How transferable are the datasets collected by active learners?
arXiv: Learning(2018)
摘要
Active learning is a widely-used training strategy for maximizing predictive performance subject to a fixed annotation budget. Between rounds of training, an active learner iteratively selects examples for annotation, typically based on some measure of the modelu0027s uncertainty, coupling the acquired dataset with the underlying model. However, owing to the high cost of annotation and the rapid pace of model development, labeled datasets may remain valuable long after a particular model is surpassed by new technology. In this paper, we investigate the transferability of datasets collected with an acquisition model A to a distinct successor model S. We seek to characterize whether the benefits of active learning persist when A and S are different models. To this end, we consider two standard NLP tasks and associated datasets: text classification and sequence tagging. We find that training S on a dataset actively acquired with a (different) model A typically yields worse performance than when S is trained with native data (i.e., acquired actively using S), and often performs worse than training on i.i.d. sampled data. These findings have implications for the use of active learning in practice,suggesting that it is better suited to cases where models are updated no more frequently than labeled data.
更多查看译文
AI 理解论文
溯源树
样例
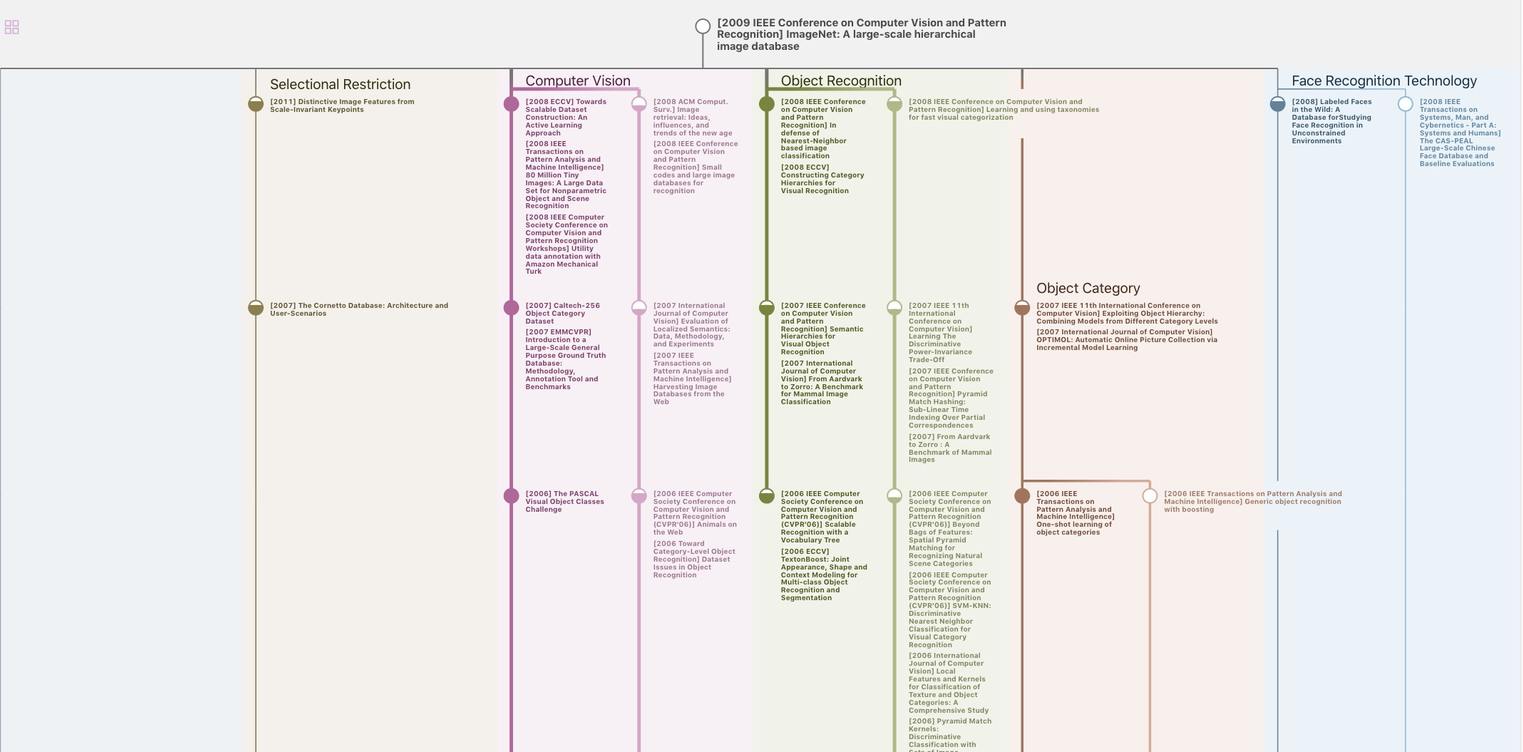
生成溯源树,研究论文发展脉络
Chat Paper
正在生成论文摘要