Multi-modal Learning from Unpaired Images: Application to Multi-organ Segmentation in CT and MRI
2018 IEEE Winter Conference on Applications of Computer Vision (WACV)(2018)
摘要
Convolutional neural networks have been widely used in medical image segmentation. The amount of training data strongly determines the overall performance. Most approaches are applied for a single imaging modality, e.g., brain MRI. In practice, it is often difficult to acquire sufficient training data of a certain imaging modality. The same anatomical structures, however, may be visible in different modalities such as major organs on abdominal CT and MRI. In this work, we investigate the effectiveness of learning from multiple modalities to improve the segmentation accuracy on each individual modality. We study the feasibility of using a dual-stream encoder-decoder architecture to learn modality-independent, and thus, generalisable and robust features. All of our MRI and CT data are unpaired, which means they are obtained from different subjects and not registered to each other. Experiments show that multi-modal learning can improve overall accuracy over modality-specific training. Results demonstrate that information across modalities can in particular improve performance on varying structures such as the spleen.
更多查看译文
关键词
convolutional neural networks,medical image segmentation,brain MRI,imaging modality,multimodal learning,modality-specific training,unpaired images,dualstream encoder-decoder,anatomical structure,MRI,CT
AI 理解论文
溯源树
样例
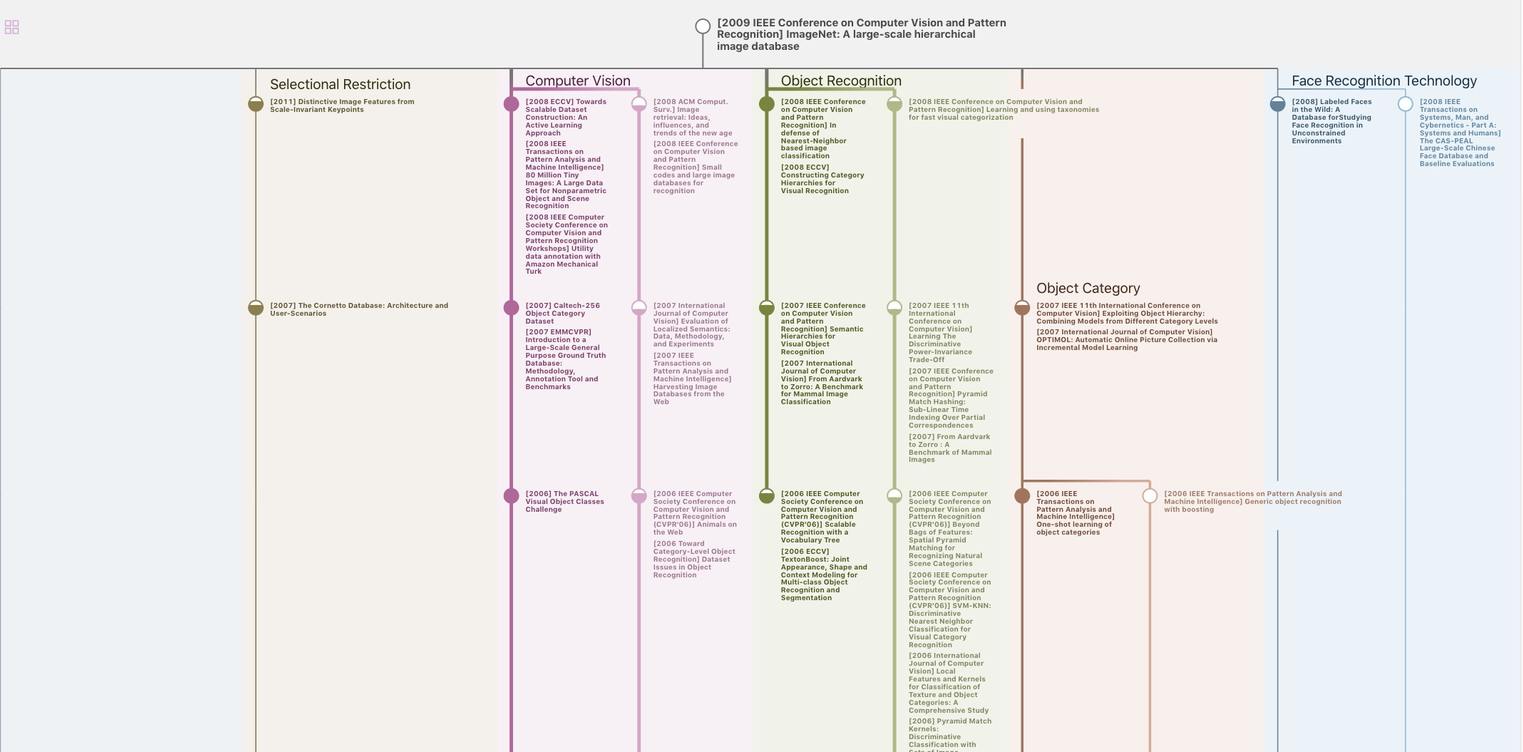
生成溯源树,研究论文发展脉络
Chat Paper
正在生成论文摘要