Use of machine learning to predict early biochemical recurrence following robotic prostatectomy.
BJU INTERNATIONAL(2019)
摘要
ObjectivesTo train and compare machine-learning algorithms with traditional regression analysis for the prediction of early biochemical recurrence after robot-assisted prostatectomy. Patients and MethodsA prospectively collected dataset of 338 patients who underwent robot-assisted prostatectomy for localized prostate cancer was examined. We used three supervised machine-learning algorithms and 19 different training variables (demographic, clinical, imaging and operative data) in a hypothesis-free manner to build models that could predict patients with biochemical recurrence at 1 year. We also performed traditional Cox regression analysis for comparison. ResultsK-nearest neighbour, logistic regression and random forest classifier were used as machine-learning models. Classic Cox regression analysis had an area under the curve (AUC) of 0.865 for the prediction of biochemical recurrence. All three of our machine-learning models (K-nearest neighbour (AUC 0.903), random forest tree (AUC 0.924) and logistic regression (AUC 0.940) outperformed the conventional statistical regression model. Accuracy prediction scores for K-nearest neighbour, random forest tree and logistic regression were 0.976, 0.953 and 0.976, respectively. ConclusionsMachine-learning techniques can produce accurate disease predictability better that traditional statistical regression. These tools may prove clinically useful for the automated prediction of patients who develop early biochemical recurrence after robot-assisted prostatectomy. For these patients, appropriate individualized treatment options can improve outcomes and quality of life.
更多查看译文
关键词
predictive model,prostate cancer,biochemical recurrence,machine learning
AI 理解论文
溯源树
样例
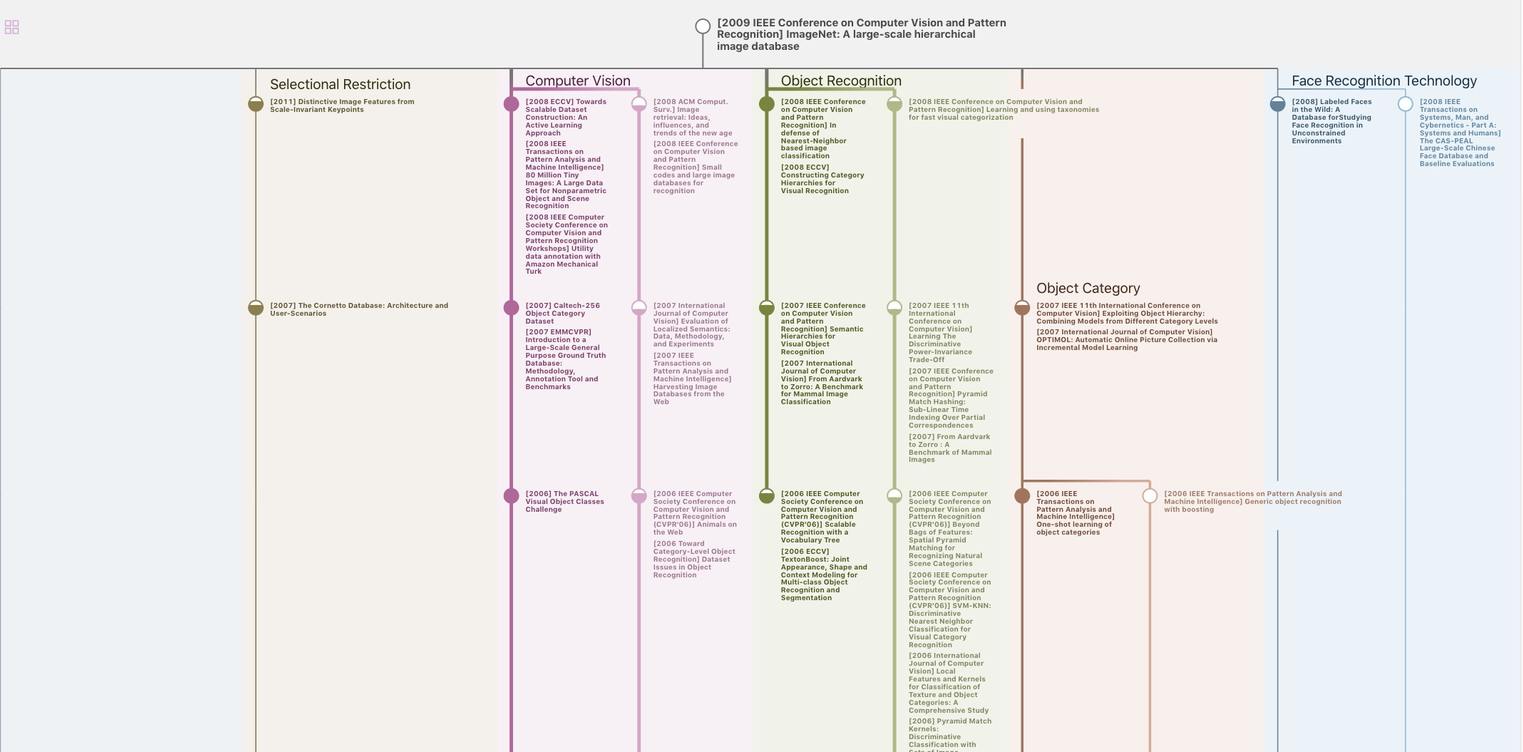
生成溯源树,研究论文发展脉络
Chat Paper
正在生成论文摘要