Varifocal-Net: A Chromosome Classification Approach Using Deep Convolutional Networks.
IEEE Transactions on Medical Imaging(2019)
摘要
Chromosome classification is critical for karyotyping in abnormality diagnosis. To expedite the diagnosis, we present a novel method named Varifocal-Net for simultaneous classification of chromosome's type and polarity using deep convolutional networks. The approach consists of one global-scale network (G-Net) and one local-scale network (L-Net). It follows three stages. The first stage is to learn both global and local features. We extract global features and detect finer local regions via the G-Net. By proposing a varifocal mechanism, we zoom into local parts and extract local features via the L-Net. Residual learning and multi-task learning strategies are utilized to promote high-level feature extraction. The detection of discriminative local parts is fulfilled by a localization subnet of the G-Net, whose training process involves both supervised and weakly supervised learning. The second stage is to build two multi-layer perceptron classifiers that exploit features of both two scales to boost classification performance. The third stage is to introduce a dispatch strategy of assigning each chromosome to a type within each patient case, by utilizing the domain knowledge of karyotyping. The evaluation results from 1909 karyotyping cases showed that the proposed Varifocal-Net achieved the highest accuracy per patient case (%) of 99.2 for both type and polarity tasks. It outperformed state-of-the-art methods, demonstrating the effectiveness of our varifocal mechanism, multi-scale feature ensemble, and dispatch strategy. The proposed method has been applied to assist practical karyotype diagnosis.
更多查看译文
关键词
Biological cells,Feature extraction,Microscopy,Task analysis,Support vector machines,Training,Indexes,Chromosome classification,varifocal mechanism,feature ensemble,convolutional networks,dispatch strategy
AI 理解论文
溯源树
样例
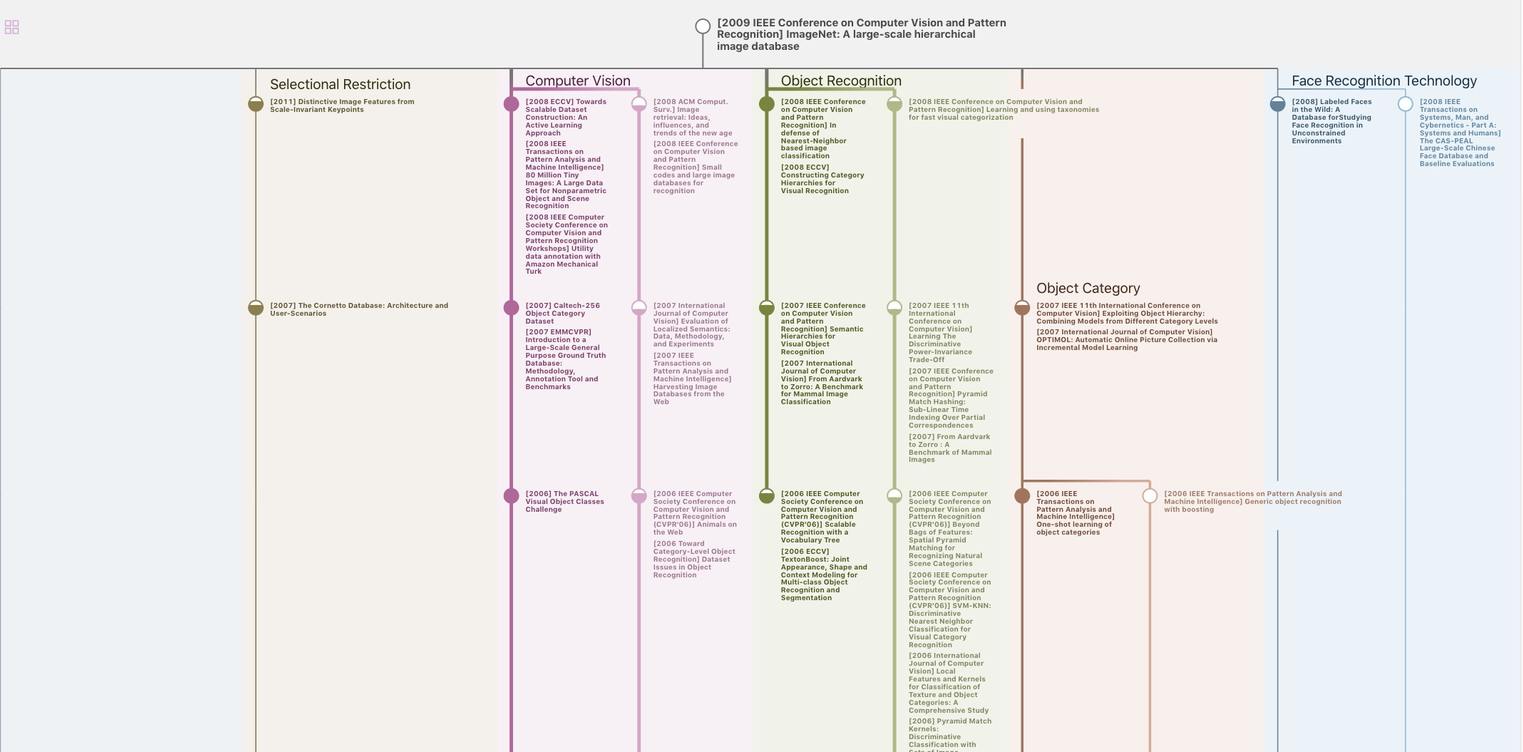
生成溯源树,研究论文发展脉络
Chat Paper
正在生成论文摘要