A Bayesian Blind Source Separation Method for a Linear-quadratic Model
2018 26th European Signal Processing Conference (EUSIPCO)(2018)
摘要
We propose a blind source separation method based on Bayesian inference in order to separate two sources in a linear-quadratic mixing model. This nonlinear model describes for example the response of a non-selective metal-oxide gas sensor (MOX) in presence of two gases such as acetone and ethanol diluted in an air buffer. In order to quantify the gas components, it is necessary to inverse the linear-quadratic model. In addition, we look for reducing the number of samples for the calibration step. Therefore, we here propose a Bayesian blind source separation method, with only few points of calibration and which is based on Monte Carlo Markov Chain (MCMC) sampling methods to estimate the mean of the posterior distribution. We analyze the performance on a set of simulated samples. We use a cross-validation approach, with three steps: first, we blindly estimate the mixing coefficients and sources; second, we correct the scale factors thanks to few calibration samples; and third, we validate the method on validation samples, estimating sources thanks to mixing coefficients estimated before on the calibration samples. We compare this unsupervised nonlinear method with a supervised method to evaluate the performance with respect to the number of calibration points: with 10 calibration points instead of 160, the performance achieves 11 dB, with a loss limited to 1.5 dB.
更多查看译文
关键词
Linear-quadratic model,Inverse problems,Statistical signal processing,Bayesian method,Blind source separation,Monte Carlo Markov Chain (MCMC),Gas mixture,MOX sensor
AI 理解论文
溯源树
样例
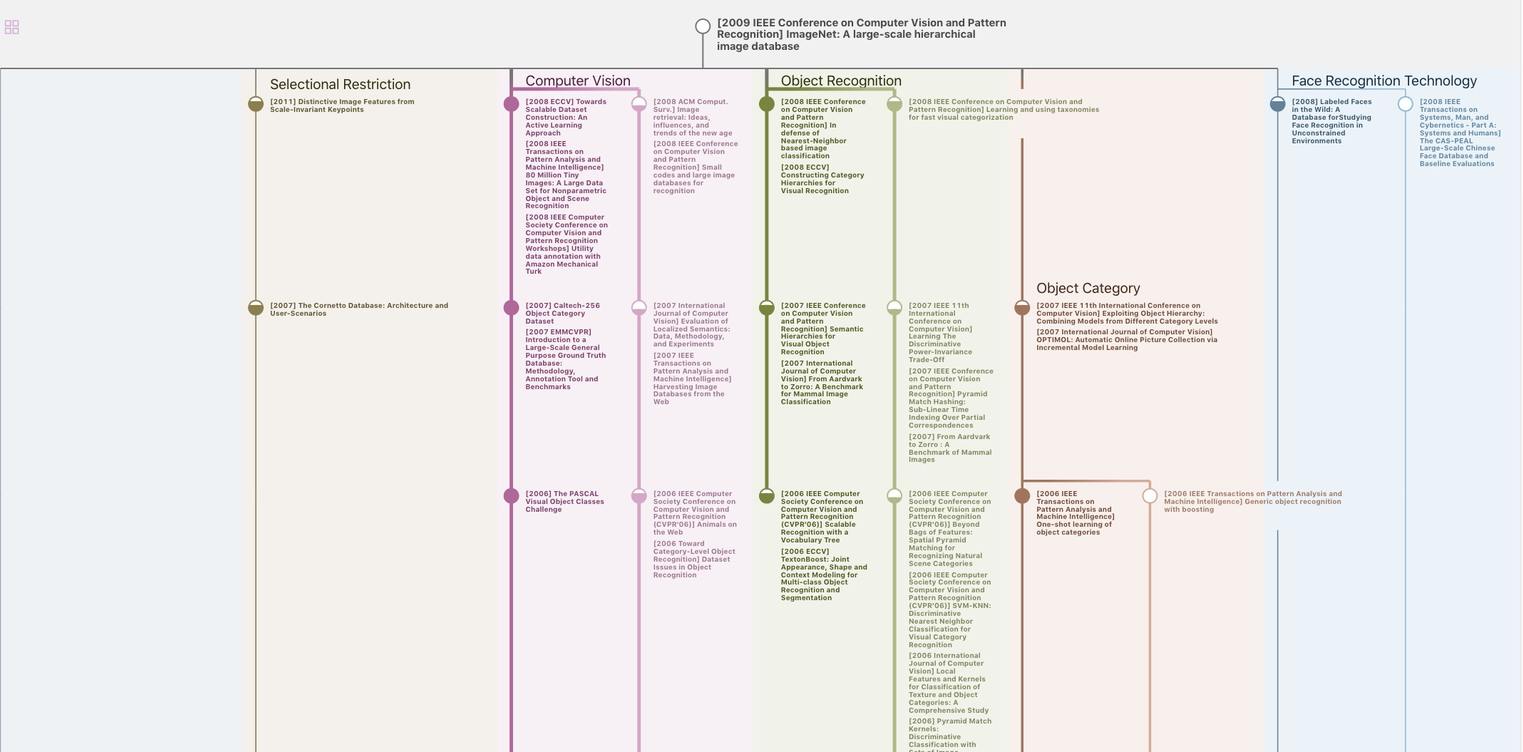
生成溯源树,研究论文发展脉络
Chat Paper
正在生成论文摘要