Efficient algorithms for graph regularized PLSA for probabilistic topic modeling.
Pattern Recognition(2019)
摘要
•We propose efficient algorithms for graph-regularized PLSA (GPLSA) as a general framework for single- or multi- modality topic analysis, where the graph regularizer is based on the divergence between discrete probability distributions. Similarities between topics are enforced in a joint latent space constraint by the graph, and topic distributions are enhanced by their nearest neighbors on the graph.•We show improved results for the L1 regularizer over the baseline. For L2 divergence as the regularizer, our GPLSA algorithm is more efficient and with a convergence guarantee than the existing method based on graph-regularized non-negative matrix factorization (GNMF). We further describe a new algorithm using symmetric KL divergences as the regularizer, and demonstrate that it is more effective compared to the L2 divergence.•The proposed algorithms extend naturally probabilistic topic analysis of a single modality to multiple modalities. Our method enables capturing of similarities between documents across modalities, by learning a joint latent space for documents of different modalities. Our topic learning representation leverages the compatible yet complementary conceptual themes among each modality. Thus it is more effective than other methods relying on features derived from direct concatenation of modalities.
更多查看译文
关键词
Probabilistic latent semantic analysis,Graph regularization,Topic analysis,Clustering
AI 理解论文
溯源树
样例
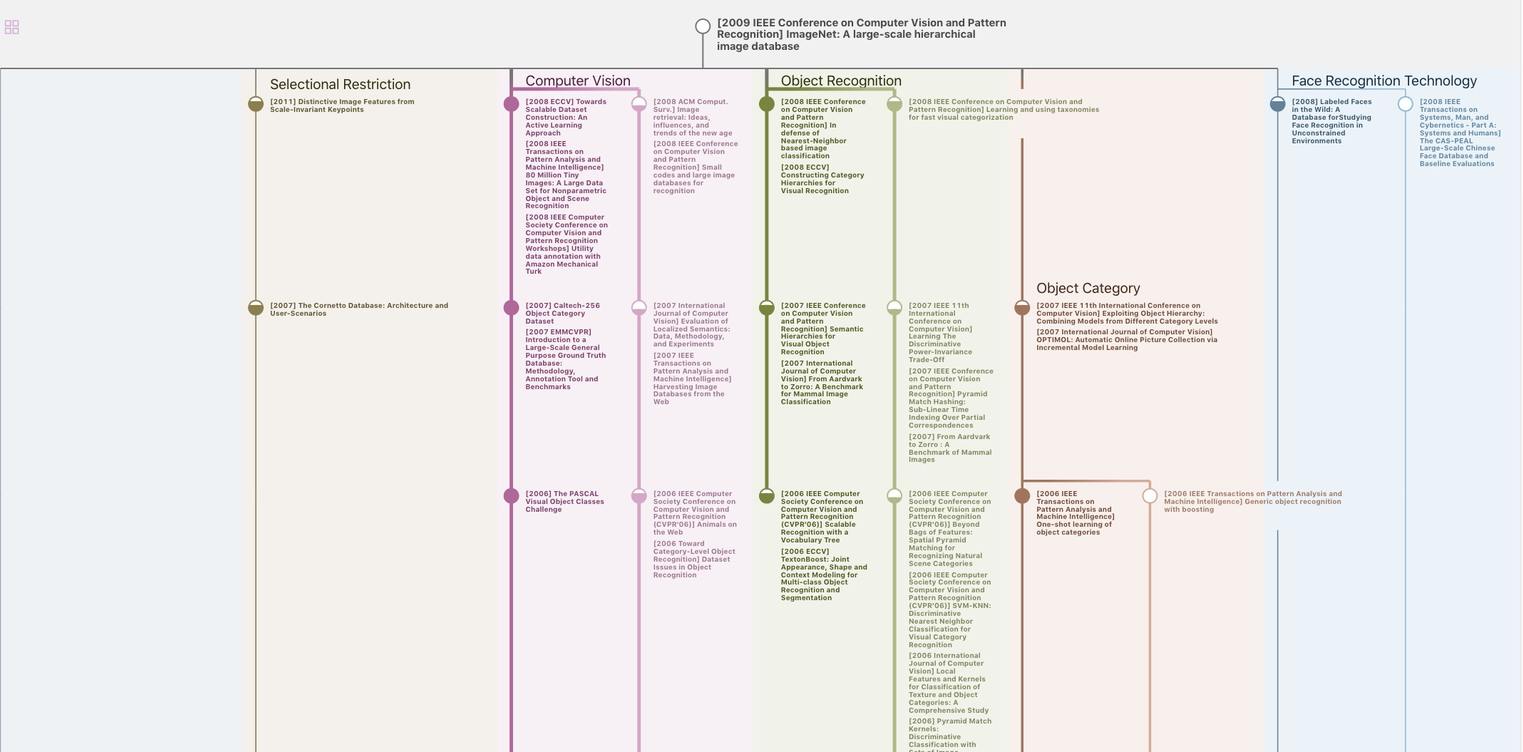
生成溯源树,研究论文发展脉络
Chat Paper
正在生成论文摘要