Structvae: Tree-Structured Latent Variable Models For Semi-Supervised Semantic Parsing
ACL (1)(2018)
摘要
Semantic parsing is the task of transducing natural language (NL) utterances into formal meaning representations (MRs), commonly represented as tree structures. Annotating NL utterances with their corresponding MRs is expensive and time-consuming, and thus the limited availability of labeled data often becomes the bottle-neck of data-driven, supervised models. We introduce STRUCTVAE, a variational auto-encoding model for semi-supervised semantic parsing, which learns both from limited amounts of parallel data, and readily-available unlabeled NL utterances. STRUCTVAE models latent MRs not observed in the unlabeled data as tree-structured latent variables. Experiments on semantic parsing on the ATIS domain and Python code generation show that with extra unlabeled data, STRUCTVAE outperforms strong supervised models.(1)
更多查看译文
AI 理解论文
溯源树
样例
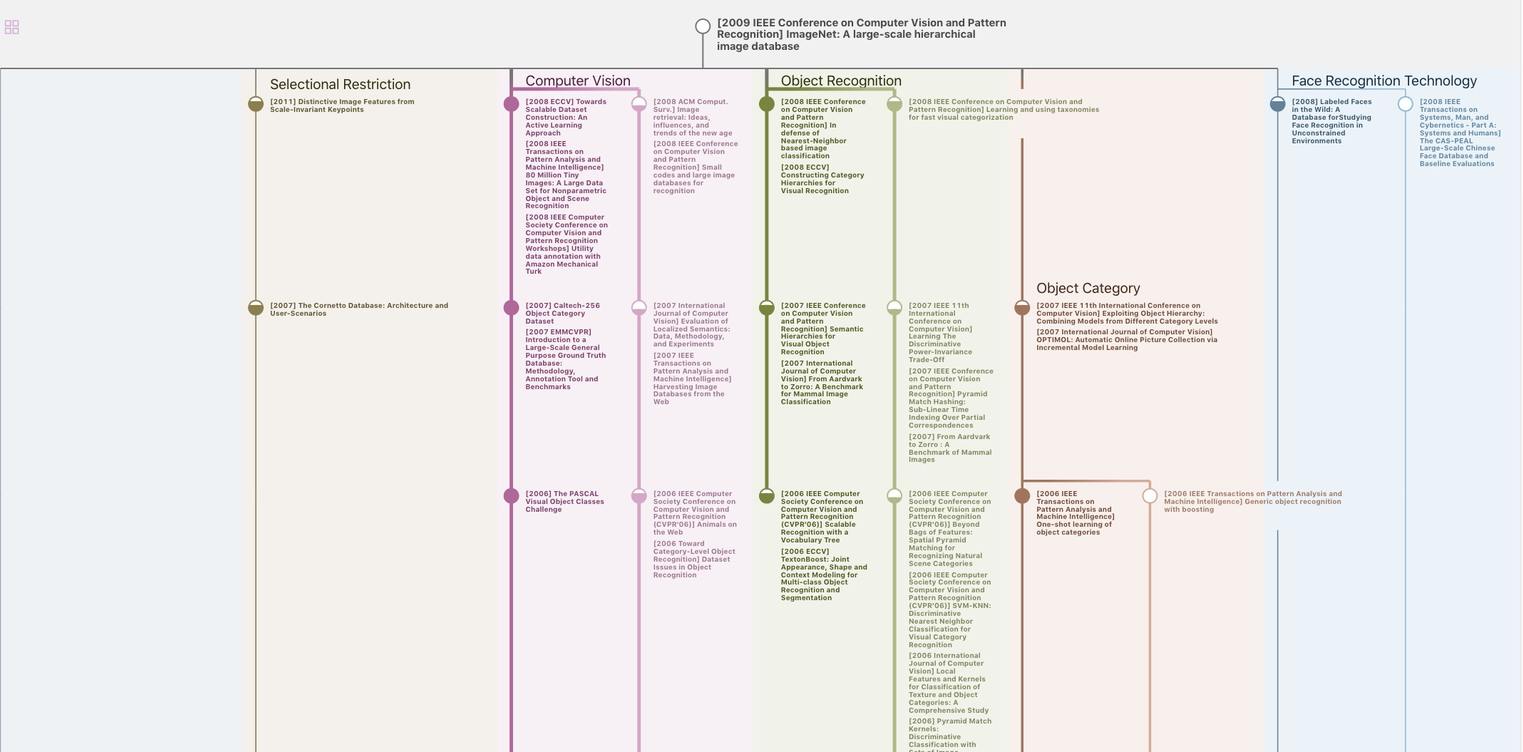
生成溯源树,研究论文发展脉络
Chat Paper
正在生成论文摘要