Asymmetric Discrete Cross-Modal Hashing.
ICMR '18: International Conference on Multimedia Retrieval Yokohama Japan June, 2018(2018)
摘要
Recently, cross-modal hashing (CMH) methods have attracted much attention. Many methods have been explored; however, there are still some issues that need to be further considered. 1) How to efficiently construct the correlations among heterogeneous modalities. 2) How to solve the NP-hard optimization problem and avoid the large quantization errors generated by relaxation. 3) How to handle the complex and difficult problem in most CMH methods that simultaneously learning the hash codes and hash functions. To address these challenges, we present a novel cross-modal hashing algorithm, named Asymmetric Discrete Cross-Modal Hashing (ADCH). Specifically, it leverages the collective matrix factorization technique to learn the common latent representations while preserving not only the cross-correlation from different modalities but also the semantic similarity. Instead of relaxing the binary constraints, it generates the hash codes directly using an iterative optimization algorithm proposed in this work. Based the learnt hash codes, ADCH further learns a series of binary classifiers as hash functions, which is flexible and effective. Extensive experiments are conducted on three real-world datasets. The results demonstrate that ADCH outperforms several state-of-the-art cross-modal hashing baselines.
更多查看译文
关键词
Multimedia Retrieval, Cross-Modal Retrieval, Hashing, Learning to Hash, Discrete Optimization
AI 理解论文
溯源树
样例
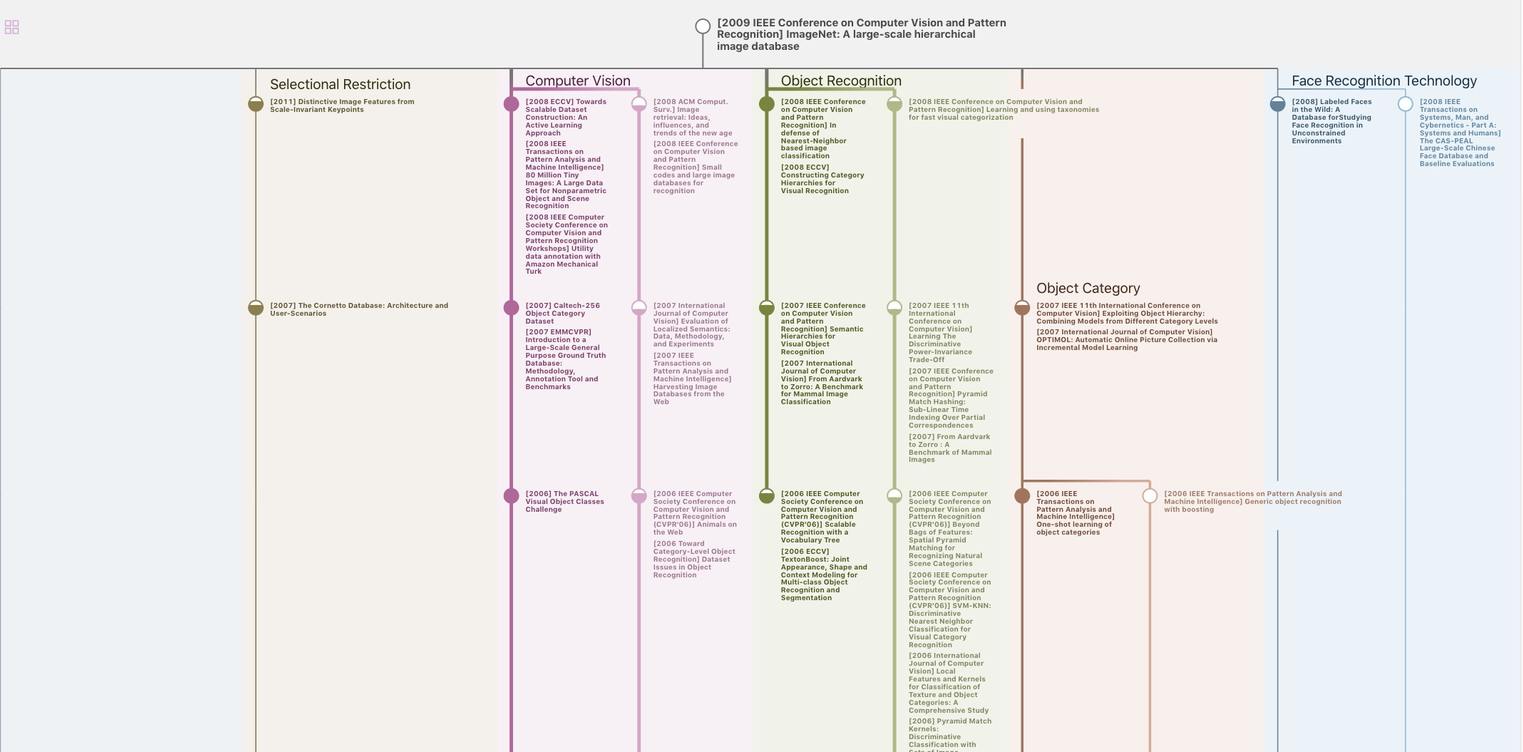
生成溯源树,研究论文发展脉络
Chat Paper
正在生成论文摘要