Sentence Modeling Via Multiple Word Embeddings And Multi-Level Comparison For Semantic Textual Similarity
Information Processing and Management: an International Journal(2019)
摘要
Recently, using a pretrained word embedding to represent words achieves success in many natural language processing tasks. According to objective functions, different word embedding models capture different aspects of linguistic properties. However, the Semantic Textual Similarity task, which evaluates similarity/relation between two sentences, requires to take into account of these linguistic aspects. Therefore, this research aims to encode various characteristics from multiple sets of word embeddings into one embedding and then learn similarity/relation between sentences via this novel embedding. Representing each word by multiple word embeddings, the proposed MaxLSTM-CNN encoder generates a novel sentence embedding. We then learn the similarity/relation between our sentence embeddings via Multi-level comparison. Our method M-MaxLSTM-CNN consistently shows strong performances in several tasks (i.e., measure textual similarity, identify paraphrase, recognize textual entailment). Our model does not use hand-crafted features (e.g., alignment features, Ngram overlaps, dependency features) as well as does not require pre-trained word embeddings to have the same dimension.
更多查看译文
关键词
Multiple word embeddings,Sentence embedding,Semantic,Similarity,Multi-level comparison
AI 理解论文
溯源树
样例
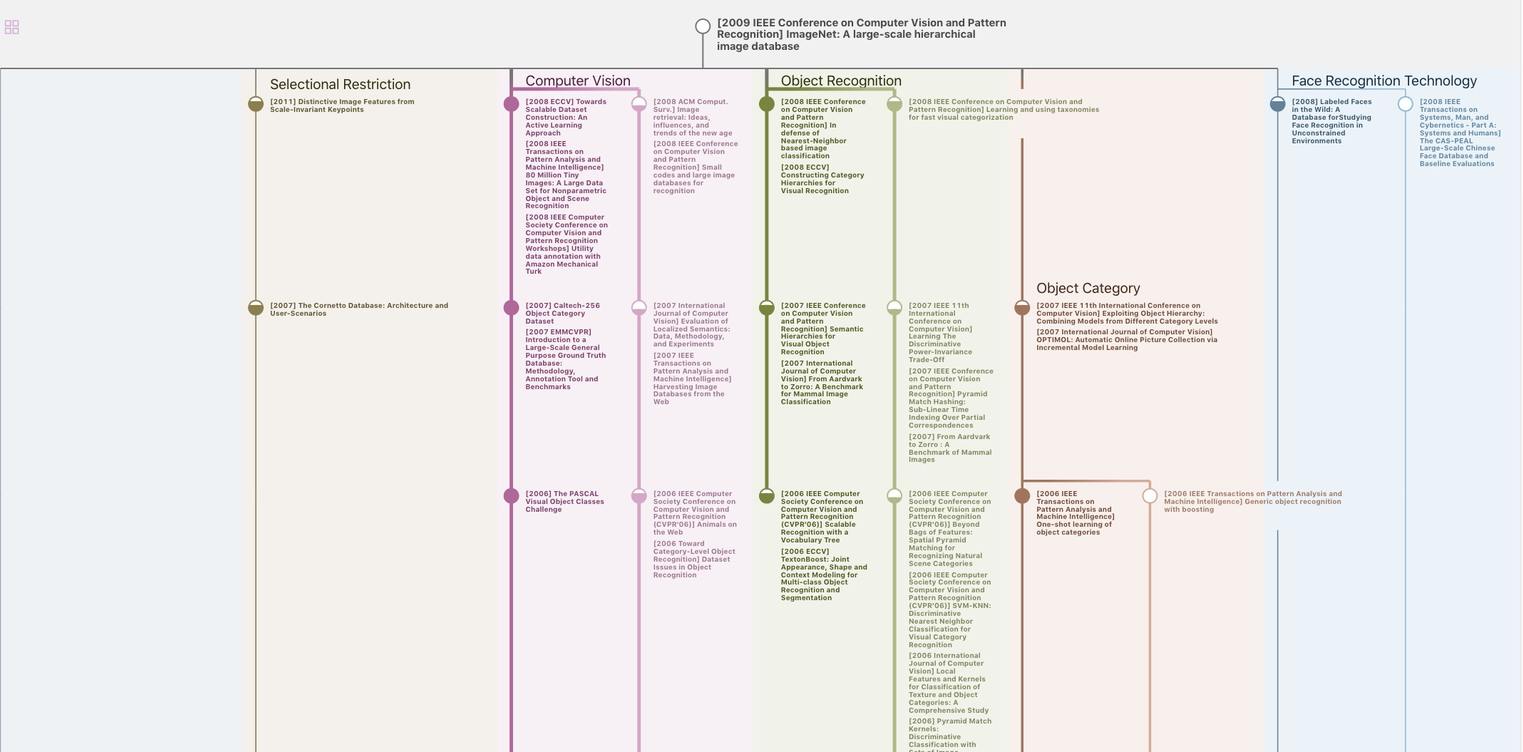
生成溯源树,研究论文发展脉络
Chat Paper
正在生成论文摘要