Learning to Run with Potential-Based Reward Shaping and Demonstrations from Video Data
2018 15th International Conference on Control, Automation, Robotics and Vision (ICARCV)(2018)
摘要
Learning to produce efficient movement behaviour for humanoid robots from scratch is a hard problem, as has been illustrated by the “Learning to run” competition at NIPS 2017. The goal of this competition was to train a two-legged model of a humanoid body to run in a simulated race course with maximum speed. All submissions took a tabula rasa approach to reinforcement learning (RL) and were able to produce relatively fast, but not optimal running behaviour. In this paper, we demonstrate how data from videos of human running (e.g. taken from YouTube) can be used to shape the reward of the humanoid learning agent to speed up the learning and produce a better result. Specifically, we are using the positions of key body parts at regular time intervals to define a potential function for potential-based reward shaping (PBRS). Since PBRS does not change the optimal policy, this approach allows the RL agent to overcome sub-optimalities in the human movements that are shown in the videos. We present experiments in which we combine selected techniques from the top ten approaches from the NIPS competition with further optimizations to create an high-performing agent as a baseline. We then demonstrate how video-based reward shaping improves the performance further, resulting in an RL agent that runs twice as fast as the baseline in 12 hours of training. We furthermore show that our approach can overcome sub-optimal running behaviour in videos, with the learned policy significantly outperforming that of the running agent from the video.
更多查看译文
关键词
two-legged model,humanoid body,simulated race course,maximum speed,tabula rasa approach,reinforcement learning,human running,humanoid learning agent,key body parts,potential function,potential-based reward shaping,PBRS,optimal policy,RL agent,sub-optimalities,human movements,NIPS competition,sub-optimal running behaviour,learned policy,running agent,video data,humanoid robots,hard problem,NIPS 2017
AI 理解论文
溯源树
样例
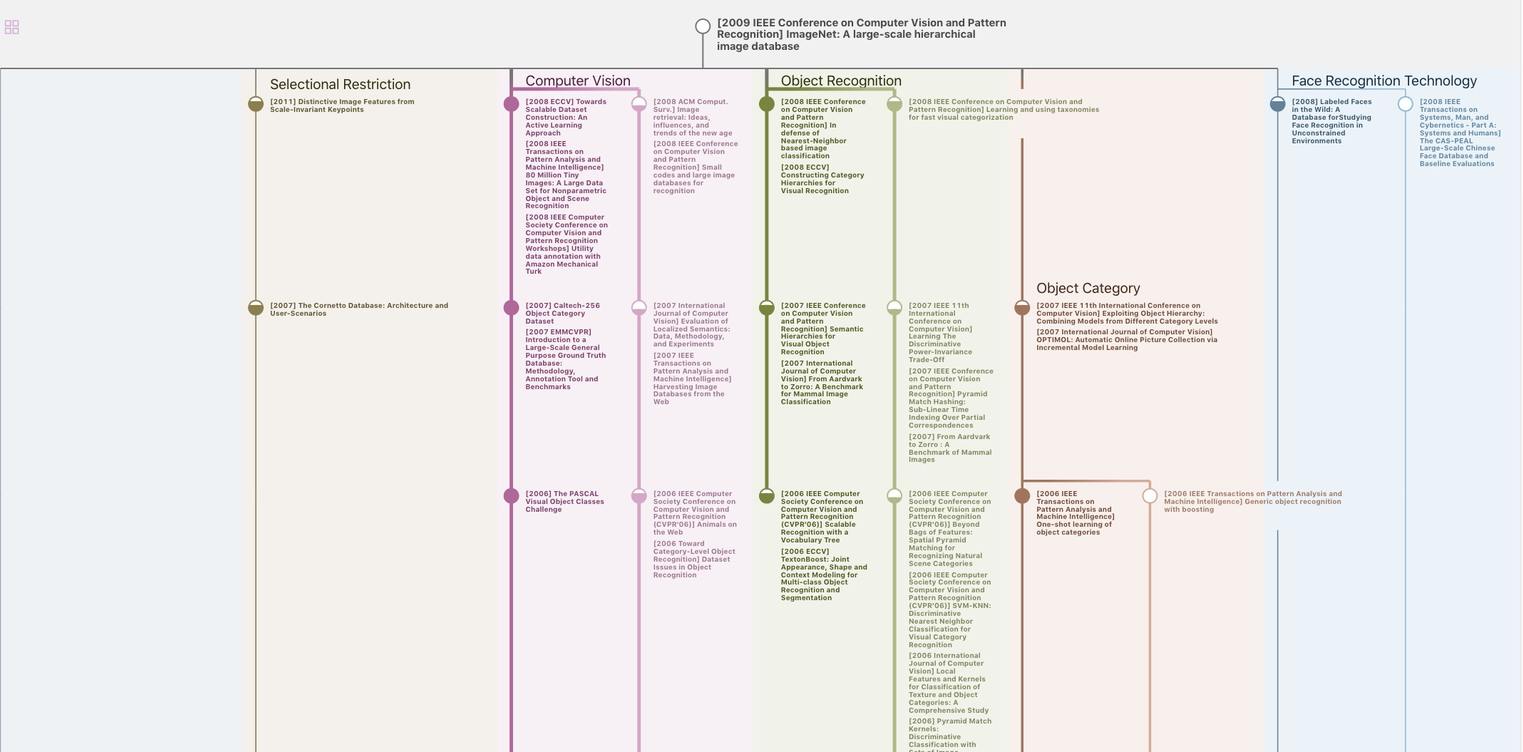
生成溯源树,研究论文发展脉络
Chat Paper
正在生成论文摘要