Skyblocking: Learning Blocking Schemes on the Skyline.
arXiv: Databases(2018)
摘要
In this paper, for the first time, we introduce the concept of skyblocking, which aims to learn scheme skylines. Given a set of blocking schemes and measures (e.g. PC and PQ), each scheme can be mapped as a point to a scheme space where each measure is one dimension. The scheme skyline points are not dominated by any other scheme point in the scheme space considering their measure values. The main difference with traditional skyline queries is that the measure values associated with the blocking schemes are not given, but have to be calculated based on given datasets. Moreover, existing work to calculate such values have the limitations that either a large amount of labels are required or the accurate measure values are hard to be guaranteed. The main purpose and novelty behind our approach is that we only need to use a limited number of labels to efficiently learn a set of blocking schemes (i.e. scheme skyline) such that no scheme points are dominated by others in the scheme space. The experimental results show that our approach has good performance on label efficiency, blocking quality and blocking efficiency. We experimentally verify that our approach outperforms several baseline approaches over four real-world datasets.
更多查看译文
关键词
learning skyblocking schemes
AI 理解论文
溯源树
样例
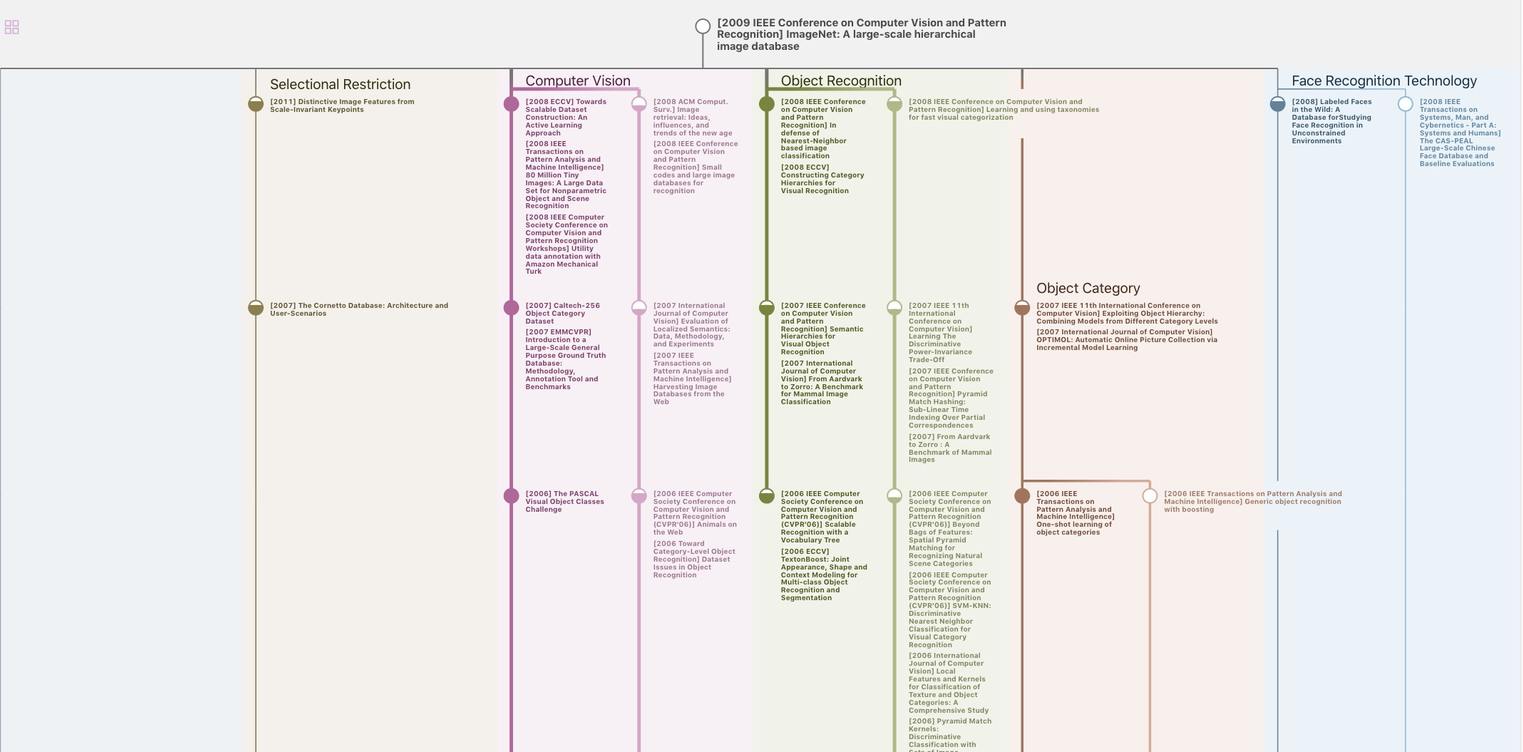
生成溯源树,研究论文发展脉络
Chat Paper
正在生成论文摘要