Multimodal Modeling of Coordination and Coregulation Patterns in Speech Rate during Triadic Collaborative Problem Solving.
ICMI(2018)
摘要
We model coordination and coregulation patterns in 33 triads engaged in collaboratively solving a challenging computer programming task for approximately 20 minutes. Our goal is to prospectively model speech rate (words/sec) - an important signal of turn taking and active participation - of one teammate (A or B or C) from time lagged nonverbal signals (speech rate and acoustic-prosodic features) of the other two (i.e., A + B → C; A + C → B; B + C → A) and task-related context features. We trained feed-forward neural networks (FFNNs) and long short-term memory recurrent neural networks (LSTMs) using group-level nested cross-validation. LSTMs outperformed FFNNs and a chance baseline and could predict speech rate up to 6s into the future. A multimodal combination of speech rate, acoustic-prosodic, and task context features outperformed unimodal and bimodal signals. The extent to which the models could predict an individual's speech rate was positively related to that individual's scores on a subsequent posttest, suggesting a link between coordination/coregulation and collaborative learning outcomes. We discuss applications of the models for real-time systems that monitor the collaborative process and intervene to promote positive collaborative outcomes.
更多查看译文
关键词
Collaborative problem solving, coordination, coregulation
AI 理解论文
溯源树
样例
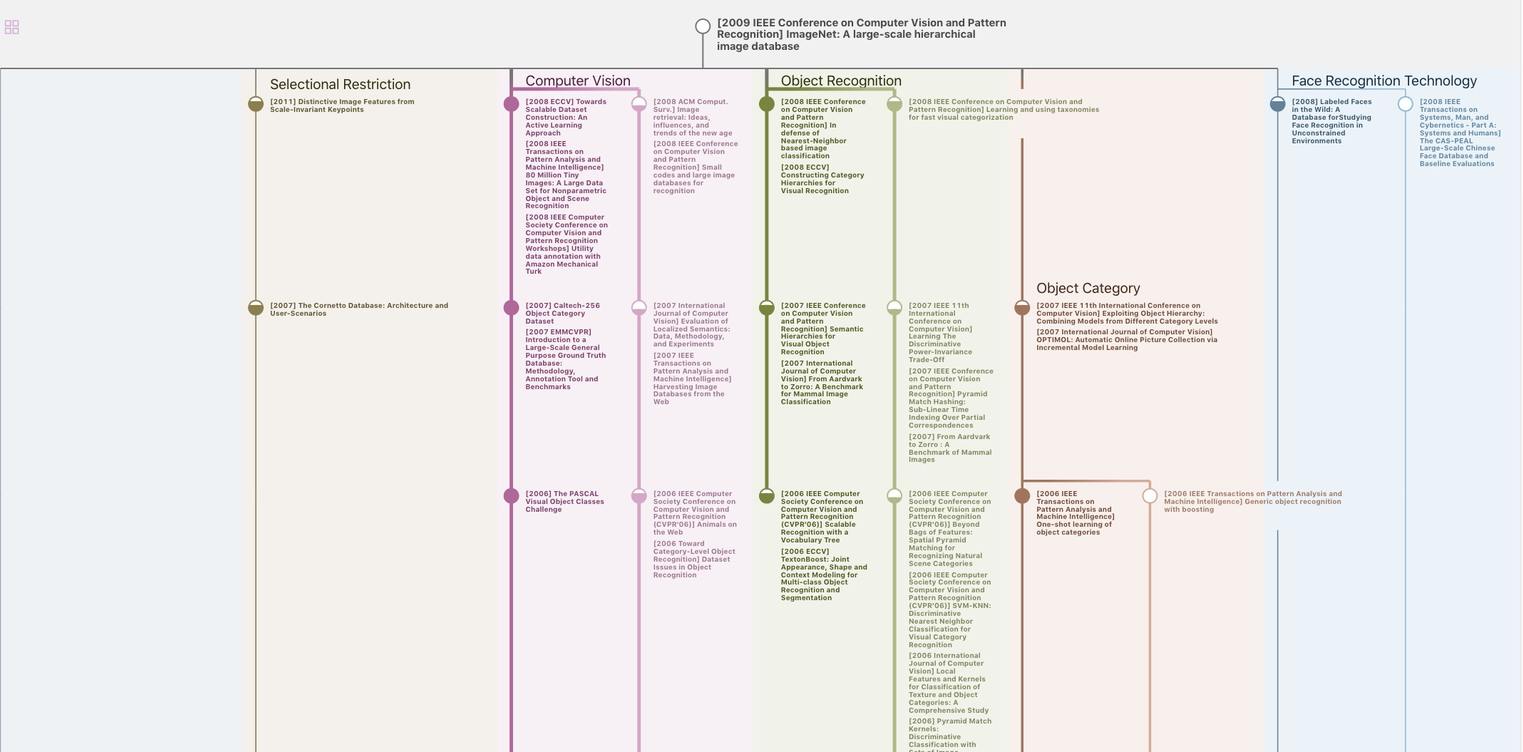
生成溯源树,研究论文发展脉络
Chat Paper
正在生成论文摘要