A Deep Deterministic Policy Gradient Approach to Medication Dosing and Surveillance in the ICU.
EMBC(2018)
摘要
Medication dosing in a critical care environment is a complex task that involves close monitoring of relevant physiologic and laboratory biomarkers and corresponding sequential adjustment of the prescribed dose. Misdosing of medications with narrow therapeutic windows (such as intravenous [IV] heparin) can result in preventable adverse events, decrease quality of care and increase cost. Therefore, a robust recommendation system can help clinicians by providing individualized dosing suggestions or corrections to existing protocols. We present a clinician-in-the-loop framework for adjusting IV heparin dose using deep reinforcement learning (RL). Our main objectives were to learn a new IV heparin dosing policy based on the multi-dimensional features of patients, and evaluate the effectiveness of the learned policy in the presence of other confounding factors that may contribute to heparin-related side effects. The data used in the experiments included 2598 intensive care patients from the publicly available MIMIC database and 2310 patients from the Emory University clinical data warehouse. Experimental results suggested that the distance from RL policy had a statistically significant association with anticoagulant complications $(p< 0.05)$, after adjusting for the effects of confounding factors.
更多查看译文
关键词
Anticoagulants,Critical Care,Heparin,Humans,Intensive Care Units,Reinforcement, Psychology
AI 理解论文
溯源树
样例
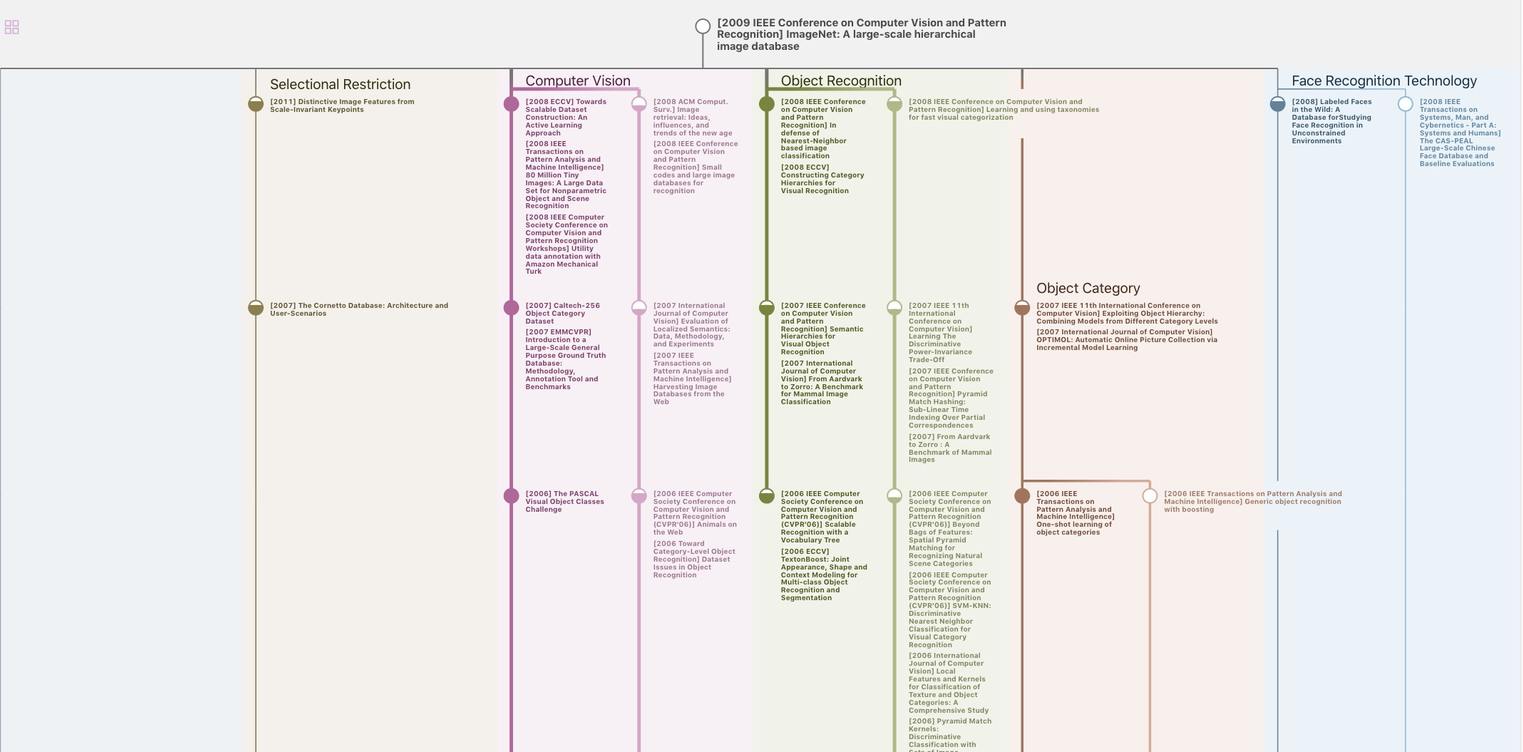
生成溯源树,研究论文发展脉络
Chat Paper
正在生成论文摘要