A Reinforcement Learning Approach of Data Forwarding in Vehicular Networks.
MSN(2017)
摘要
As the basis of vehicle ad hoc networks, the method of forwarding data is one of the most important parts which ensures the stability and efficiency of network communication. However, the high-speed mobile vehicle nodes cause frequent changes of network topology and disconnections of network links, casting a big challenge to the performance of network data delivery. Data forwarding methods based on the prior knowledge of vehicle’s trajectory are difficult to adapt to the changing vehicle trajectory in real world applications, while getting destination vehicles’ positions in broadcast way are extremely costly. To solve the above problems, we have proposed an association state based optimized data forwarding method (ASODF) with the assistance of low loaded road side units (RSU). The proposed method maps the urban road network into a directed graph, utilizes the carry-forward mechanism and decomposes the data transmission into decision-making data forwarding at intersections and data delivery on roads. The vehicles carried data combine the destination nodes locations obtained by low loaded road side units and their locations into association states, and the association state optimization problem is formalized as a Reinforcement Learning problem with Markov Decision Process (MDP). We utilized the value iteration scheme to figure out the delay-optimal policy, which is further used to forward data packets to obtain the best delay of data transmission. Experiments based on a real vehicle trajectory data set demonstrate the effectiveness of our model ASODF.
更多查看译文
AI 理解论文
溯源树
样例
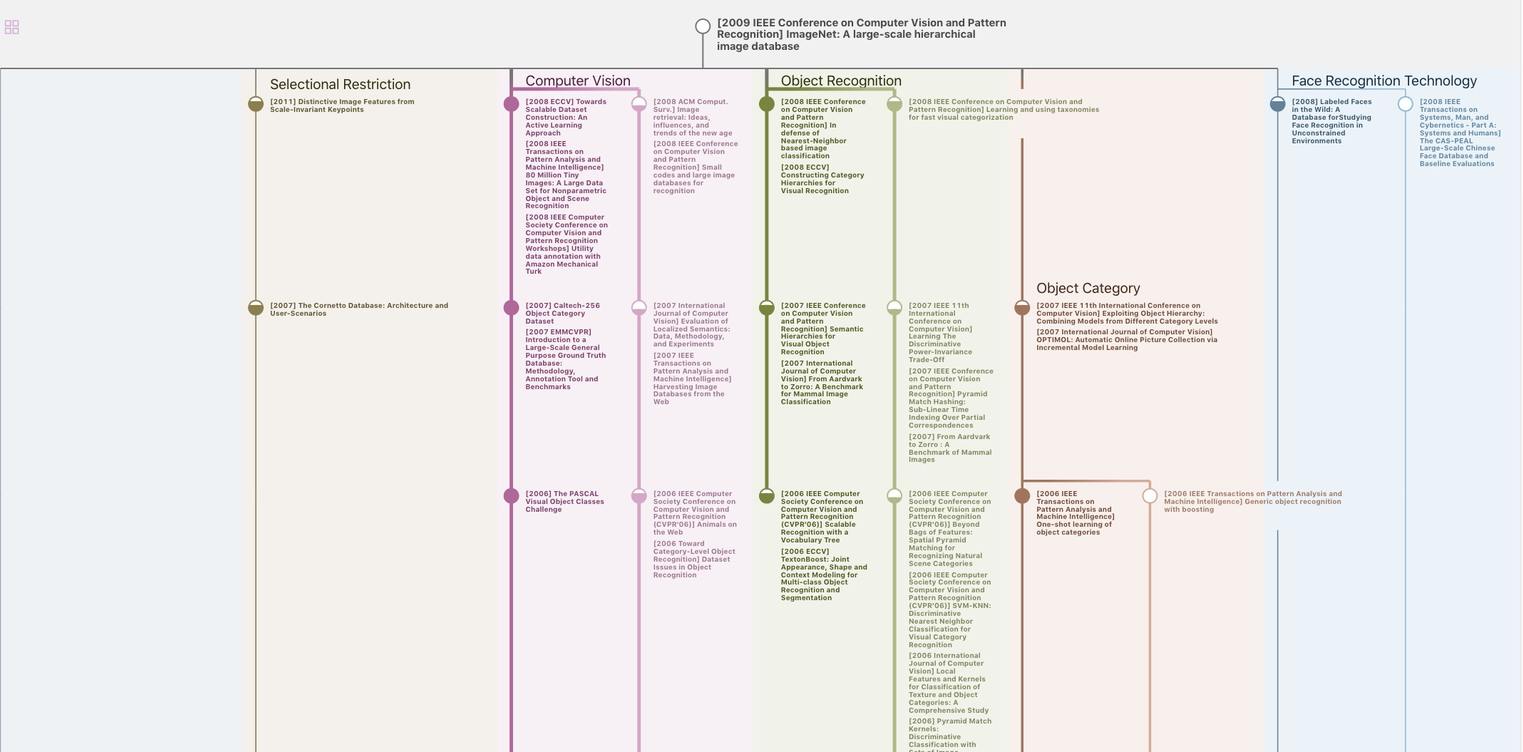
生成溯源树,研究论文发展脉络
Chat Paper
正在生成论文摘要