Joint Discrete and Continuous Emotion Prediction Using Ensemble and End-to-End Approaches.
ICMI(2018)
摘要
This paper presents a novel approach in continuous emotion prediction that characterizes dimensional emotion labels jointly with continuous and discretized representations. Continuous emotion labels can capture subtle emotion variations, but their inherent noise often has negative effects on model training. Recent approaches found a performance gain when converting the continuous labels into a discrete set (e.g., using k-means clustering), despite a label quantization error. To find the optimal trade-off between the continuous and discretized emotion representations, we investigate two joint modeling approaches: ensemble and end-to-end. The ensemble model combines the predictions from two models that are trained separately, one with discretized prediction and the other with continuous prediction. On the other hand, the end-to-end model is trained to simultaneously optimize both discretized and continuous prediction tasks in addition to the final combination between them. Our experimental results using the state-of-the-art deep BLSTM network on the RECOLA dataset demonstrate that (i) the joint representation outperforms both individual representation baselines and the state-of-the-art speech based results on RECOLA, validating the assumption that combining continuous and discretized emotion representations yields better performance in emotion prediction; and (ii) the joint representation can help to accelerate convergence, particularly for valence prediction. Our work provides insights into joint discrete and continuous emotion representation and its efficacy for describing dynamically changing affective behavior in valence and activation prediction.
更多查看译文
关键词
Emotion recognition, Continuous emotion prediction, Joint representation, Bidirectional long-short-term memory
AI 理解论文
溯源树
样例
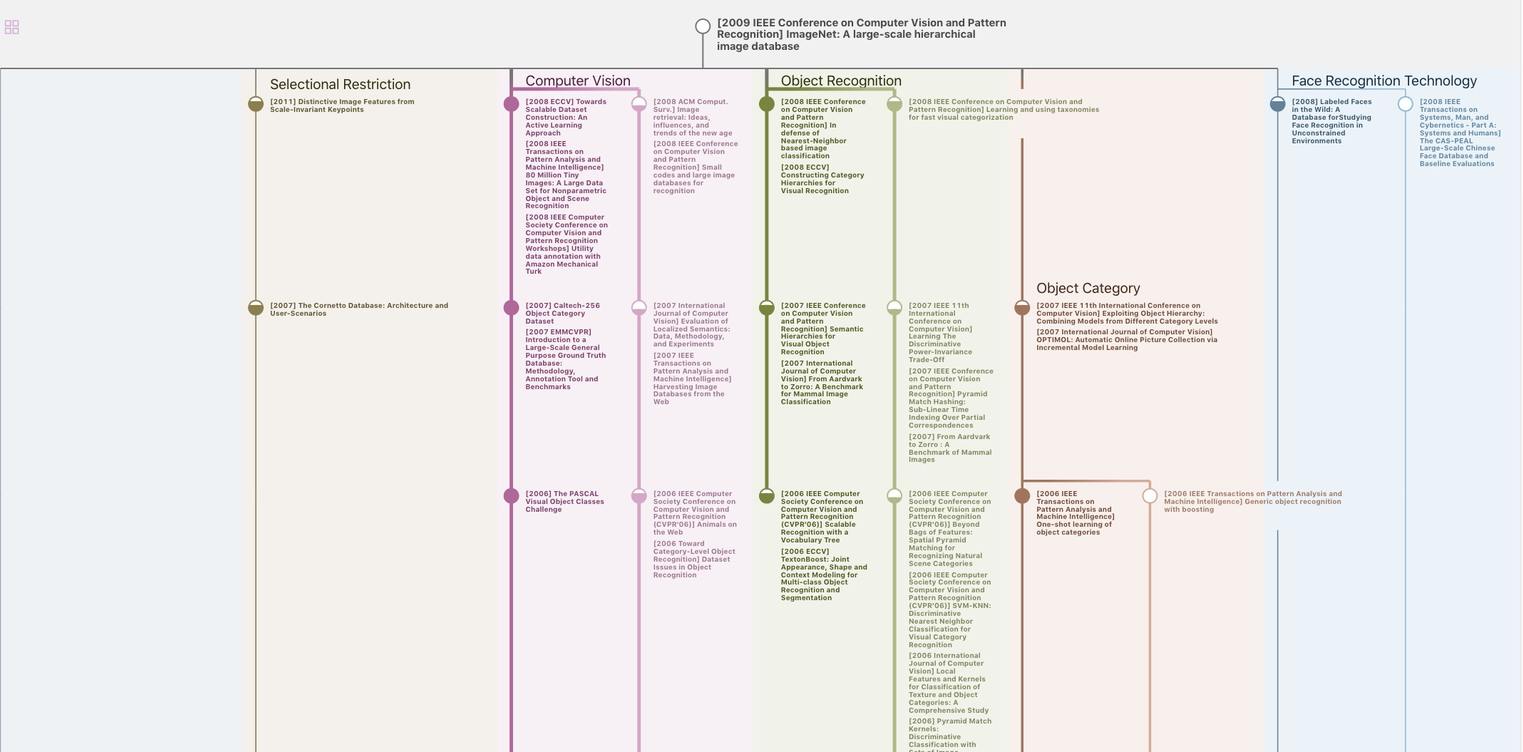
生成溯源树,研究论文发展脉络
Chat Paper
正在生成论文摘要