Privacy-Aware Kalman Filtering
2018 IEEE INTERNATIONAL CONFERENCE ON ACOUSTICS, SPEECH AND SIGNAL PROCESSING (ICASSP)(2018)
摘要
We are concerned with a privacy-preserving problem in Kalman filter: a sensor releases a set of measurements to fusion center, who has perfect knowledge of the dynamical model, to allow it to estimate the public state, while prevent it from estimating the private state. We propose to linearly transform the original observation into a lower dimensional space before sending them to fusion center. Two privacy-utility tradeoffs are formulated: one concerns only at the current time step and the other concerns over two time steps. The transformation that leads to the optimal tradeoff can be found in closed-form. The privacy (estimation of private state) and utility (estimation of public state) are measured based on recursive Bayesian Cramer-Rao bound.
更多查看译文
关键词
Kalman filter, inference privacy, compression, linear transformation, parameter estimation, recursive Bayesian Cramer-Rao bound
AI 理解论文
溯源树
样例
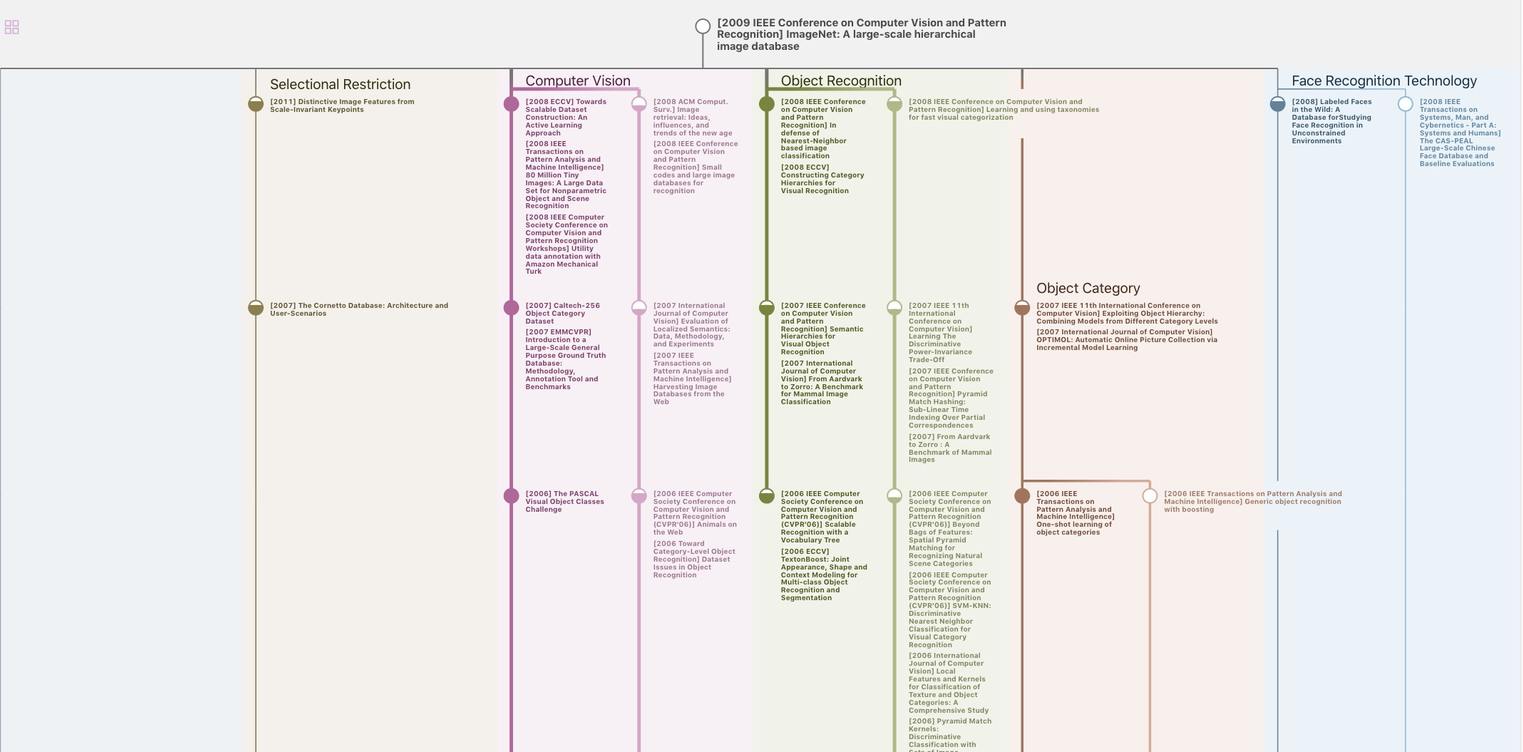
生成溯源树,研究论文发展脉络
Chat Paper
正在生成论文摘要