FPGA Hardware Implementation of Smart Home Autonomous System Based on Deep Learning.
ICIOT(2018)
摘要
The use of deep learning algorithms, as a core element of artificial intelligence, has attracted increased attention from industrial and academic institutes recently. One important use of deep learning is to predict the next user action inside an intelligent home environment that is based on Internet of Things (IoT). Recent researcher discusses the benefit of using deep learning based on different datasets to assist their result. However, assuring the best performance to satisfy real-time applications leads us to use a real-world dataset to make sure that the designed system meets the requirements of real-time applications. This paper uses the MavPad dataset which was gathered from distributed sensors and actuators in a real-world environment. The authors use simulation to investigate the performance of a multilayer neural network that predicts future human actions. The authors also present a hardware implementation of the deep learning model on an FPGA. The results showed that the hardware implementation demonstrated similar accuracy with significantly improved performance compared to the software-based implementation due to the exploitation of parallel computing and using optimization techniques to map the designed system into the target device. Additionally, our implementation of FPGA-based neural network system supports its future utilization for other applications.
更多查看译文
关键词
IoT, Smart environment, Middleware, Machine Learning Algorithm, Neural network, Hardware implementation, FPGA, Embedded systems
AI 理解论文
溯源树
样例
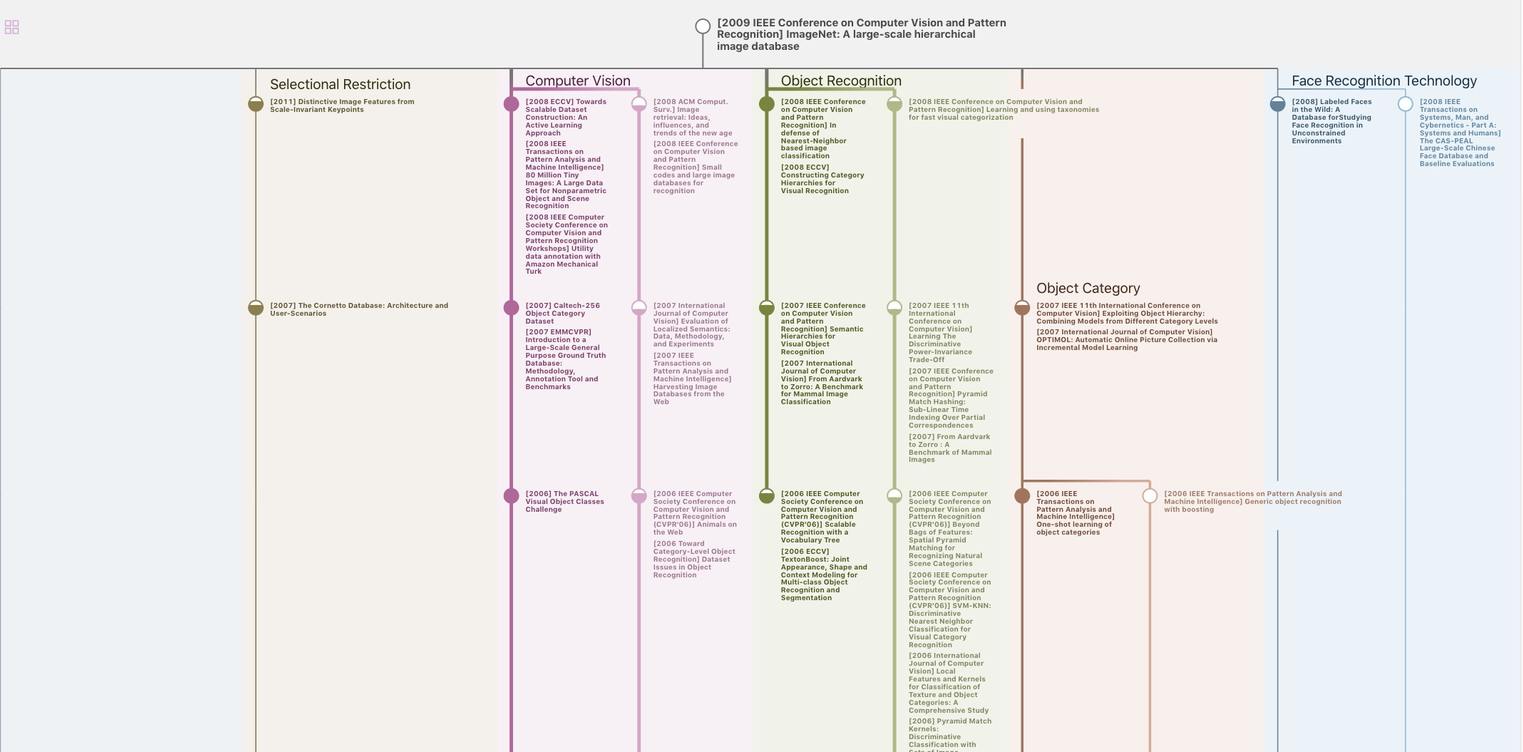
生成溯源树,研究论文发展脉络
Chat Paper
正在生成论文摘要