Paying Attention to Attention: Highlighting Influential Samples in Sequential Analysis.
arXiv: Learning(2018)
摘要
In (Yang et al. 2016), a hierarchical attention network (HAN) is created for document classification. The attention layer can be used to visualize text influential in classifying the document, thereby explaining the modelu0027s prediction. We successfully applied HAN to a sequential analysis task in the form of real-time monitoring of turn taking in conversations. However, we discovered instances where the attention weights were uniform at the stopping point (indicating all turns were equivalently influential to the classifier), preventing meaningful visualization for real-time human review or classifier improvement. We observed that attention weights for turns fluctuated as the conversations progressed, indicating turns had varying influence based on conversation state. Leveraging this observation, we develop a method to create more informative real-time visuals (as confirmed by human reviewers) in cases of uniform attention weights using the changes in turn importance as a conversation progresses over time.
更多查看译文
AI 理解论文
溯源树
样例
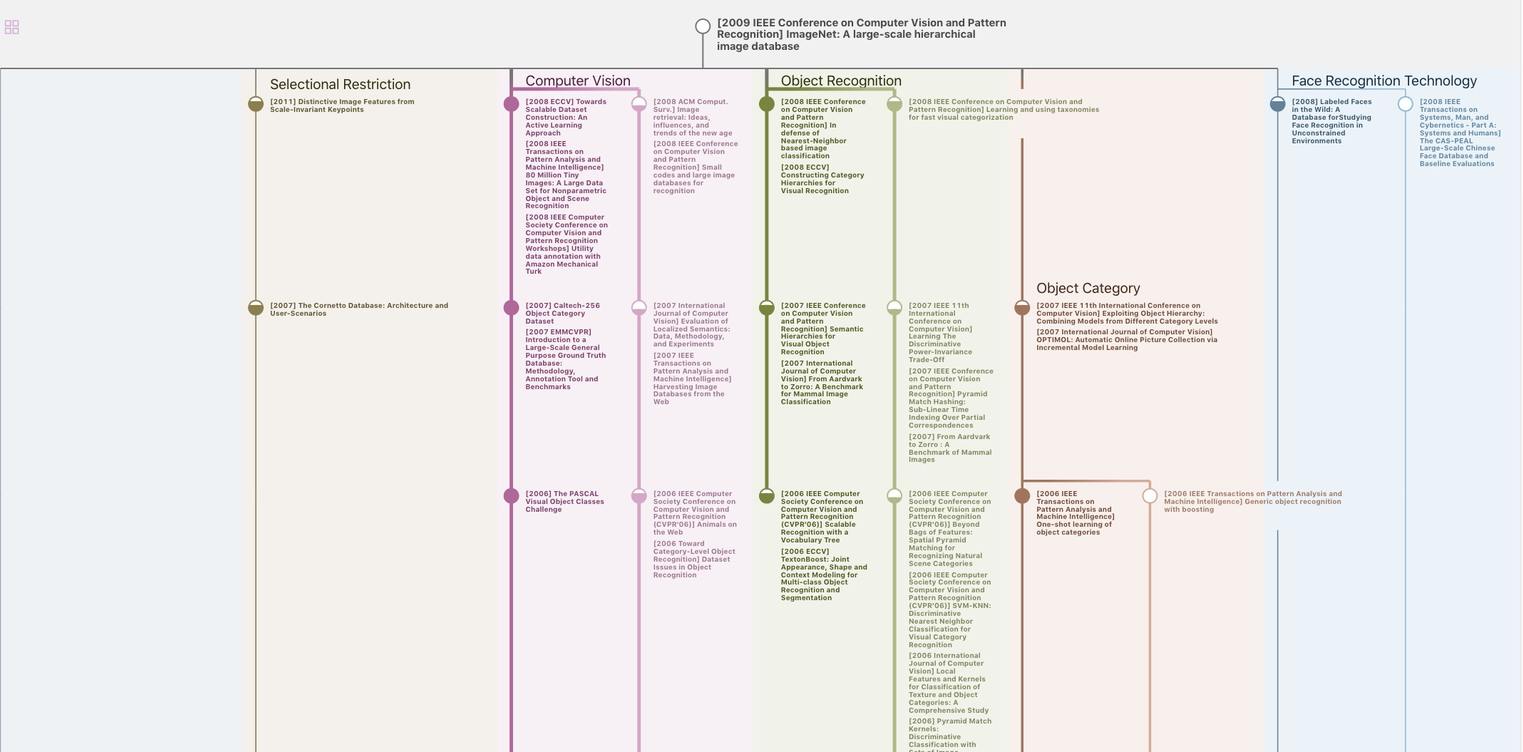
生成溯源树,研究论文发展脉络
Chat Paper
正在生成论文摘要