Improving 2D Face Recognition via Discriminative Face Depth Estimation
2018 International Conference on Biometrics (ICB)(2018)
摘要
As face recognition progresses from constrained scenarios to unconstrained scenarios, new challenges such as large pose, bad illumination, and partial occlusion, are encountered. While 3D or multi-modality RGB-D sensors are helpful for face recognition systems to achieve robustness against these challenges, the requirement of new sensors limits their application scenarios. In our paper, we propose a discriminative face depth estimation approach to improve 2D face recognition accuracies under unconstrained scenarios. Our discriminative depth estimation method uses a cascaded FCN and CNN architecture, in which FCN aims at recovering the depth from an RGB image, and CNN retains the separability of individual subjects. The estimated depth information is then used as a complementary modality to RGB for face recognition tasks. Experiments on two public datasets and a dataset we collect show that the proposed face recognition method using RGB and estimated depth information can achieve better accuracy than using RGB modality alone.
更多查看译文
关键词
face depth estimation,RGB D face recognition,Subject discriminability,Cascaded network
AI 理解论文
溯源树
样例
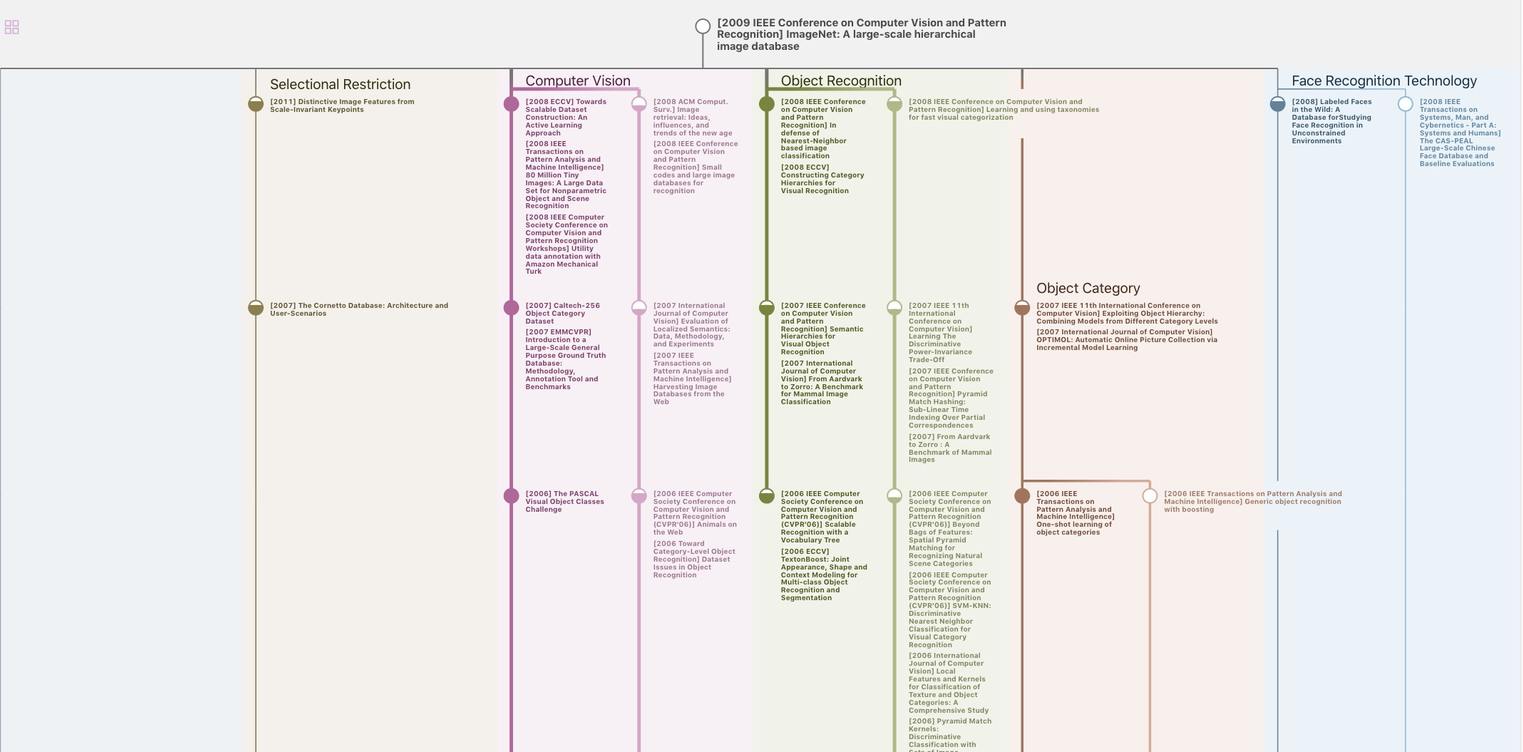
生成溯源树,研究论文发展脉络
Chat Paper
正在生成论文摘要