Video Jigsaw: Unsupervised Learning of Spatiotemporal Context for Video Action Recognition
2019 IEEE Winter Conference on Applications of Computer Vision (WACV)(2018)
摘要
We propose a self-supervised learning method to jointly reason about spatial and temporal context for video recognition. Recent self-supervised approaches have used spatial context [9, 34] as well as temporal coherency [32] but a combination of the two requires extensive preprocessing such as tracking objects through millions of video frames [59] or computing optical flow to determine frame regions with high motion [30]. We propose to combine spatial and temporal context in one self-supervised framework without any heavy preprocessing. We divide multiple video frames into grids of patches and train a network to solve jigsaw puzzles on these patches from multiple frames. So the network is trained to correctly identify the position of a patch within a video frame as well as the position of a patch over time. We also propose a novel permutation strategy that outperforms random permutations while significantly reducing computational and memory constraints. We use our trained network for transfer learning tasks such as video activity recognition and demonstrate the strength of our approach on two benchmark video action recognition datasets without using a single frame from these datasets for unsupervised pretraining of our proposed video jigsaw network.
更多查看译文
关键词
Task analysis,Training,Optical imaging,Visualization,Tracking,Unsupervised learning,Spatiotemporal phenomena
AI 理解论文
溯源树
样例
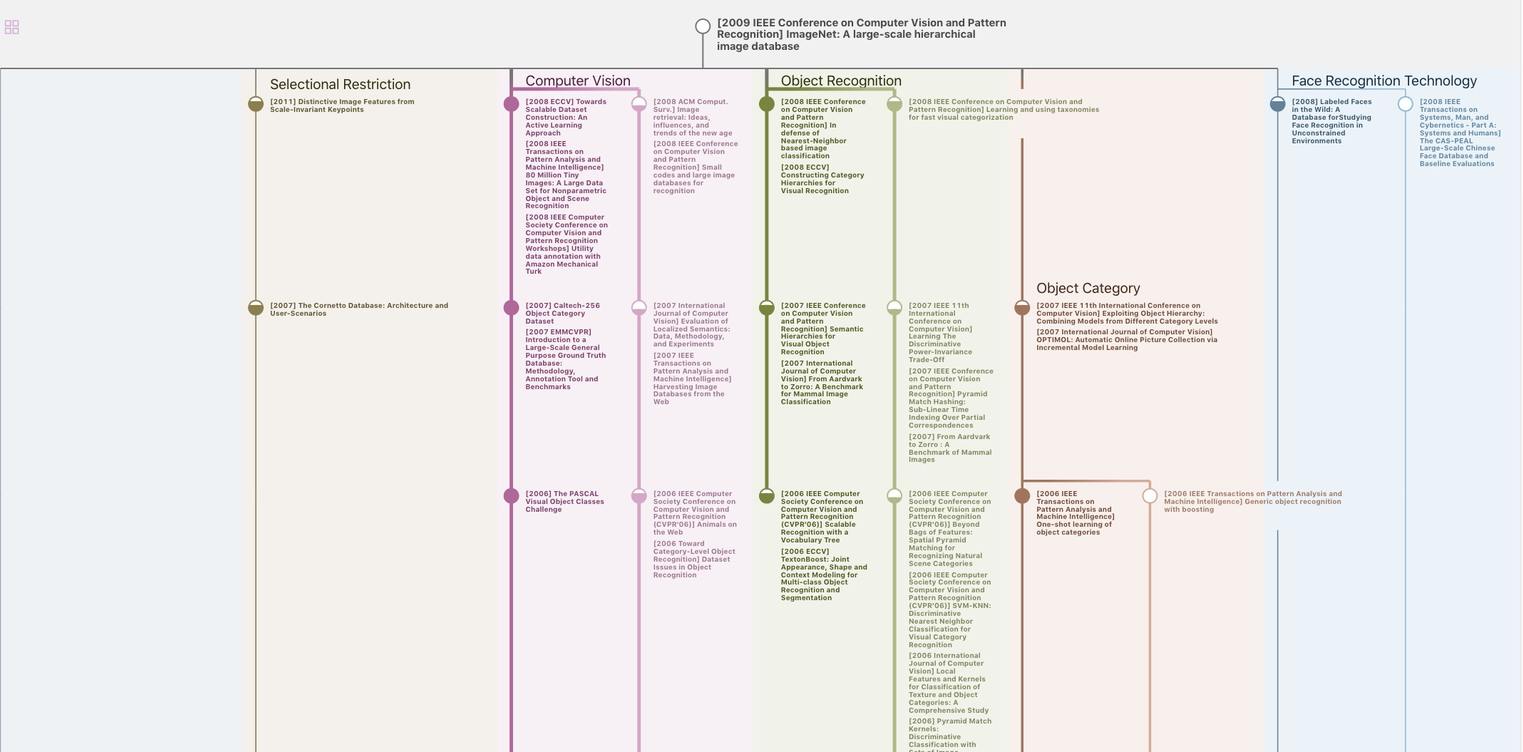
生成溯源树,研究论文发展脉络
Chat Paper
正在生成论文摘要