Designing Non-Greedy Reinforcement Learning Agents With Diminishing Reward Shaping
PROCEEDINGS OF THE 2018 AAAI/ACM CONFERENCE ON AI, ETHICS, AND SOCIETY (AIES'18)(2018)
摘要
This paper intends to address an issue in RL that when agents possessing varying capabilities, most resources may be acquired by stronger agents, leaving the weaker ones "starving". We introduce a simple method to train non-greedy agents in multi-agent reinforcement learning scenarios with nearly no extra cost. Our model can achieve the following goals in designing the non-greedy agent: non-homogeneous equality, only need local information, cost-effective, generalizable and configurable. We propose the idea of diminishing reward that makes the agent feel less satisfied for consecutive rewards obtained. This idea allows the agents to behave less greedy without the need to explicitly coding any ethical pattern nor monitor other agents' status. Given our framework, resources can be distributed more equally without running the risk of reaching homogeneous equality. We designed two games, Gathering Game and Hunter Prey to evaluate the quality of the model.
更多查看译文
关键词
multi-agent reinforcement learning, reward shaping, non-greedy
AI 理解论文
溯源树
样例
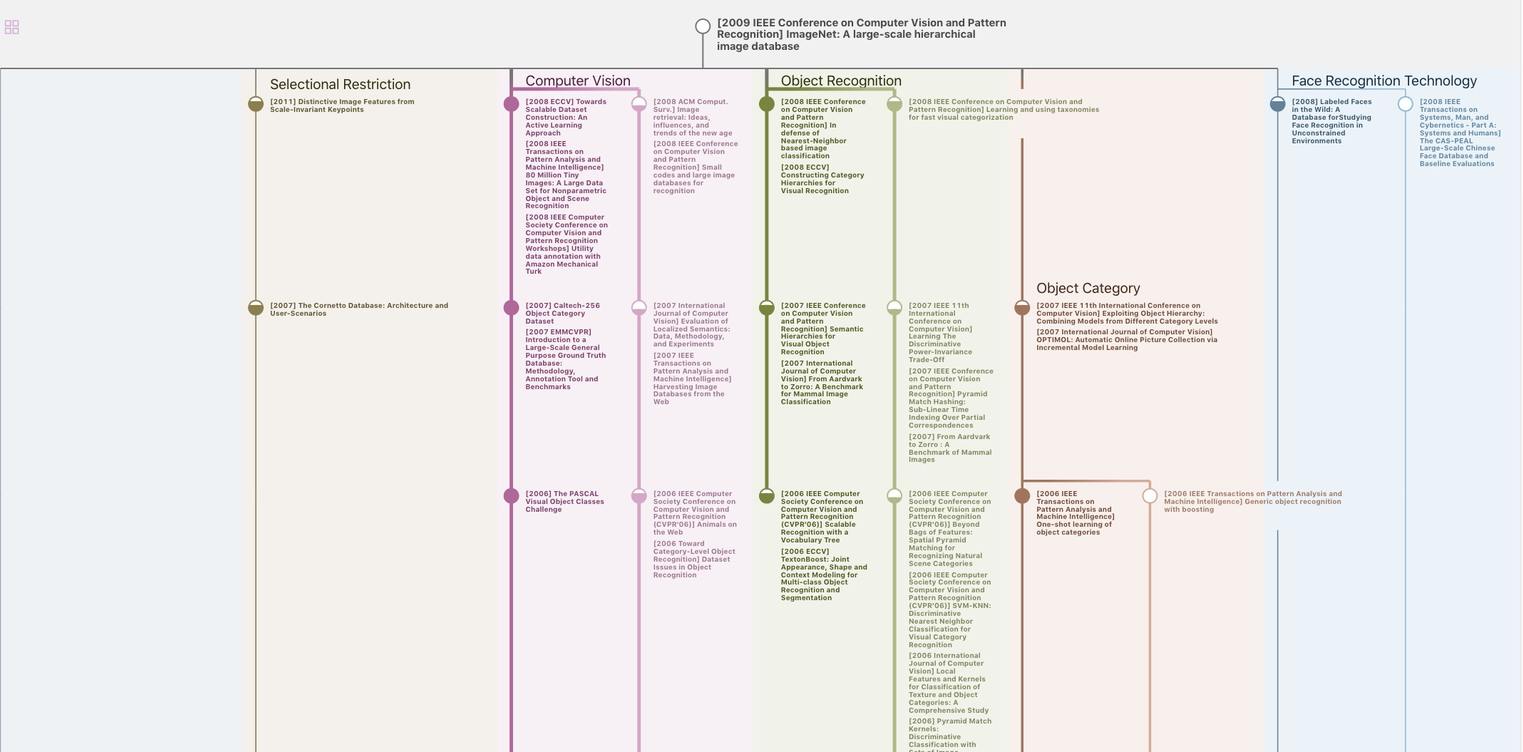
生成溯源树,研究论文发展脉络
Chat Paper
正在生成论文摘要