Social Anchor-Unit Graph Regularized Tensor Completion for Large-Scale Image Retagging.
IEEE Transactions on Pattern Analysis and Machine Intelligence(2019)
摘要
Image retagging aims to improve the tag quality of social images by completing the missing tags, recrifying the noise-corrupted tags, and assigning new high-quality tags. Recent approaches simultaneously explore visual, user and tag information to improve the performance of image retagging by mining the tag-image-user associations. However, such methods will become computationally infeasible with the rapidly increasing number of images, tags and users. It has been proven that the anchor graph can significantly accelerate large-scale graph-based learning by exploring only a small number of anchor points. Inspired by this, we propose a novel Social anchor-Unit GrAph Regularized Tensor Completion (SUGAR-TC) method to efficiently refine the tags of social images, which is insensitive to the scale of data. First, we construct an anchor-unit graph across multiple domains (e.g., image and user domains) rather than traditional anchor graph in a single domain. Second, a tensor completion based on Social anchor-Unit GrAph Regularization (SUGAR) is implemented to refine the tags of the anchor images. Finally, we efficiently assign tags to non-anchor images by leveraging the relationship between the non-anchor units and the anchor units. Experimental results on a real-world social image database well demonstrate the effectiveness and efficiency of SUGAR-TC, outperforming the state-of-the-arts methods.
更多查看译文
关键词
Matrix decomposition,Sugar,Visualization,Computational efficiency,Sparse matrices,Image retrieval
AI 理解论文
溯源树
样例
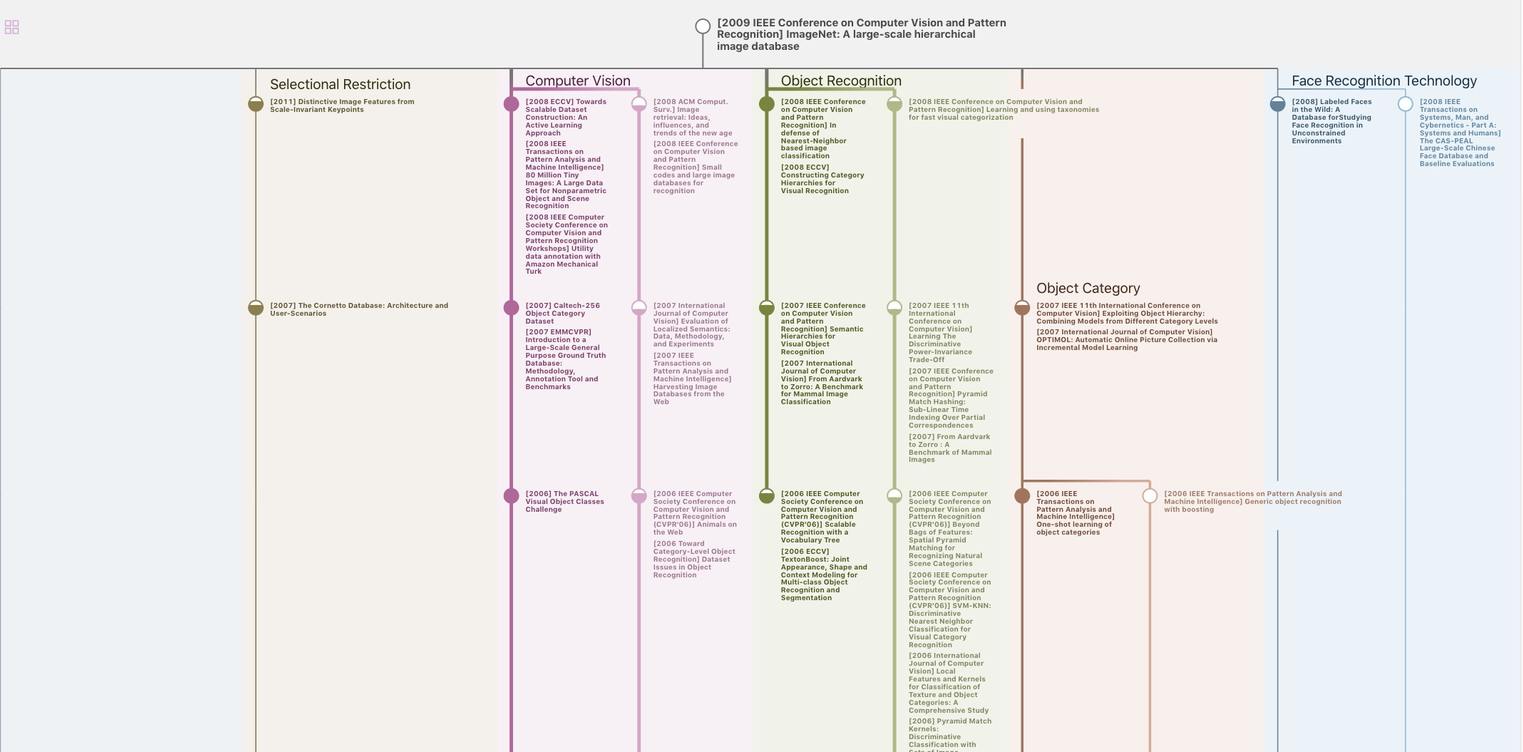
生成溯源树,研究论文发展脉络
Chat Paper
正在生成论文摘要