CReaM: Condensed Real-time Models for Depth Prediction using Convolutional Neural Networks
2018 IEEE/RSJ International Conference on Intelligent Robots and Systems (IROS)(2018)
摘要
Since the resurgence of CNNs the robotic vision community has developed a range of algorithms that perform classification, semantic segmentation and structure prediction (depths, normals, surface curvature) using neural networks. While some of these models achieve state-of-the art results and super human level performance, deploying these models in a time critical robotic environment remains an ongoing challenge. Real-time frameworks are of paramount importance to build a robotic society where humans and robots integrate seamlessly. To this end, we present a novel real-time structure prediction framework that predicts depth at 30 frames per second on an NVIDIA-TX2. At the time of writing, this is the first piece of work to showcase such a capability on a mobile platform. We also demonstrate with extensive experiments that neural networks with very large model capacities can be leveraged in order to train accurate condensed model architectures in a “from teacher to student” style knowledge transfer.
更多查看译文
关键词
CNNs,robotic vision community,semantic segmentation,surface curvature,robotic society,real-time structure prediction framework,NVIDIA-TX2,CReaM,real-time models,depth prediction,convolutional neural networks,classification,mobile platform,condensed model architectures
AI 理解论文
溯源树
样例
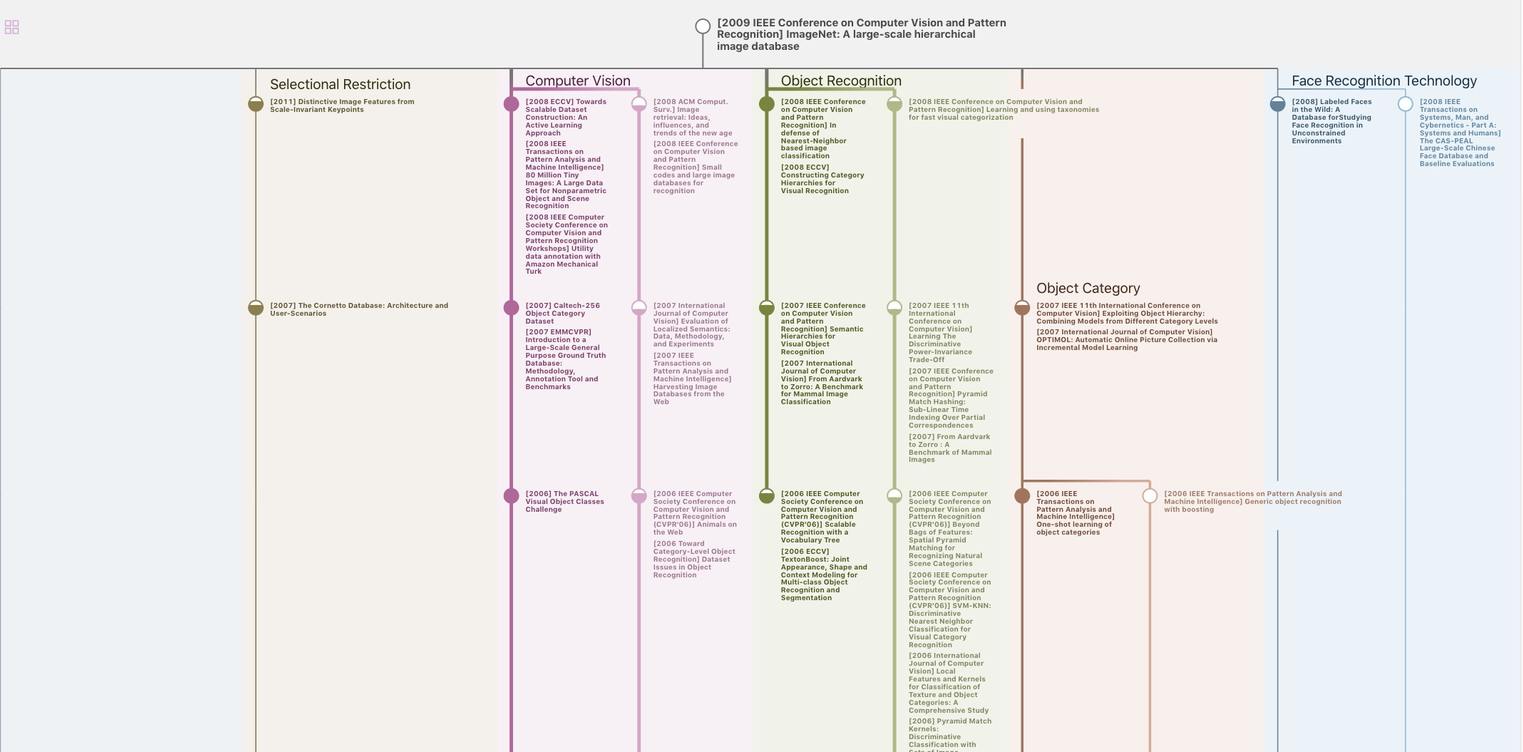
生成溯源树,研究论文发展脉络
Chat Paper
正在生成论文摘要