PRISMO - Priority Based Spam Detection Using Multi Optimization.
BDA(2018)
摘要
The rapid growth of social networking sites such as Twitter, Facebook, Google+, MySpace, Snapchat, Instagram, etc., along with its local invariants such as Weibo, Hyves, etc., has made them infiltrated with a large amount of spamming activities. Based on the features, an account or content can be classified as spam or benign. The presence of some irrelevant features decreases the performance of the classifier, understandability of dataset, and the time requirement for training and classification increases. Therefore, Feature subset selection is an essential phase in the process of machine learning mechanism. The objective of feature subset selection is to choose a subset of size ‘s’ (s u003c n) from the total set of ‘n’ features that results in the least classification error. The feature subset selection problem can be represented as a problem of optimization in which the objective is to choose the near-optimal subset of features. Based on the literature survey, it is found that the classifier will offer its best performance if the data with high dimension is reduced such that it includes only appropriate features having lesser redundancy. The contribution of this paper comprises feature subset and its cost optimization simultaneously. The fundamental aspect PRISMO is to generate a primary feature subset through various optimization algorithms for the initialization stage. Further, the subset has been generated using the initial feature set based on their priority using basic rules of conjunction and disjunction. To evaluate the overall efficiency of PRISMO, various experiments were carried out using different dataset. The obtained result shows that the proposed model effectively reduces the cardinality of features without any bias to a specific dataset and any degradation to the classifier accurateness.
更多查看译文
关键词
Dimensionality reduction, Genetic Algorithm, Machine learning, Spamming, Particle Swarm Optimization, Web 2.0
AI 理解论文
溯源树
样例
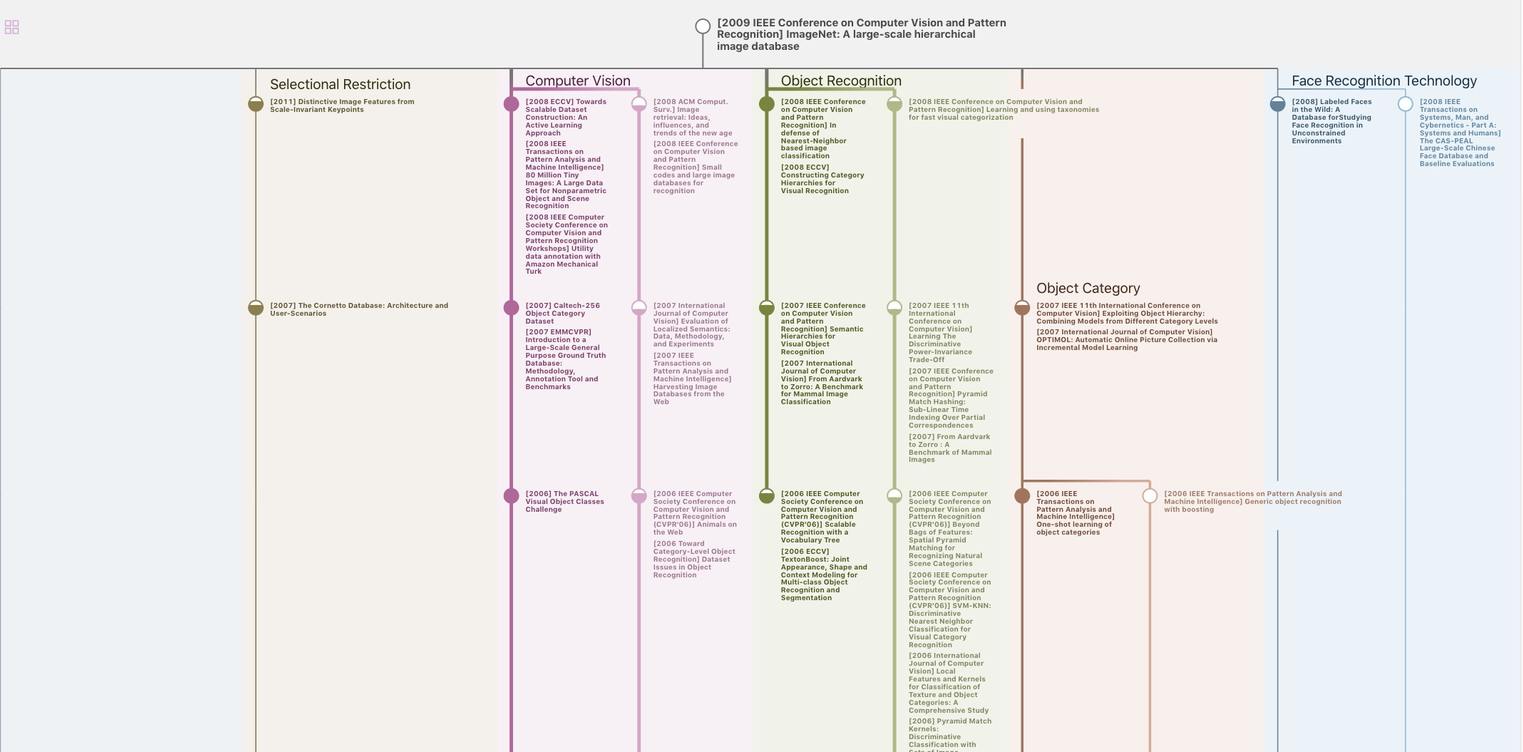
生成溯源树,研究论文发展脉络
Chat Paper
正在生成论文摘要