RDF shape induction using knowledge base profiling.
SAC 2018: Symposium on Applied Computing Pau France April, 2018(2018)
摘要
Knowledge Graphs (KGs) are becoming the core of most artificial intelligent and cognitive applications. Popular KGs such as DBpedia and Wikidata have chosen the RDF data model to represent their data. Despite the advantages, there are challenges in using RDF data, for example, data validation. Ontologies for specifying domain conceptualizations in RDF data are designed for entailments rather than validation. Most ontologies lack the granular information needed for validating constraints. Recent work on RDF Shapes and standardization of languages such as SHACL and ShEX provide better mechanisms for representing integrity constraints for RDF data. However, manually creating constraints for large KGs is still a tedious task. In this paper, we present a data driven approach for inducing integrity constraints for RDF data using data profiling. Those constraints can be combined into RDF Shapes and can be used to validate RDF graphs. Our method is based on machine learning techniques to automatically generate RDF shapes using profiled RDF data as features. In the experiments, the proposed approach achieved 97% precision in deriving RDF Shapes with cardinality constraints for a subset of DBpedia data.
更多查看译文
关键词
RDF Shape, Knowledge Base, Data Quality, Machine Learning
AI 理解论文
溯源树
样例
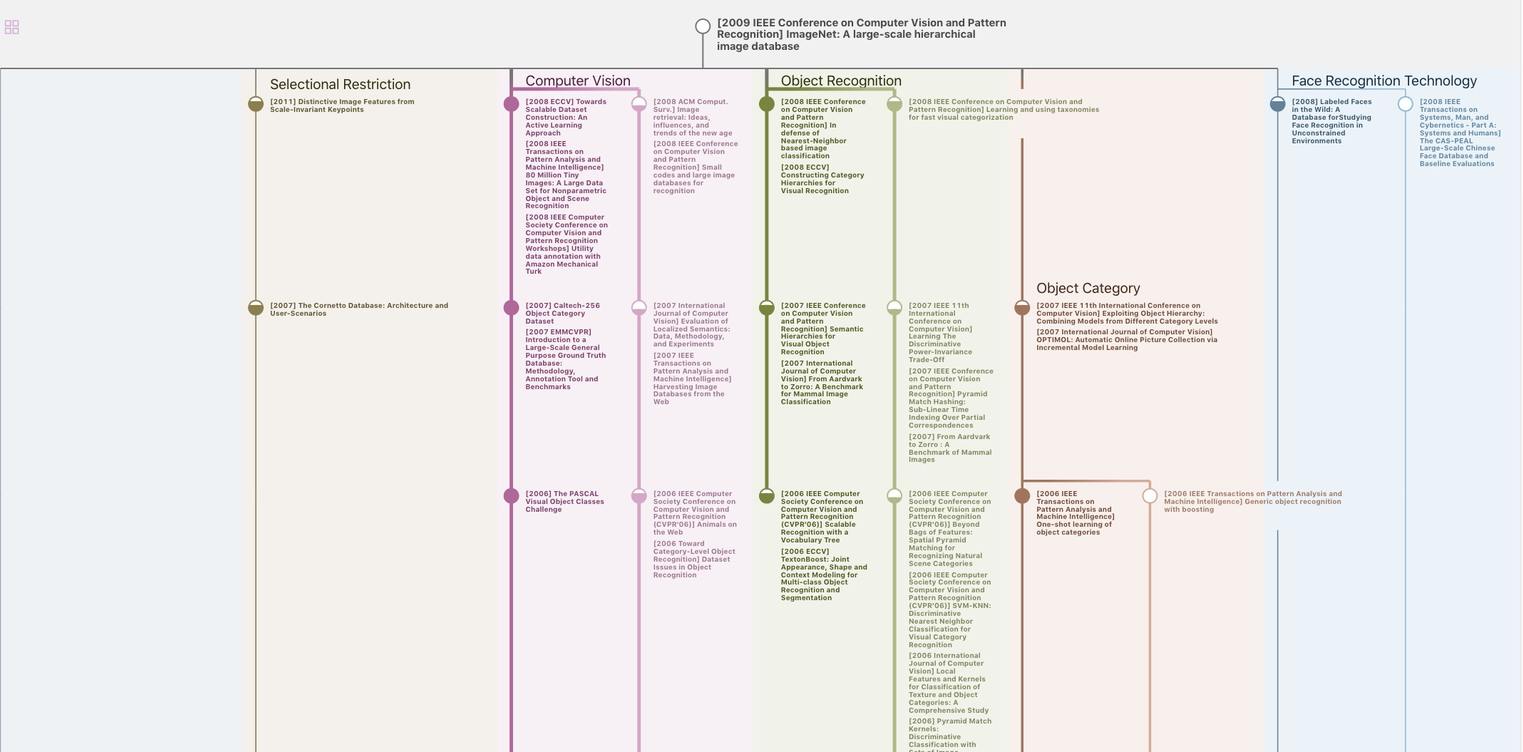
生成溯源树,研究论文发展脉络
Chat Paper
正在生成论文摘要