Online model-learning algorithm from samples and trajectories
Journal of Ambient Intelligence and Humanized Computing(2018)
摘要
Learning of the value function and the policy for continuous MDPs is non-trial due to the difficulty in collecting enough data. Model learning can use the collected data effectively, to learn a model and then use the learned model for planning, so as to accelerate the learning of the value function and the policy. Most of the existing works about model learning only concern the improvement of the single-step or multiple-step prediction, while the combination of them may be a better choice. Therefore, we propose an online algorithm where the samples for learning the model are both from the samples and from the trajectories, called Online-ML-ST. Other than the existing work, the trajectories collected in the interaction with the environment are not only used to learn the model offline, but also to learn the model, the value function and the policy online. The experiments are implemented in two typical continuous benchmarks such as the Pole Balancing and Inverted Pendulum, and the result shows that Online-ML-ST outperforms the other three typical methods in learning rate and convergence rate.
更多查看译文
关键词
Model learning,Planning,Multiple-step prediction,Continuous space,MDPs
AI 理解论文
溯源树
样例
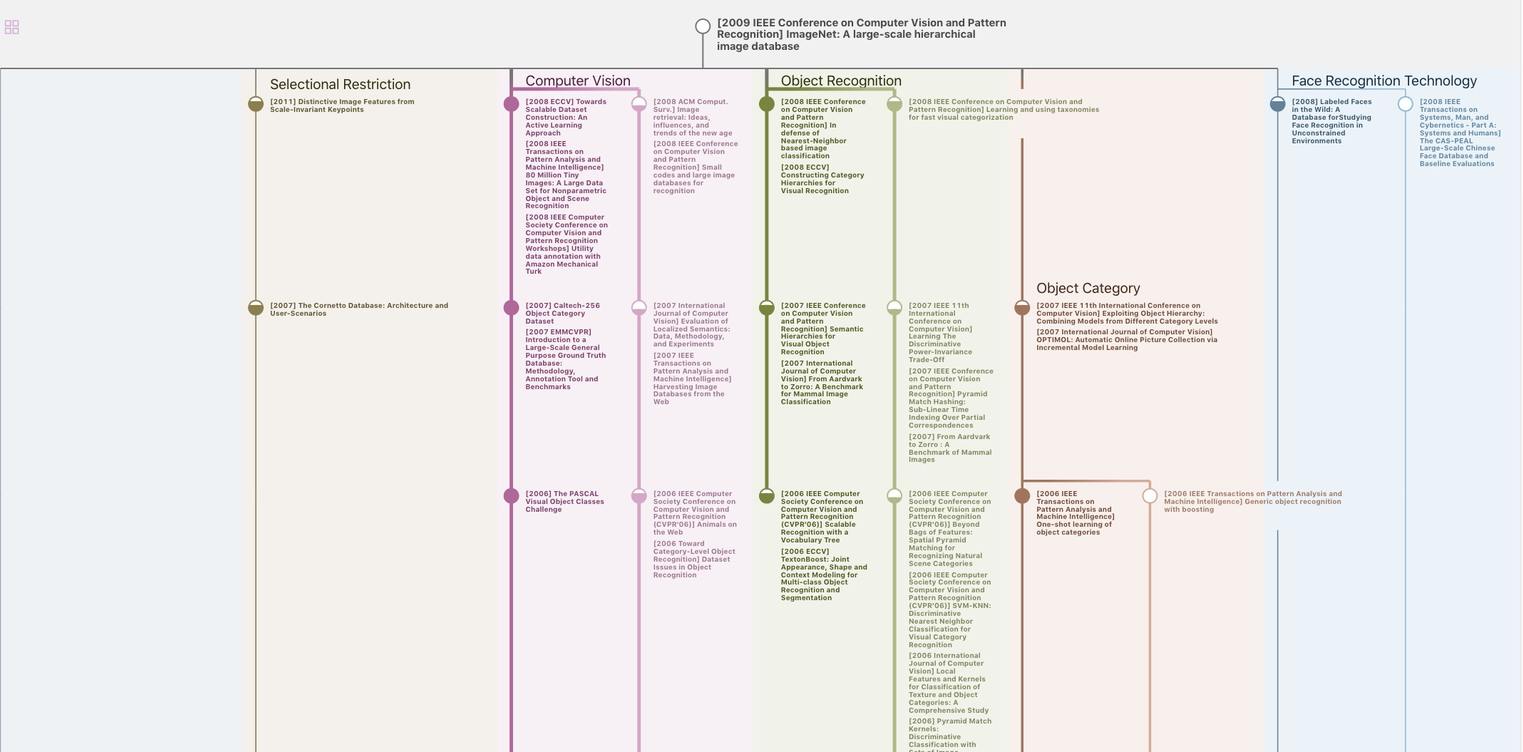
生成溯源树,研究论文发展脉络
Chat Paper
正在生成论文摘要