Noise-Tolerant Techniques for Decomposition-Based Multiobjective Evolutionary Algorithms.
IEEE Transactions on Cybernetics(2020)
摘要
Over the last few decades, the decomposition-based multiobjective evolutionary algorithms (DMOEAs) have became one of the mainstreams for multiobjective optimization. However, there is not too much research on applying DMOEAs to uncertain problems until now. Usually, the uncertainty is modeled as additive noise in the objective space, which is the case this paper concentrates on. This paper first carries out experiments to examine the impact of noisy environments on DMOEAs. Then, four noise-handling techniques based upon the analyses of empirical results are proposed. First, a Pareto-based nadir point estimation strategy is put forward to provide a good normalization of each objective. Next, we introduce two adaptive sampling strategies that vary the number of samples used per solution based on the differences among neighboring solutions and their variance to control the tradeoff between exploration and exploitation. Finally, a mixed objective evaluation strategy and a mixed repair mechanism are proposed to alleviate the effects of noise and remedy the loss of diversity in the decision space, respectively. These features are embedded in two popular DMOEAs (i.e., MOEA/D and DMOEA-
${\varepsilon }\text{C}$
), and DMOEAs with these features are called noise-tolerant DMOEAs (NT-DMOEAs). NT-DMOEAs are compared with their various variants and four noise-tolerant multiobjective algorithms, including the improved NSGA-II, the classical algorithm Bayesian (1+1)-ES (BES), and the state-of-the-art algorithms MOP-EA and rolling tide evolutionary algorithm to show the superiority of proposed features on 17 benchmark problems with different strength levels of noise. Experimental studies demonstrate that two NT-DMOEAs, especially NT-DMOEA-
${\varepsilon }\text{C}$
, show remarkable advantages over competitors in the majority of test instances.
更多查看译文
关键词
Optimization,Noise measurement,Estimation,Benchmark testing,Maintenance engineering,Evolutionary computation,Uncertainty
AI 理解论文
溯源树
样例
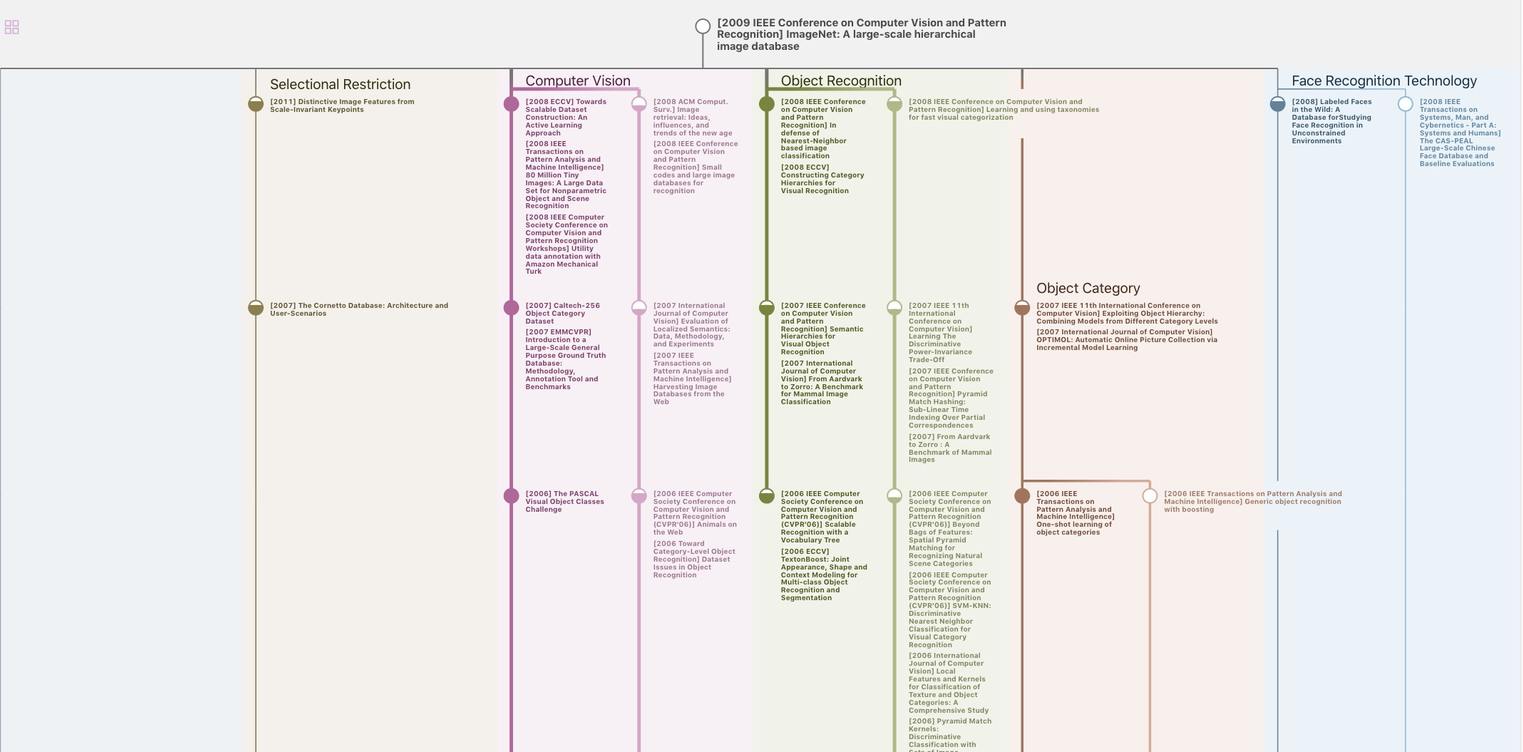
生成溯源树,研究论文发展脉络
Chat Paper
正在生成论文摘要