Real-time Convolutional Networks for Depth-based Human Pose Estimation
2018 IEEE/RSJ International Conference on Intelligent Robots and Systems (IROS)(2018)
摘要
We propose to combine recent Convolutional Neural Networks (CNN) models with depth imaging to obtain a reliable and fast multi-person pose estimation algorithm applicable to Human Robot Interaction (HRI) scenarios. Our hypothesis is that depth images contain less structures and are easier to process than RGB images while keeping the required information for human detection and pose inference, thus allowing the use of simpler networks for the task. Our contributions are threefold. (i) we propose a fast and efficient network based on residual blocks (called RPM) for body landmark localization from depth images; (ii) we created a public dataset DIH comprising more than 170k synthetic images of human bodies with various shapes and viewpoints as well as real (annotated) data for evaluation; (iii) we show that our model trained on synthetic data from scratch can perform well on real data, obtaining similar results to larger models initialized with pre-trained networks. It thus provides a good trade-off between performance and computation. Experiments on real data demonstrate the validity of our approach.
更多查看译文
关键词
convolutional neural networks models,human robot interaction,depth-based human pose estimation,pose inference,residual blocks,body landmark localization,depth imaging,human bodies,human detection,RGB images
AI 理解论文
溯源树
样例
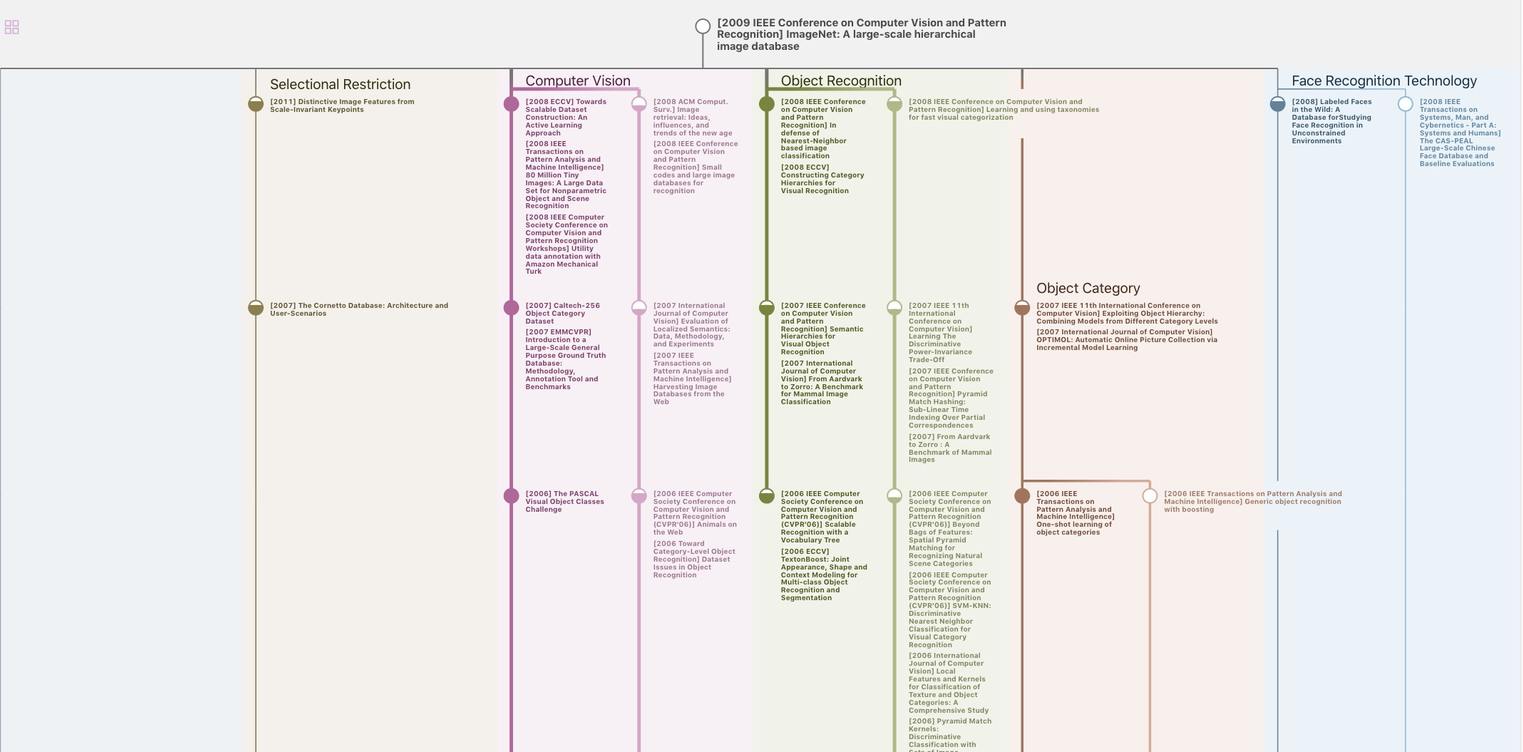
生成溯源树,研究论文发展脉络
Chat Paper
正在生成论文摘要