Data center cooling using model-predictive control
ADVANCES IN NEURAL INFORMATION PROCESSING SYSTEMS 31 (NIPS 2018)(2018)
Abstract
Despite the impressive recent advances in reinforcement learning (RL) algorithms, their deployment to real-world physical systems is often complicated by unexpected events, limited data, and the potential for expensive failures. In this paper, we describe an application of RL "in the wild" to the task of regulating temperatures and airflow inside a large-scale data center (DC). Adopting a data-driven, model-based approach, we demonstrate that an RL agent with little prior knowledge is able to effectively and safely regulate conditions on a server floor after just a few hours of exploration, while improving operational efficiency relative to existing PID controllers.
MoreTranslated text
Key words
reinforcement learning,mathematical model,rl agent,unexpected events,operational efficiency
AI Read Science
Must-Reading Tree
Example
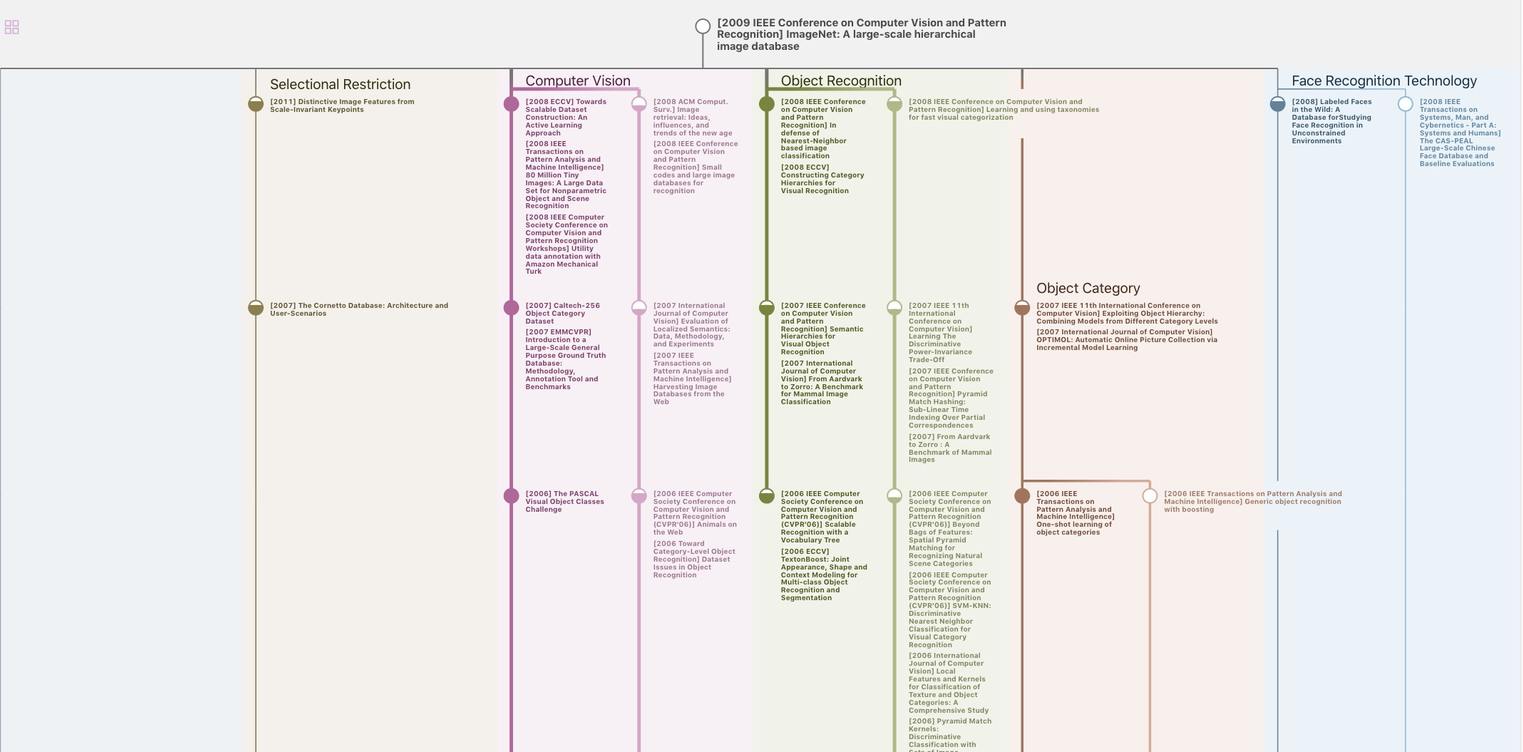
Generate MRT to find the research sequence of this paper
Chat Paper
Summary is being generated by the instructions you defined