A comparative study on large scale kernelized support vector machines
Adv. Data Analysis and Classification(2016)
摘要
Kernelized support vector machines (SVMs) belong to the most widely used classification methods. However, in contrast to linear SVMs, the computation time required to train such a machine becomes a bottleneck when facing large data sets. In order to mitigate this shortcoming of kernel SVMs, many approximate training algorithms were developed. While most of these methods claim to be much faster than the state-of-the-art solver LIBSVM, a thorough comparative study is missing. We aim to fill this gap. We choose several well-known approximate SVM solvers and compare their performance on a number of large benchmark data sets. Our focus is to analyze the trade-off between prediction error and runtime for different learning and accuracy parameter settings. This includes simple subsampling of the data, the poor-man’s approach to handling large scale problems. We employ model-based multi-objective optimization, which allows us to tune the parameters of learning machine and solver over the full range of accuracy/runtime trade-offs. We analyze (differences between) solvers by studying and comparing the Pareto fronts formed by the two objectives classification error and training time. Unsurprisingly, given more runtime most solvers are able to find more accurate solutions, i.e., achieve a higher prediction accuracy. It turns out that LIBSVM with subsampling of the data is a strong baseline. Some solvers systematically outperform others, which allows us to give concrete recommendations of when to use which solver.
更多查看译文
关键词
62-07 Data analysis, Large scale, Machine learning, Multi-objective optimization, Nonlinear SVM, Parameter tuning, Supervised learning, Support vector machine
AI 理解论文
溯源树
样例
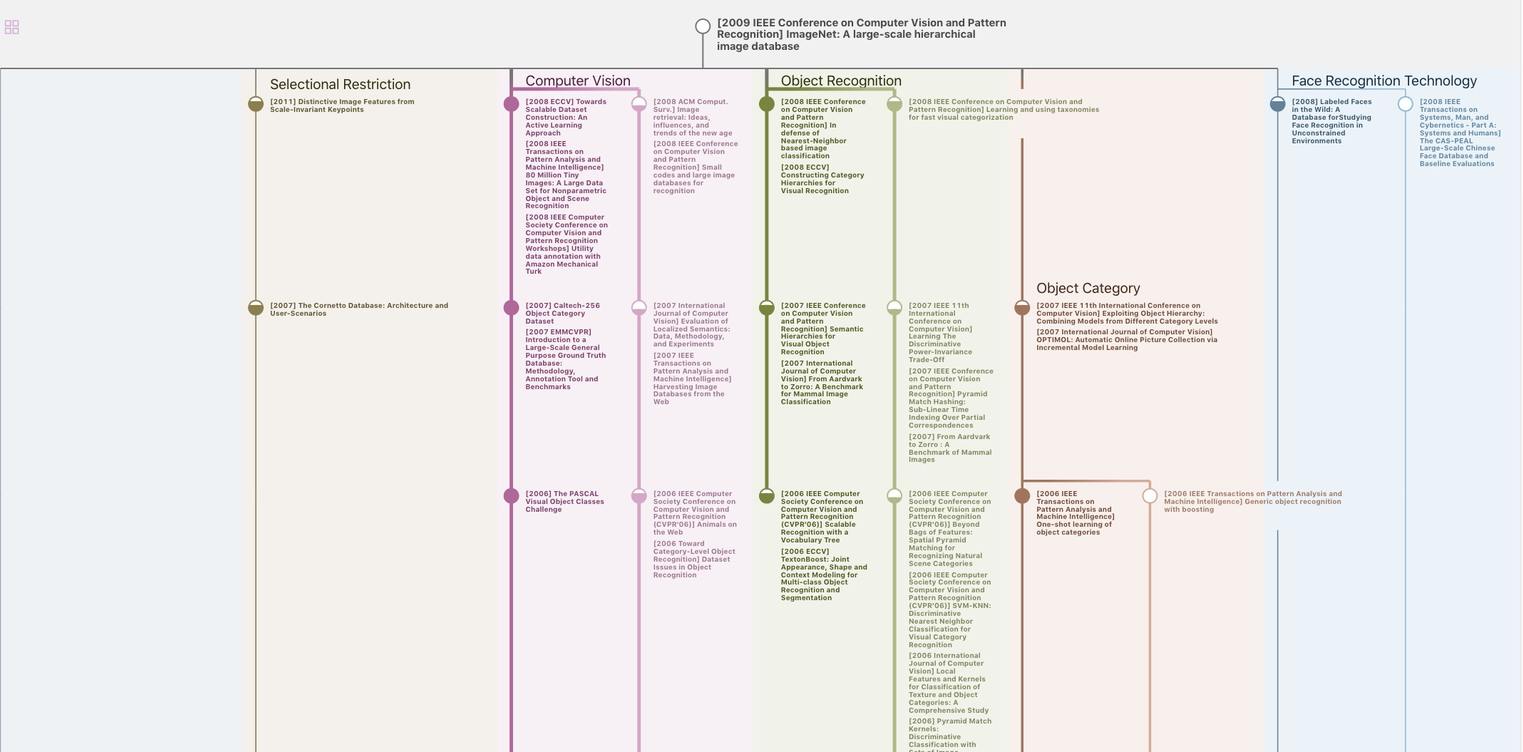
生成溯源树,研究论文发展脉络
Chat Paper
正在生成论文摘要