Two-Stream Part-Based Deep Representation for Human Attribute Recognition
2018 International Conference on Biometrics (ICB)(2018)
摘要
Recognizing human attributes in unconstrained environments is a challenging computer vision problem. State-of-the-art approaches to human attribute recognition are based on convolutional neural networks (CNNs). The de facto practice when training these CNNs on a large labeled image dataset is to take RGB pixel values of an image as input to the network. In this work, we propose a two-stream part-based deep representation for human attribute classification. Besides the standard RGB stream, we train a deep network by using mapped coded images with explicit texture information, that complements the standard RGB deep model. To integrate human body parts knowledge, we employ the deformable part-based models together with our two-stream deep model. Experiments are performed on the challenging Human Attributes (HAT-27) Dataset consisting of 27 different human attributes. Our results clearly show that (a) the two-stream deep network provides consistent gain in performance over the standard RGB model and (b) that the attribute classification results are further improved with our two-stream part-based deep representations, leading to state-of-the-art results.
更多查看译文
关键词
Deep Learning,Human attribute Recognition,Part-based representation
AI 理解论文
溯源树
样例
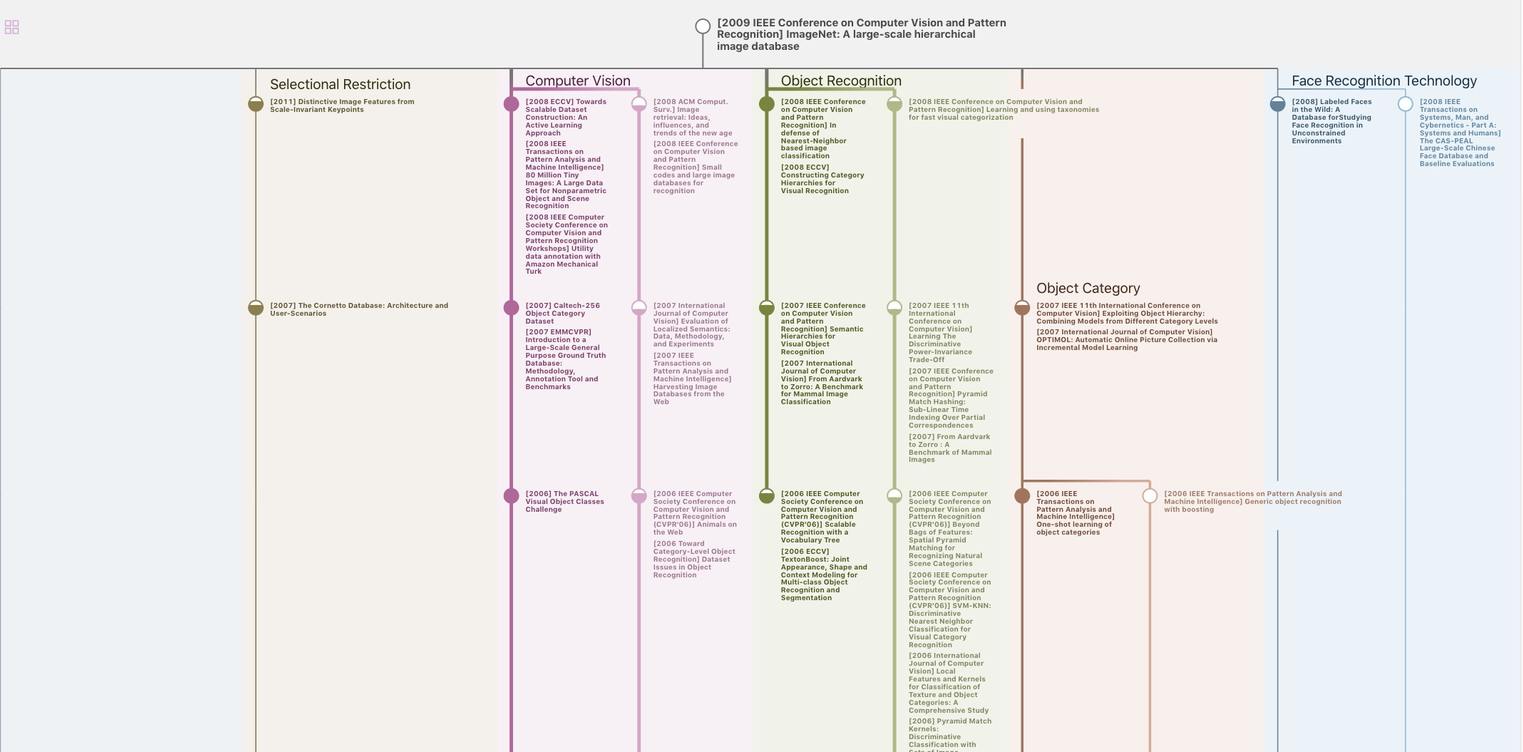
生成溯源树,研究论文发展脉络
Chat Paper
正在生成论文摘要