Linear regression for uplift modeling
Data Min. Knowl. Discov.(2018)
摘要
The purpose of statistical modeling is to select targets for some action, such as a medical treatment or a marketing campaign. Unfortunately, classical machine learning algorithms are not well suited to this task since they predict the results after the action, and not its causal impact. The answer to this problem is uplift modeling, which, in addition to the usual training set containing objects on which the action was taken, uses an additional control group of objects not subjected to it. The predicted true effect of the action on a given individual is modeled as the difference between responses in both groups. This paper analyzes two uplift modeling approaches to linear regression, one based on the use of two separate models and the other based on target variable transformation. Adapting the second estimator to the problem of regression is one of the contributions of the paper. We identify the situations when each model performs best and, contrary to several claims in the literature, show that the double model approach has favorable theoretical properties and often performs well in practice. Finally, based on our analysis we propose a third model which combines the benefits of both approaches and seems to be the model of choice for uplift linear regression. Experimental analysis confirms our theoretical results on both simulated and real data, clearly demonstrating good performance of the double model and the advantages of the proposed approach.
更多查看译文
关键词
Uplift modeling,Linear regression,Causal discovery
AI 理解论文
溯源树
样例
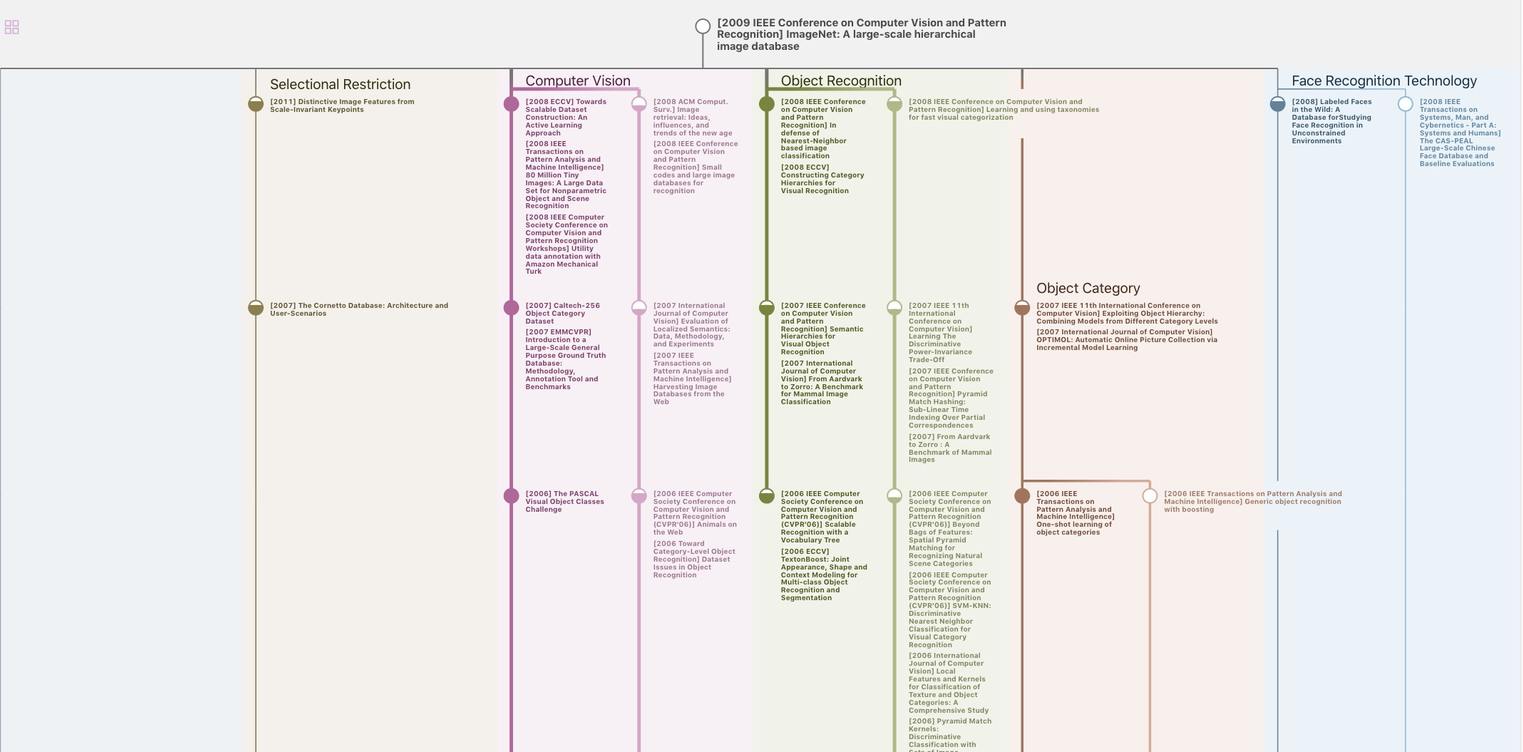
生成溯源树,研究论文发展脉络
Chat Paper
正在生成论文摘要