A workflow for identifying metabolically active chemicals to complement in vitro toxicity screening
Computational Toxicology(2018)
摘要
The new paradigm of toxicity testing approaches involves rapid screening of thousands of chemicals across hundreds of biological targets through use of in vitro assays. Such assays may lead to false negatives when the complex metabolic processes that render a chemical bioactive in a living system are unable to be replicated in an in vitro environment. In the current study, a workflow is presented for complementing in vitro testing results with in silico and in vitro techniques to identify inactive parents that may produce active metabolites. A case study applying this workflow involved investigating the influence of metabolism for over 1,400 chemicals considered inactive across 18 in vitro assays related to the estrogen receptor (ER) pathway. Over 7,500 first-generation and second-generation metabolites were generated for these in vitro inactive chemicals using an in silico software program. Next, a consensus model comprised of four individual quantitative structure activity relationship (QSAR) models was used to predict ER-binding activity for each of the metabolites. Binding activity was predicted for ∼8–10% of metabolites in each generation, with these metabolites linked to 259 in vitro inactive parent chemicals. Metabolites were enriched in substructures consisting of alcohol, aromatic, and phenol bonds relative to their inactive parent chemicals, suggesting these features are potentially favorable for ER-binding. The workflow presented here can be used to identify parent chemicals that can be potentially bioactive, to aid confidence in high throughput risk screening.
更多查看译文
关键词
Metabolite prediction,QSAR,ER,in vitro screening
AI 理解论文
溯源树
样例
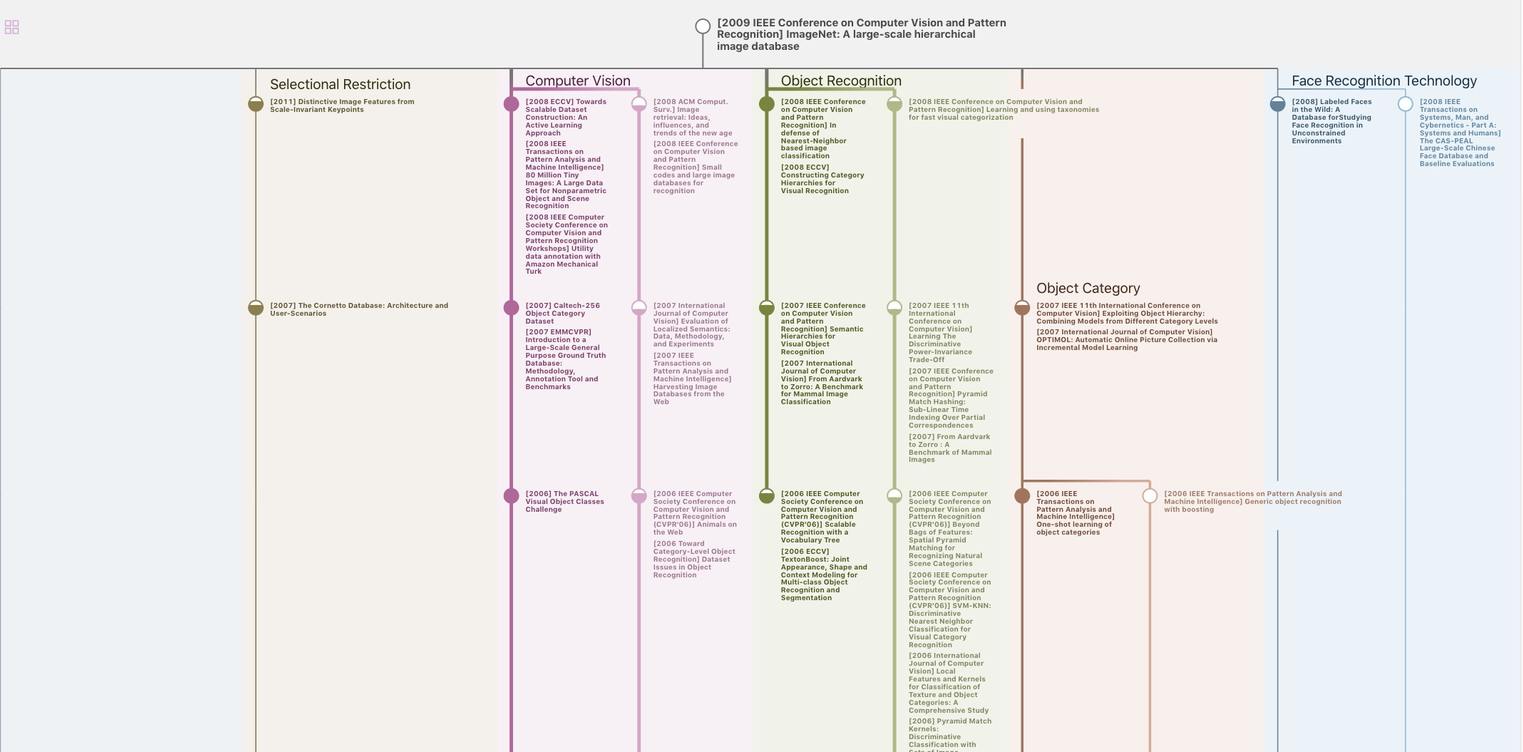
生成溯源树,研究论文发展脉络
Chat Paper
正在生成论文摘要