Multi-Interest Refinement by Collaborative Attributes Modeling for Click-Through Rate Prediction
Conference on Information and Knowledge Management(2022)
摘要
ABSTRACTLearning interest representation plays a core role in click-through rate prediction task. Existing Transformer-based approaches learn multi-interests from a sequence of interacted items with rich attributes. The attention weights explain how relevant an item's specific attribute sequence is to the user's interest. However, it implicitly assumes the independence of attributes regarding the same item, which may not always hold in practice. Empirically, the user places varied emphasis on different attributes to consider whether interacting with one item, which is unobserved. Independently modeling each attribute may allow attention to assign probability mass to some unimportant attributes. Collaborative attributes of varied emphasis can be incorporated to help the model more reasonably approximate attributes' relevance to others and generate refined interest representations. To this end, we novelly propose to integrate a dynamic collaborative attribute routing module into Transformer. The module assigns collaborative scores to each attribute of clicked items and induces the extended Transformer to prioritize the influential attributes. To learn collaborative scores without labels, we design a diversity loss to facilitate score differentiation. The comparison with baselines on two real-world benchmark datasets and one industrial dataset validates the effectiveness of the framework.
更多查看译文
关键词
collaborative attributes modeling,rate,prediction,multi-interest,click-through
AI 理解论文
溯源树
样例
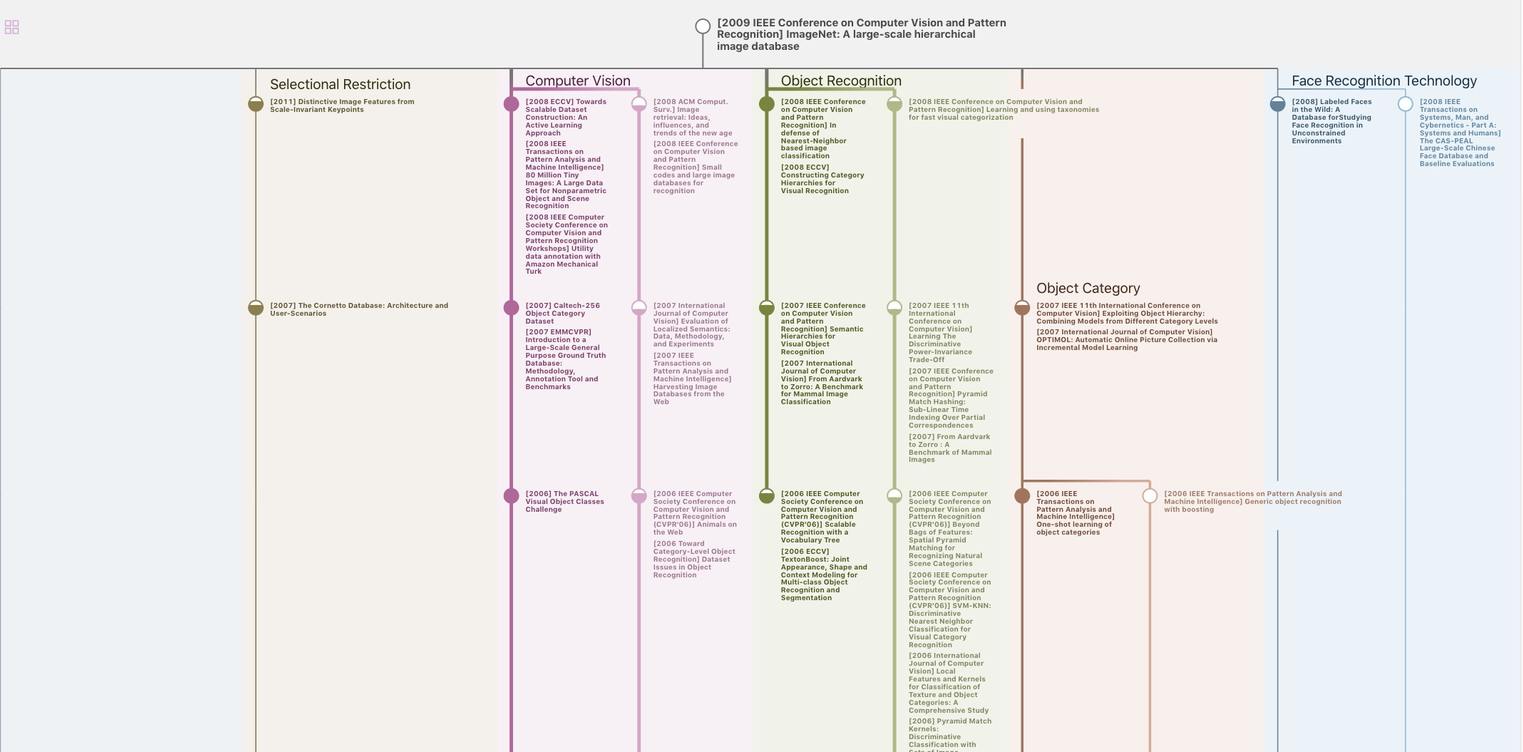
生成溯源树,研究论文发展脉络
Chat Paper
正在生成论文摘要