Characterizing DNN Models for Edge-Cloud Computing
2018 IEEE International Symposium on Workload Characterization (IISWC)(2018)
摘要
Traditionally Deep Neural Networks (DNNs) services are deployed in the cloud, due to the computation-intensive DNN models. In recent years, as emerging edge computing provides new possibilities for DNN applications, we have opportunities to process DNN models in the cloud and on the device collaboratively, i.e., edge-cloud computing. Since cloud and edge devices demonstrate significant diversity on inference latency, network transmission overhead, memory capacity and power consumption, it is a big challenge to determine the DNN model deployment in the cloud and on edge devices. In this paper, we characterize the behaviours of three types of DNN models, i.e., CNN, LSTM and MLP, on four types of platforms, i.e., server-class CPU, server-class GPU, embedded device with GPU, and smart-phones. Our experimental results demonstrate that we can carefully tune a deployment strategy for DNN models in the cloud, and on big and (or) little cores of the edge device, to balance performance and power consumption.
更多查看译文
关键词
edge-cloud computing,Deep Neural Networks services,computation-intensive DNN models,edge devices,cloud devices,CNN,LSTM,MLP
AI 理解论文
溯源树
样例
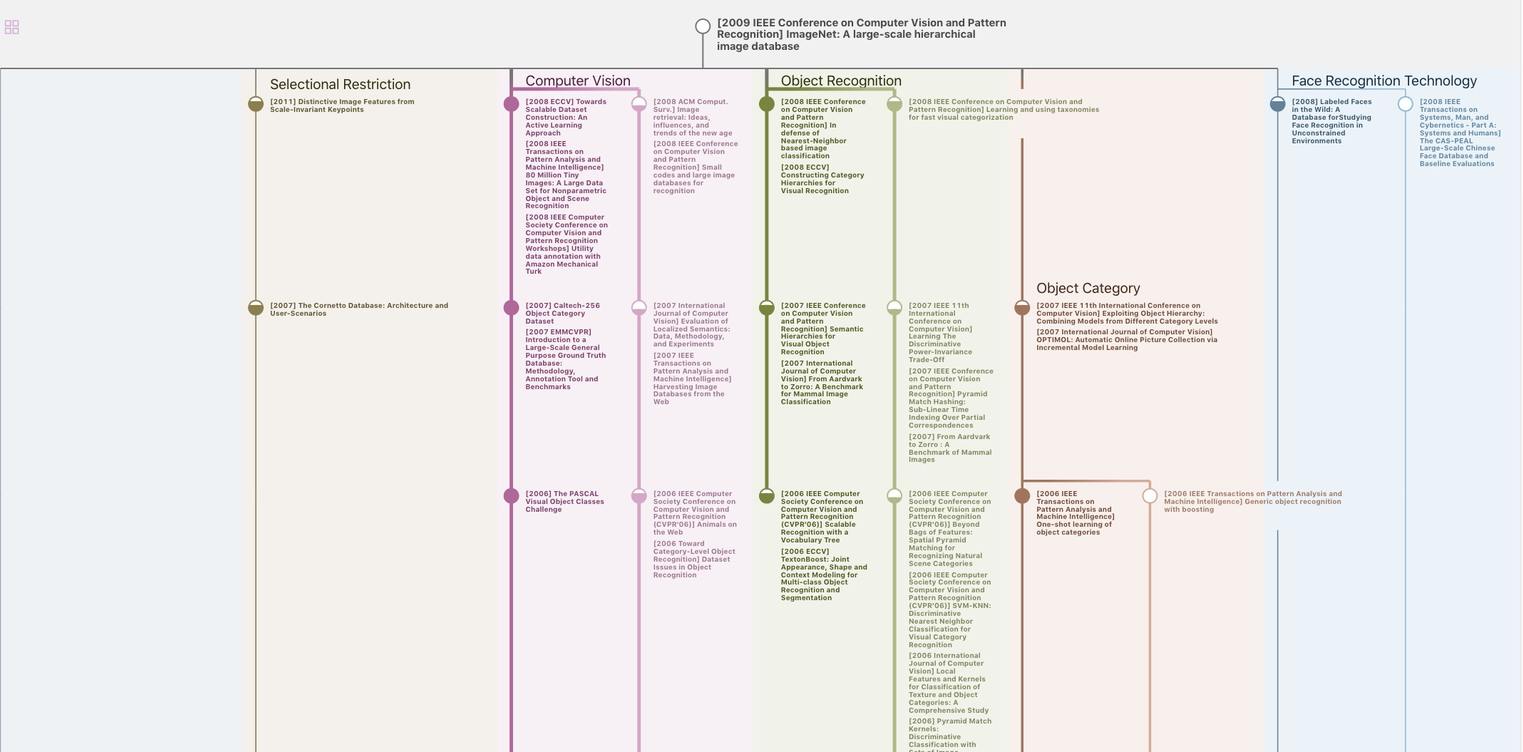
生成溯源树,研究论文发展脉络
Chat Paper
正在生成论文摘要