Cooperative photometric redshift estimation.
IAU Symposium Proceedings Series(2017)
摘要
In the modern galaxy surveys photometric redshifts play a central role in a broad range of studies, from gravitational lensing and dark matter distribution to galaxy evolution. Using a dataset of similar to 25, 000 galaxies from the second data release of the Kilo Degree Survey (KiDS) we obtain photometric redshifts with five different methods: (i) Random forest, (ii) Multi Layer Perceptron with Quasi Newton Algorithm, (iii) Multi Layer Perceptron with an optimization network based on the Levenberg-Marquardt learning rule, (iv) the Bayesian Photometric Redshift model (or BPZ) and (v) a classical SED template fitting procedure (Le Phare). We show how SED fitting techniques could provide useful information on the galaxy spectral type which can be used to improve the capability of machine learning methods constraining systematic errors and reduce the occurrence of catastrophic outliers. We use such classification to train specialized regression estimators, by demonstrating that such hybrid approach, involving SED fitting and machine learning in a single collaborative framework, is capable to improve the overall prediction accuracy of photometric redshifts.
更多查看译文
关键词
methods: data analysis,methods: statistical,catalogs
AI 理解论文
溯源树
样例
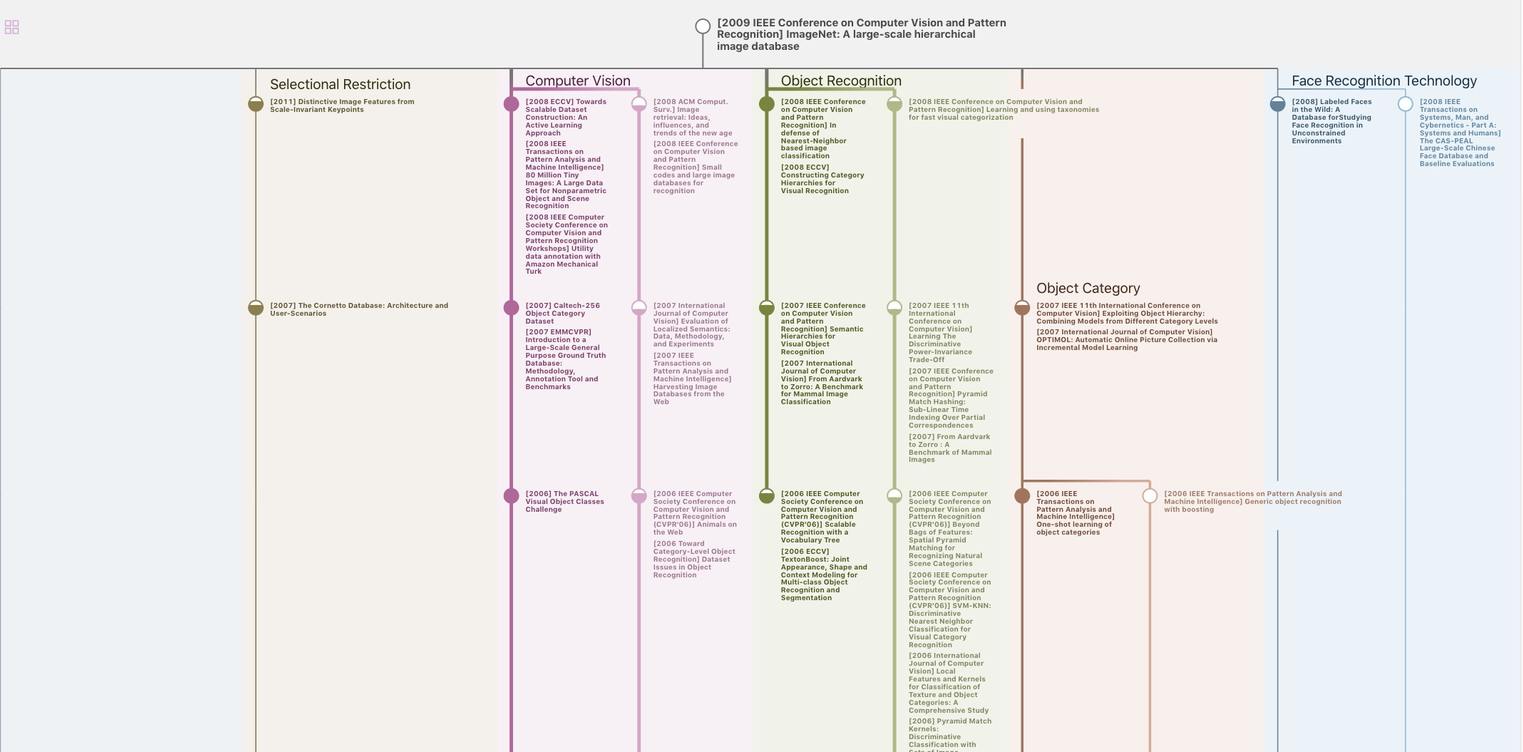
生成溯源树,研究论文发展脉络
Chat Paper
正在生成论文摘要