Add: Actionness-Pooled Deep-Convolutional Descriptor
2018 IEEE International Conference on Multimedia and Expo (ICME)(2018)
摘要
Recognition of general actions has achieved great breakthroughs in recent years. However, in real-world applications, finer-grained action classification is often needed. The major challenge is that fine-grained actions usually share high similarities in both appearance and motion pattern, making it difficult to distinguish them with existing general action representation. To solve this problem, we introduce visual attention mechanism into the proposed descriptor, termed as Actionness-pooled Deep-convolutional Descriptor (ADD). Instead of pooling features uniformly from the entire video, we aggregate features in sub-regions that are more likely to contain actions according to actionness maps, which endow ADD with the capability of capturing the subtle differences between fine-grained actions. We conduct experiments on HIT Dances dataset, one of the few existing datasets for fine-grained action analysis. Quantitative results have demonstrated that ADD remarkably outperforms traditional two-stream representation. Extensive experiments on two general action benchmarks, JHMDB and UCF101, have additionally proved that combining ADD with end-to-end ConvNet can further boost the recognition performance.
更多查看译文
关键词
ADD,deep-convolutional descriptor,actionness estimation,action recognition
AI 理解论文
溯源树
样例
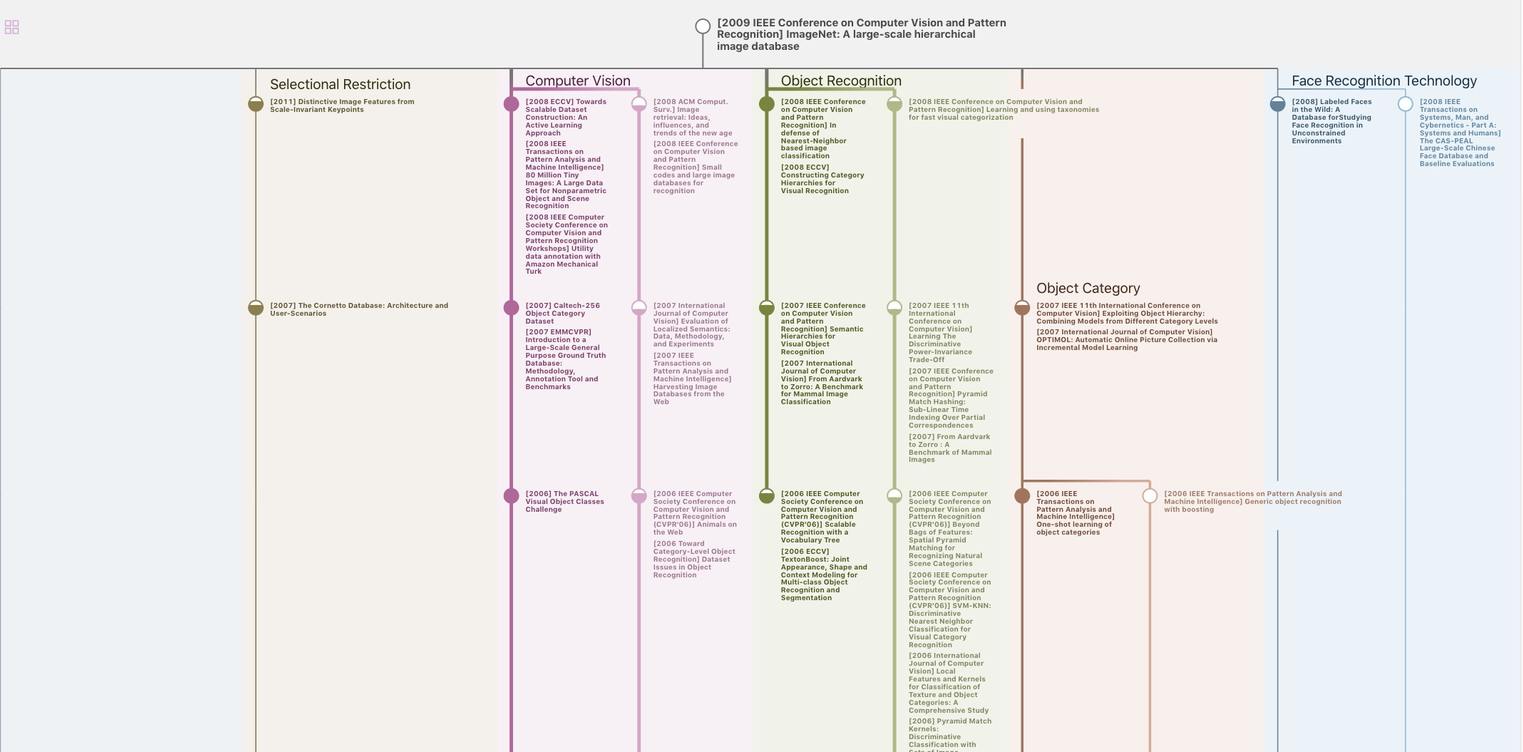
生成溯源树,研究论文发展脉络
Chat Paper
正在生成论文摘要