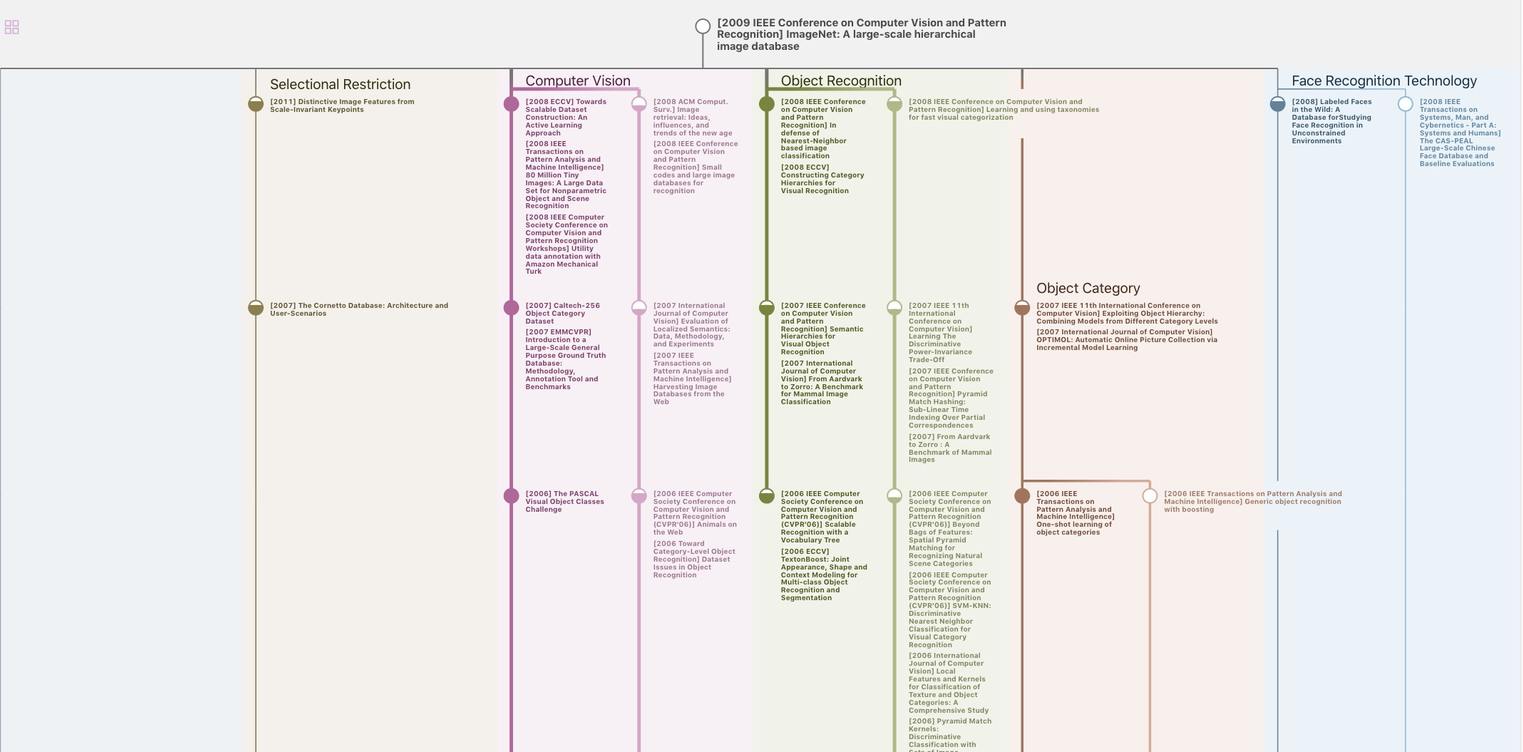
生成溯源树,研究论文发展脉络
Chat Paper
正在生成论文摘要