A multitask multiview clustering algorithm in heterogeneous situations based on LLE and LE.
Knowledge-Based Systems(2019)
摘要
Multi-view clustering and multi-task clustering attract much attention in recent years. With the development of data mining, a new learning scenario containing the properties of multi-task and multi-view together appears, which called multi-task multi-view learning. Existing multi-task multi-view learning usually applies for classification and considers that all tasks share the same class label sets. Nevertheless, there is not much information about label sets in real world applications and it is difficult for all learning tasks to contain the same label sets. Hence, in order to overcome the two limitations, we propose a multi-task multi-view clustering algorithm in heterogeneous situations based on Locally Linear Embedding (LLE) and Laplacian Eigenmaps (LE) methods (L3E-M2VC). It maps the samples of multiple views from each task to a common view space firstly, then transforms the samples to a discriminative task space secondly, and finally exploits K-Means for clustering. Experiments on several multi-task multi-view data sets are evaluated by RI and CA and the results show that our L3E-M2VC outperforms the other 11 methods, including single-task single-view, multi-view, multi-task, multi-view multi-task algorithms and the varieties of our method.
更多查看译文
关键词
Data mining,Clustering,Multi-task multi-view
AI 理解论文
溯源树
样例
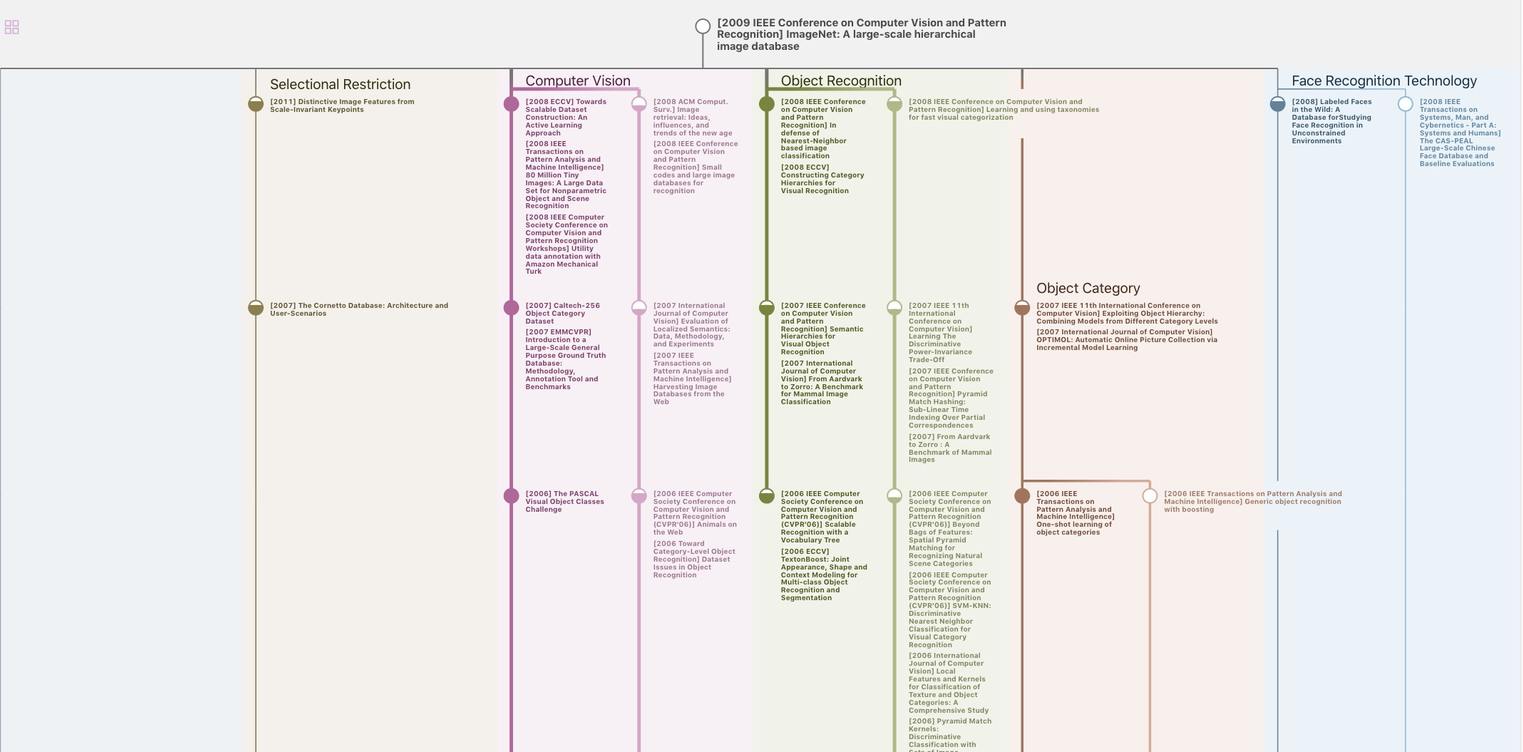
生成溯源树,研究论文发展脉络
Chat Paper
正在生成论文摘要