Simulation Study To Identify The Characteristics Of Markov Chain Properties
SIGSIM-PADS '18: SIGSIM Principles of Advanced Discrete Simulation Rome Italy May, 2018(2020)
摘要
Markov models have a long tradition in modeling and simulation of dynamic systems. In this article, we look at certain properties of a discrete-time Markov chain, including entropy, trace, and second-largest eigenvalue to better understand their role for time-series analysis. We simulate a number of possible input signals, fit a discrete-time Markov chain, and explore properties with the help of Sobol indices, partial correlation coefficients, and the Morris elementary effect screening method. Our analysis suggests that the presence of a trend, periodicity, and autocorrelation impact entropy, trace, and second-largest eigenvalue to varying degrees but not independently of each other and with Markov chain parameter settings as other influencing factors. The properties of interest are promising to distinguish time-series data, as evidenced for the entropy measure by recent results in the analysis of cell development for Xenopus laevis in cell biology.
更多查看译文
关键词
Monte Carlo simulation,discrete-time Markov chain (DTMC),sensitivity analysis,partial correlation coefficients (PCC),Sobol indices,Morris method,entropy,trace,eigenvalue
AI 理解论文
溯源树
样例
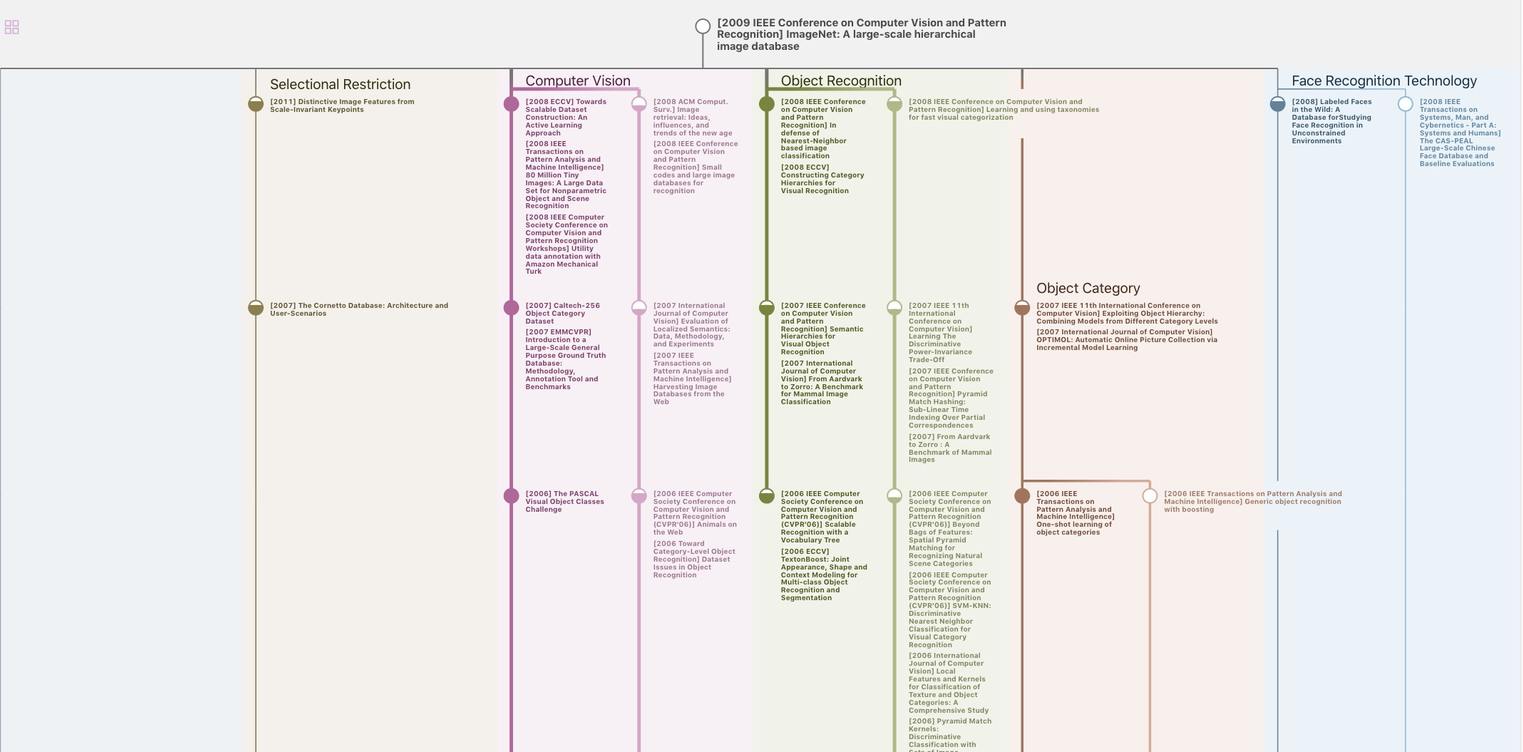
生成溯源树,研究论文发展脉络
Chat Paper
正在生成论文摘要